کد مقاله | کد نشریه | سال انتشار | مقاله انگلیسی | نسخه تمام متن |
---|---|---|---|---|
408022 | 678242 | 2011 | 7 صفحه PDF | دانلود رایگان |
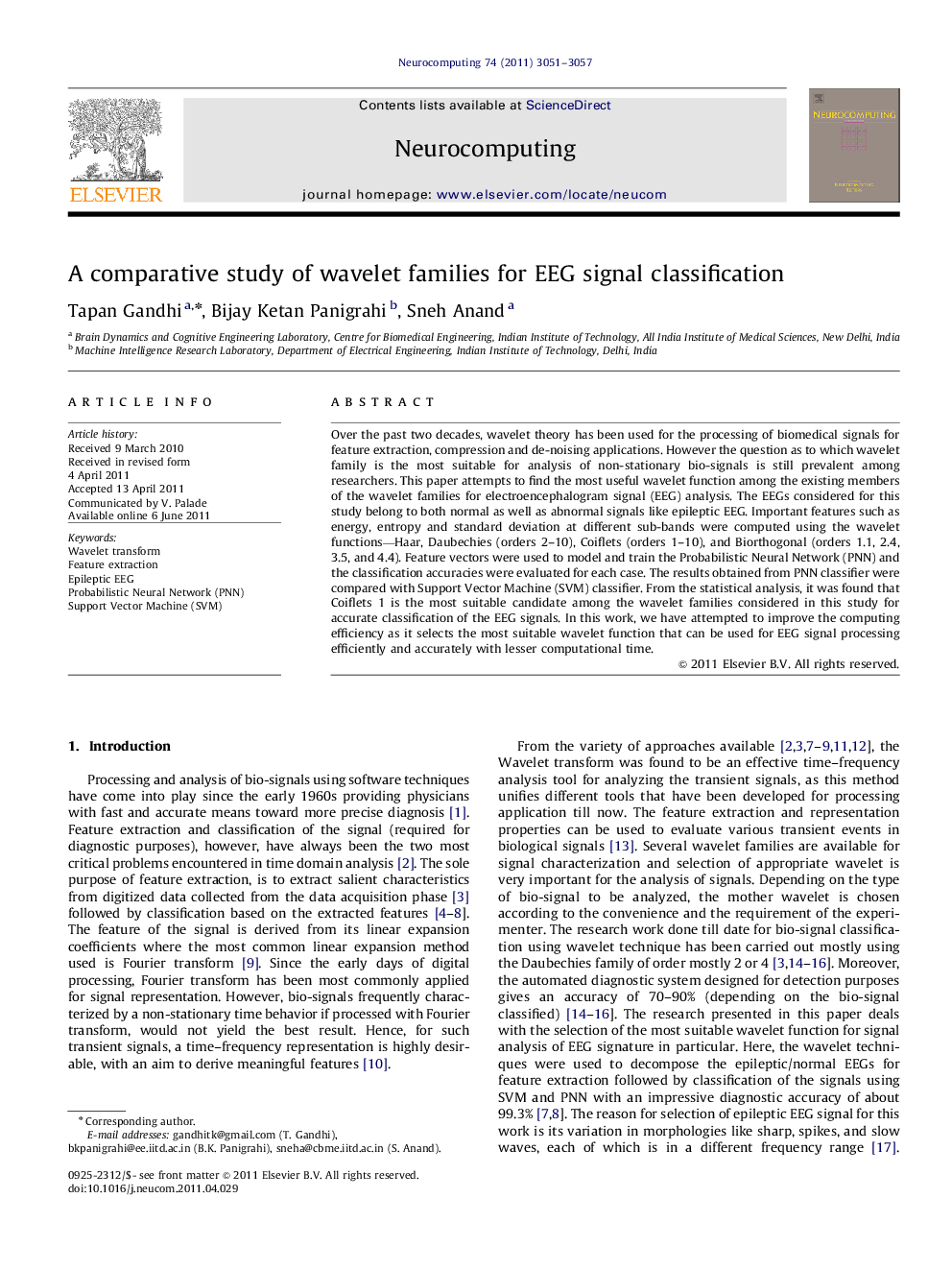
Over the past two decades, wavelet theory has been used for the processing of biomedical signals for feature extraction, compression and de-noising applications. However the question as to which wavelet family is the most suitable for analysis of non-stationary bio-signals is still prevalent among researchers. This paper attempts to find the most useful wavelet function among the existing members of the wavelet families for electroencephalogram signal (EEG) analysis. The EEGs considered for this study belong to both normal as well as abnormal signals like epileptic EEG. Important features such as energy, entropy and standard deviation at different sub-bands were computed using the wavelet functions—Haar, Daubechies (orders 2–10), Coiflets (orders 1–10), and Biorthogonal (orders 1.1, 2.4, 3.5, and 4.4). Feature vectors were used to model and train the Probabilistic Neural Network (PNN) and the classification accuracies were evaluated for each case. The results obtained from PNN classifier were compared with Support Vector Machine (SVM) classifier. From the statistical analysis, it was found that Coiflets 1 is the most suitable candidate among the wavelet families considered in this study for accurate classification of the EEG signals. In this work, we have attempted to improve the computing efficiency as it selects the most suitable wavelet function that can be used for EEG signal processing efficiently and accurately with lesser computational time.
► The most useful wavelet function was found out for EEG signal analysis.
► Coiflets 1 is the suitable candidate for accurate classification of the EEG signal.
► Higher efficiency and accuracy were obtained in less computational time.
Journal: Neurocomputing - Volume 74, Issue 17, October 2011, Pages 3051–3057