کد مقاله | کد نشریه | سال انتشار | مقاله انگلیسی | نسخه تمام متن |
---|---|---|---|---|
410150 | 679124 | 2013 | 14 صفحه PDF | دانلود رایگان |
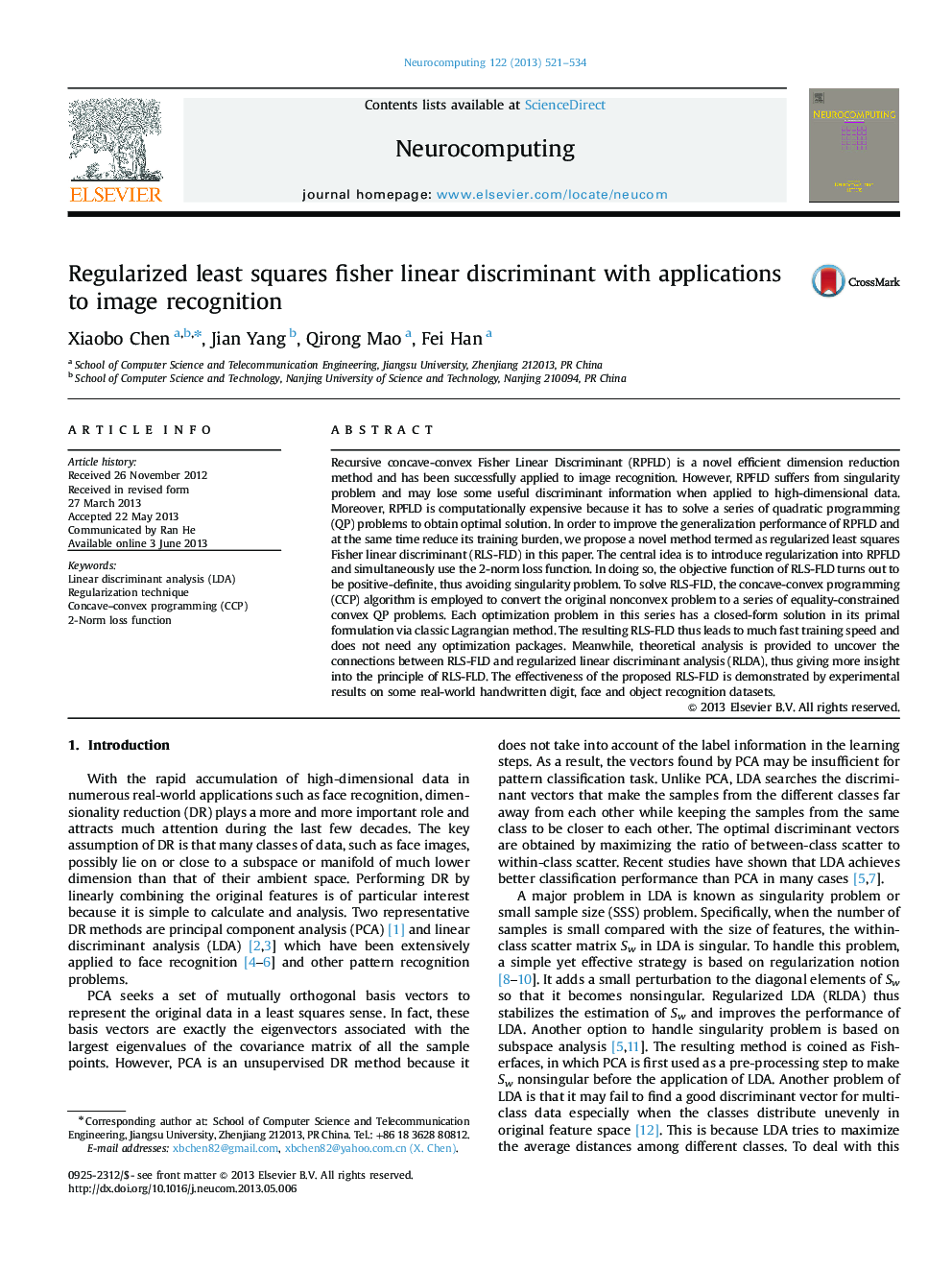
Recursive concave-convex Fisher Linear Discriminant (RPFLD) is a novel efficient dimension reduction method and has been successfully applied to image recognition. However, RPFLD suffers from singularity problem and may lose some useful discriminant information when applied to high-dimensional data. Moreover, RPFLD is computationally expensive because it has to solve a series of quadratic programming (QP) problems to obtain optimal solution. In order to improve the generalization performance of RPFLD and at the same time reduce its training burden, we propose a novel method termed as regularized least squares Fisher linear discriminant (RLS-FLD) in this paper. The central idea is to introduce regularization into RPFLD and simultaneously use the 2-norm loss function. In doing so, the objective function of RLS-FLD turns out to be positive-definite, thus avoiding singularity problem. To solve RLS-FLD, the concave-convex programming (CCP) algorithm is employed to convert the original nonconvex problem to a series of equality-constrained convex QP problems. Each optimization problem in this series has a closed-form solution in its primal formulation via classic Lagrangian method. The resulting RLS-FLD thus leads to much fast training speed and does not need any optimization packages. Meanwhile, theoretical analysis is provided to uncover the connections between RLS-FLD and regularized linear discriminant analysis (RLDA), thus giving more insight into the principle of RLS-FLD. The effectiveness of the proposed RLS-FLD is demonstrated by experimental results on some real-world handwritten digit, face and object recognition datasets.
Journal: Neurocomputing - Volume 122, 25 December 2013, Pages 521–534