کد مقاله | کد نشریه | سال انتشار | مقاله انگلیسی | نسخه تمام متن |
---|---|---|---|---|
410887 | 679170 | 2006 | 14 صفحه PDF | دانلود رایگان |
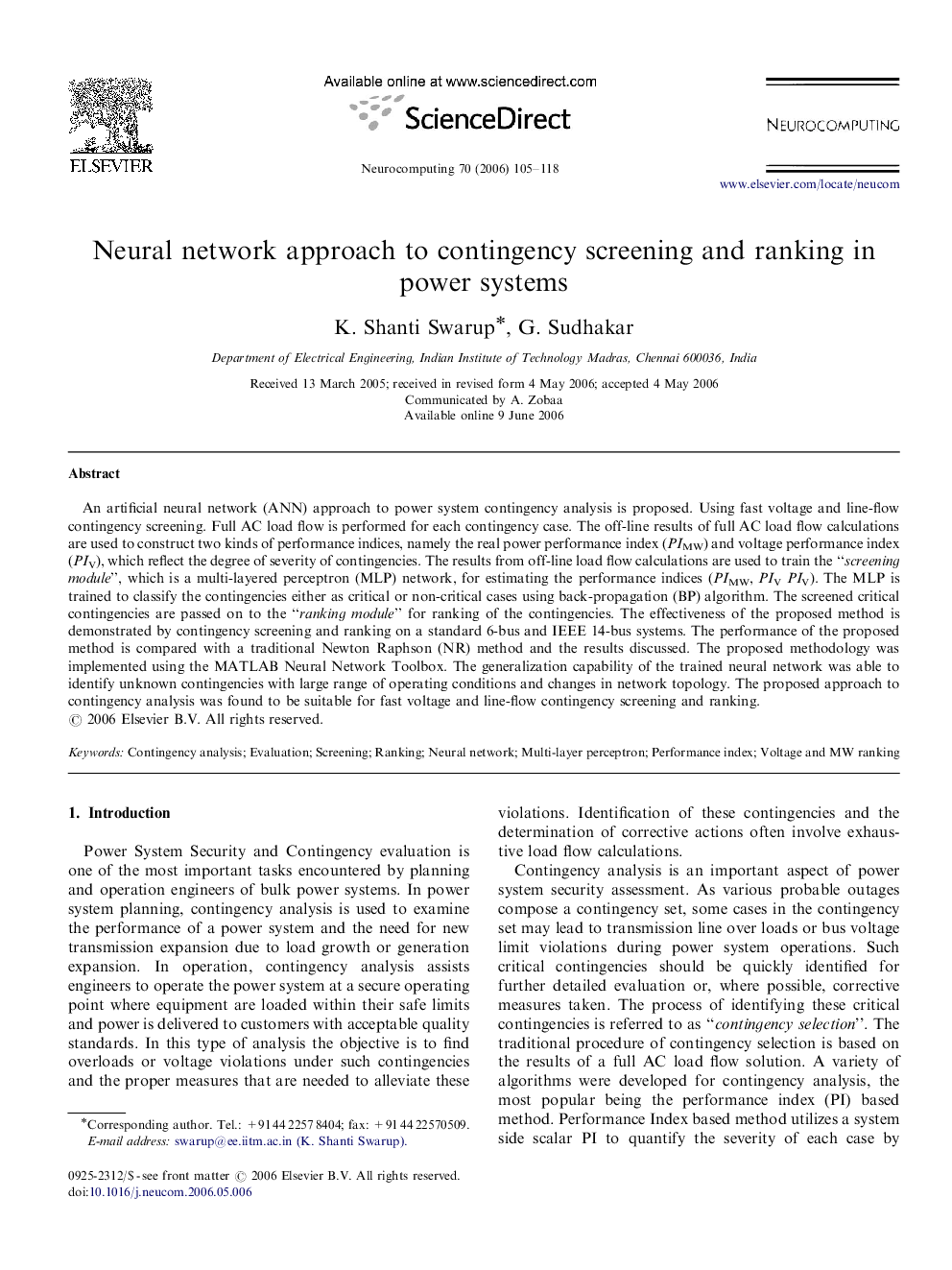
An artificial neural network (ANN) approach to power system contingency analysis is proposed. Using fast voltage and line-flow contingency screening. Full AC load flow is performed for each contingency case. The off-line results of full AC load flow calculations are used to construct two kinds of performance indices, namely the real power performance index (PIMW) and voltage performance index (PIV), which reflect the degree of severity of contingencies. The results from off-line load flow calculations are used to train the “screening module”, which is a multi-layered perceptron (MLP) network, for estimating the performance indices (PIMW, PIVPIV). The MLP is trained to classify the contingencies either as critical or non-critical cases using back-propagation (BP) algorithm. The screened critical contingencies are passed on to the “ranking module” for ranking of the contingencies. The effectiveness of the proposed method is demonstrated by contingency screening and ranking on a standard 6-bus and IEEE 14-bus systems. The performance of the proposed method is compared with a traditional Newton Raphson (NR) method and the results discussed. The proposed methodology was implemented using the MATLAB Neural Network Toolbox. The generalization capability of the trained neural network was able to identify unknown contingencies with large range of operating conditions and changes in network topology. The proposed approach to contingency analysis was found to be suitable for fast voltage and line-flow contingency screening and ranking.
Journal: Neurocomputing - Volume 70, Issues 1–3, December 2006, Pages 105–118