کد مقاله | کد نشریه | سال انتشار | مقاله انگلیسی | نسخه تمام متن |
---|---|---|---|---|
409922 | 679106 | 2014 | 8 صفحه PDF | دانلود رایگان |
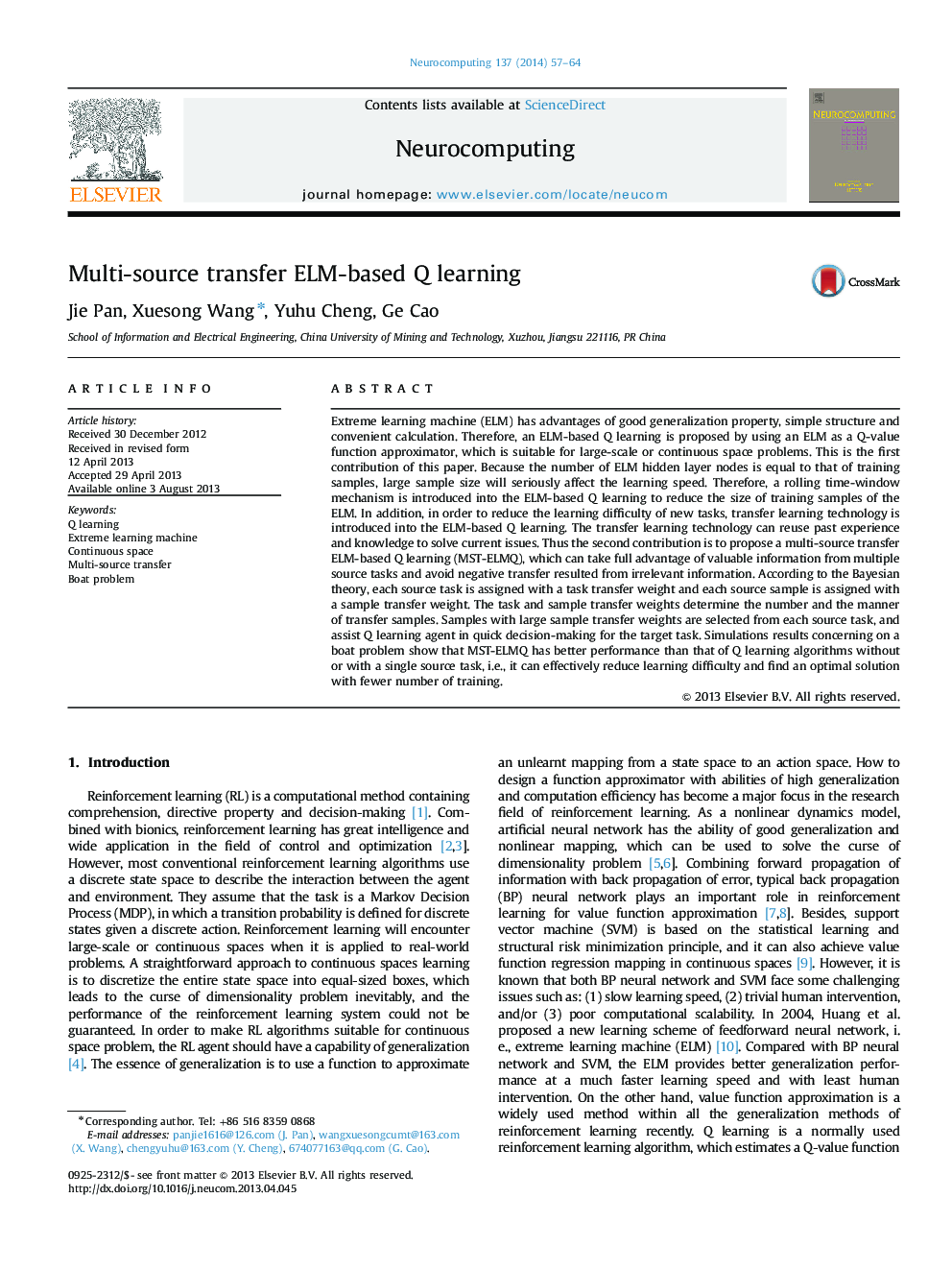
Extreme learning machine (ELM) has advantages of good generalization property, simple structure and convenient calculation. Therefore, an ELM-based Q learning is proposed by using an ELM as a Q-value function approximator, which is suitable for large-scale or continuous space problems. This is the first contribution of this paper. Because the number of ELM hidden layer nodes is equal to that of training samples, large sample size will seriously affect the learning speed. Therefore, a rolling time-window mechanism is introduced into the ELM-based Q learning to reduce the size of training samples of the ELM. In addition, in order to reduce the learning difficulty of new tasks, transfer learning technology is introduced into the ELM-based Q learning. The transfer learning technology can reuse past experience and knowledge to solve current issues. Thus the second contribution is to propose a multi-source transfer ELM-based Q learning (MST-ELMQ), which can take full advantage of valuable information from multiple source tasks and avoid negative transfer resulted from irrelevant information. According to the Bayesian theory, each source task is assigned with a task transfer weight and each source sample is assigned with a sample transfer weight. The task and sample transfer weights determine the number and the manner of transfer samples. Samples with large sample transfer weights are selected from each source task, and assist Q learning agent in quick decision-making for the target task. Simulations results concerning on a boat problem show that MST-ELMQ has better performance than that of Q learning algorithms without or with a single source task, i.e., it can effectively reduce learning difficulty and find an optimal solution with fewer number of training.
Journal: Neurocomputing - Volume 137, 5 August 2014, Pages 57–64