کد مقاله | کد نشریه | سال انتشار | مقاله انگلیسی | نسخه تمام متن |
---|---|---|---|---|
410473 | 679146 | 2009 | 12 صفحه PDF | دانلود رایگان |
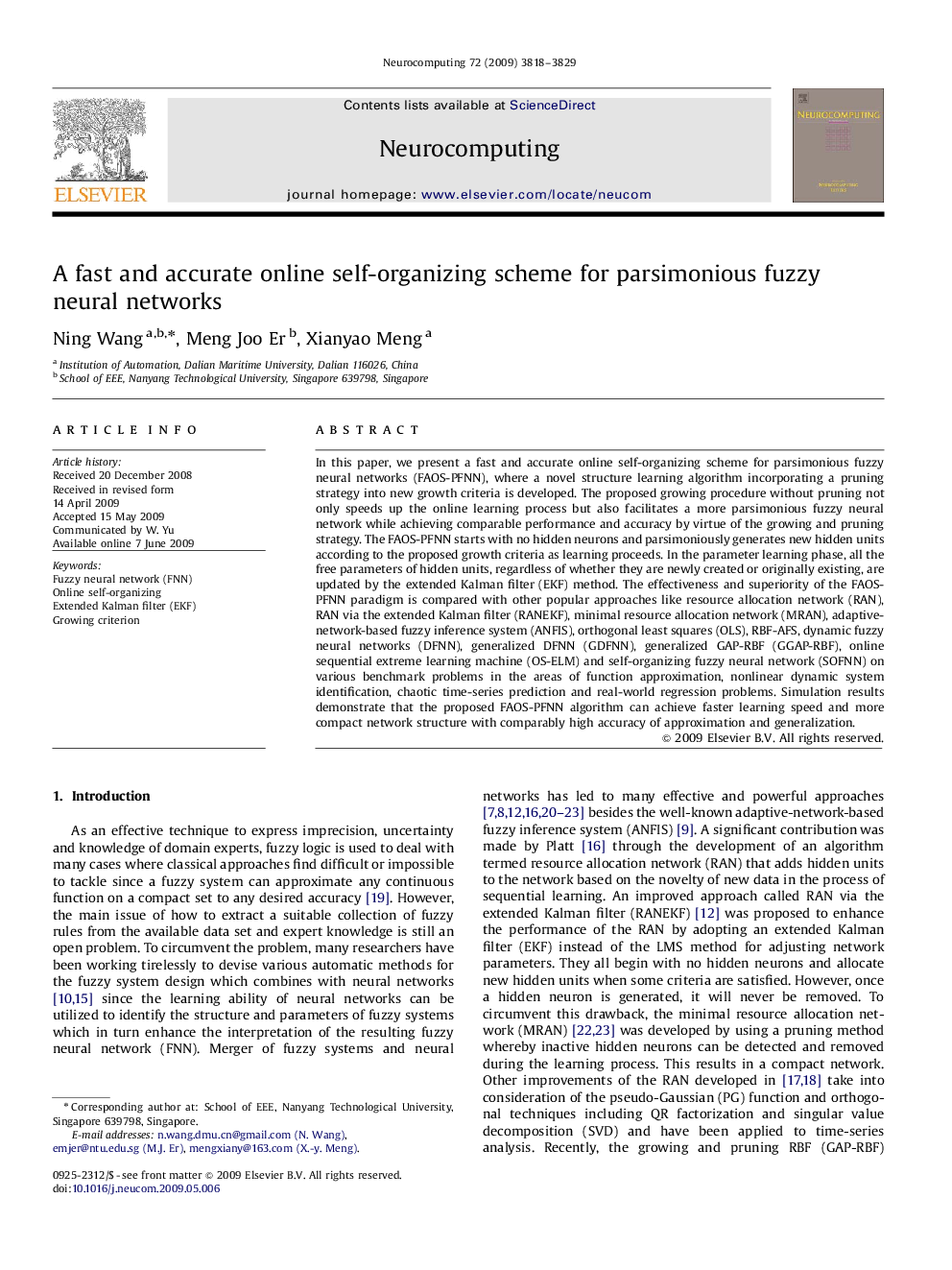
In this paper, we present a fast and accurate online self-organizing scheme for parsimonious fuzzy neural networks (FAOS-PFNN), where a novel structure learning algorithm incorporating a pruning strategy into new growth criteria is developed. The proposed growing procedure without pruning not only speeds up the online learning process but also facilitates a more parsimonious fuzzy neural network while achieving comparable performance and accuracy by virtue of the growing and pruning strategy. The FAOS-PFNN starts with no hidden neurons and parsimoniously generates new hidden units according to the proposed growth criteria as learning proceeds. In the parameter learning phase, all the free parameters of hidden units, regardless of whether they are newly created or originally existing, are updated by the extended Kalman filter (EKF) method. The effectiveness and superiority of the FAOS-PFNN paradigm is compared with other popular approaches like resource allocation network (RAN), RAN via the extended Kalman filter (RANEKF), minimal resource allocation network (MRAN), adaptive-network-based fuzzy inference system (ANFIS), orthogonal least squares (OLS), RBF-AFS, dynamic fuzzy neural networks (DFNN), generalized DFNN (GDFNN), generalized GAP-RBF (GGAP-RBF), online sequential extreme learning machine (OS-ELM) and self-organizing fuzzy neural network (SOFNN) on various benchmark problems in the areas of function approximation, nonlinear dynamic system identification, chaotic time-series prediction and real-world regression problems. Simulation results demonstrate that the proposed FAOS-PFNN algorithm can achieve faster learning speed and more compact network structure with comparably high accuracy of approximation and generalization.
Journal: Neurocomputing - Volume 72, Issues 16–18, October 2009, Pages 3818–3829