کد مقاله | کد نشریه | سال انتشار | مقاله انگلیسی | نسخه تمام متن |
---|---|---|---|---|
410385 | 679140 | 2010 | 9 صفحه PDF | دانلود رایگان |
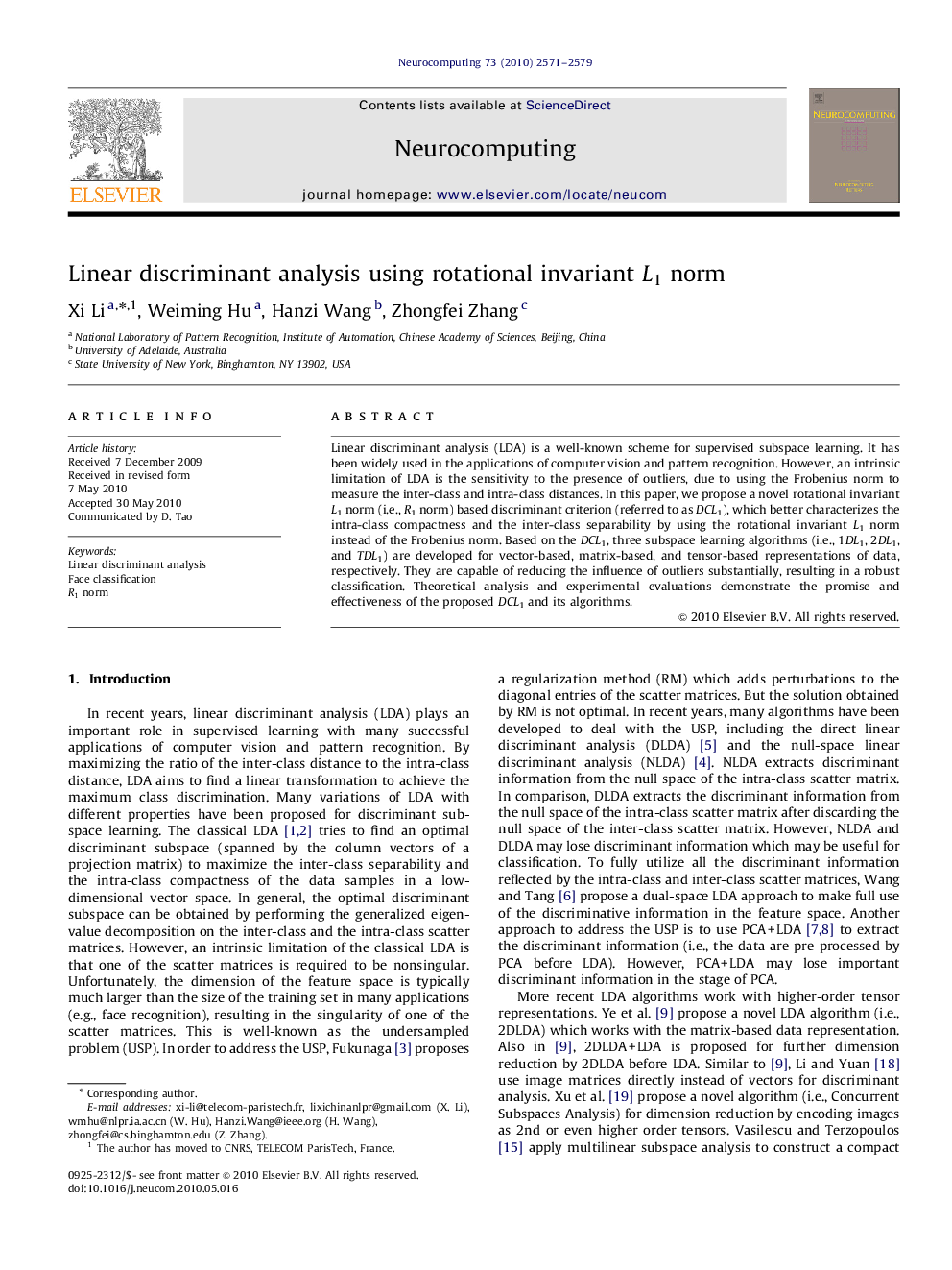
Linear discriminant analysis (LDA) is a well-known scheme for supervised subspace learning. It has been widely used in the applications of computer vision and pattern recognition. However, an intrinsic limitation of LDA is the sensitivity to the presence of outliers, due to using the Frobenius norm to measure the inter-class and intra-class distances. In this paper, we propose a novel rotational invariant L1 norm (i.e., R1 norm) based discriminant criterion (referred to as DCL1), which better characterizes the intra-class compactness and the inter-class separability by using the rotational invariant L1 norm instead of the Frobenius norm. Based on the DCL1, three subspace learning algorithms (i.e., 1DL1, 2DL1, and TDL1) are developed for vector-based, matrix-based, and tensor-based representations of data, respectively. They are capable of reducing the influence of outliers substantially, resulting in a robust classification. Theoretical analysis and experimental evaluations demonstrate the promise and effectiveness of the proposed DCL1 and its algorithms.
Journal: Neurocomputing - Volume 73, Issues 13–15, August 2010, Pages 2571–2579