کد مقاله | کد نشریه | سال انتشار | مقاله انگلیسی | نسخه تمام متن |
---|---|---|---|---|
408267 | 679015 | 2011 | 10 صفحه PDF | دانلود رایگان |
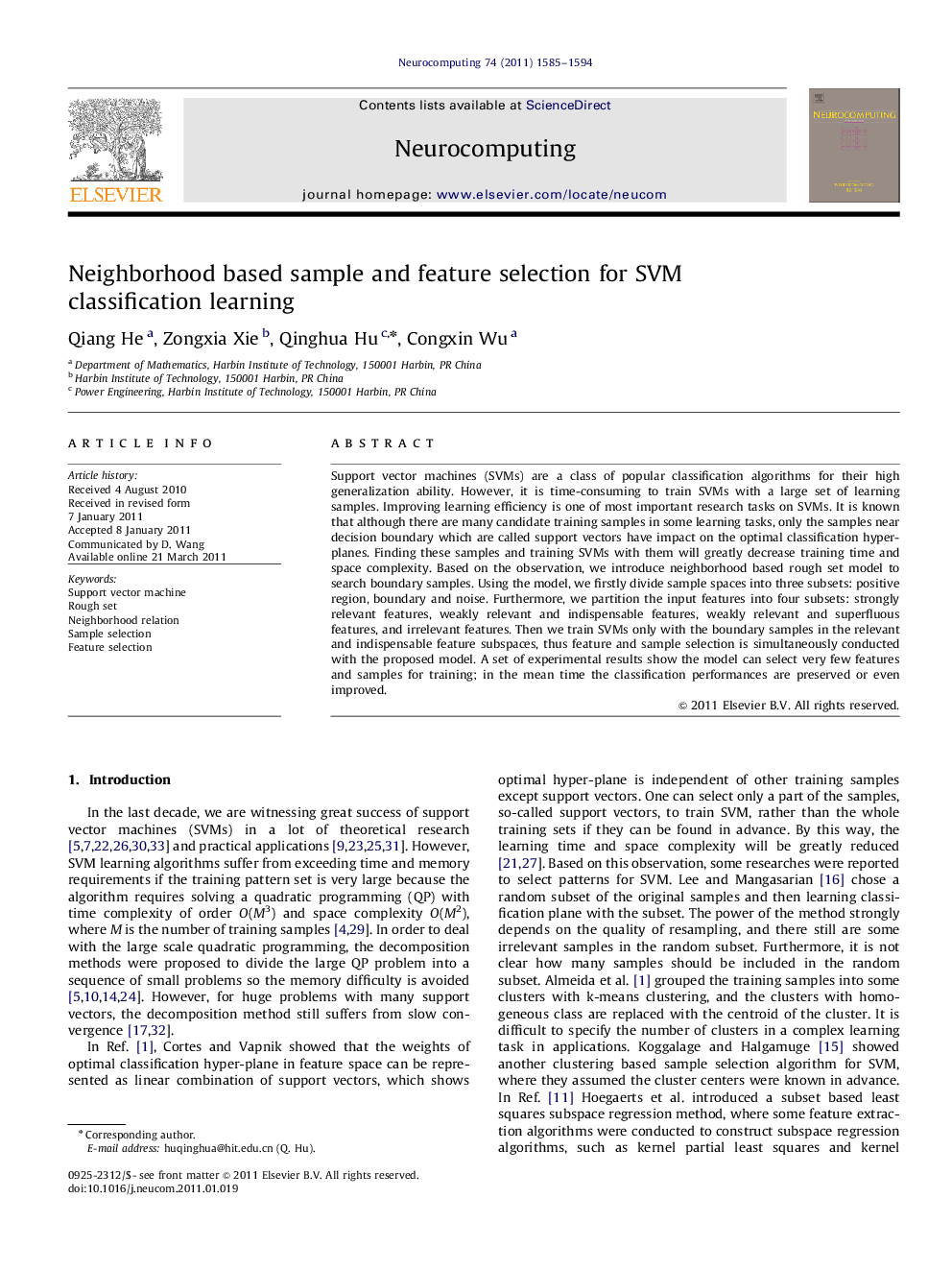
Support vector machines (SVMs) are a class of popular classification algorithms for their high generalization ability. However, it is time-consuming to train SVMs with a large set of learning samples. Improving learning efficiency is one of most important research tasks on SVMs. It is known that although there are many candidate training samples in some learning tasks, only the samples near decision boundary which are called support vectors have impact on the optimal classification hyper-planes. Finding these samples and training SVMs with them will greatly decrease training time and space complexity. Based on the observation, we introduce neighborhood based rough set model to search boundary samples. Using the model, we firstly divide sample spaces into three subsets: positive region, boundary and noise. Furthermore, we partition the input features into four subsets: strongly relevant features, weakly relevant and indispensable features, weakly relevant and superfluous features, and irrelevant features. Then we train SVMs only with the boundary samples in the relevant and indispensable feature subspaces, thus feature and sample selection is simultaneously conducted with the proposed model. A set of experimental results show the model can select very few features and samples for training; in the mean time the classification performances are preserved or even improved.
Journal: Neurocomputing - Volume 74, Issue 10, May 2011, Pages 1585–1594