کد مقاله | کد نشریه | سال انتشار | مقاله انگلیسی | نسخه تمام متن |
---|---|---|---|---|
412041 | 679608 | 2015 | 9 صفحه PDF | دانلود رایگان |
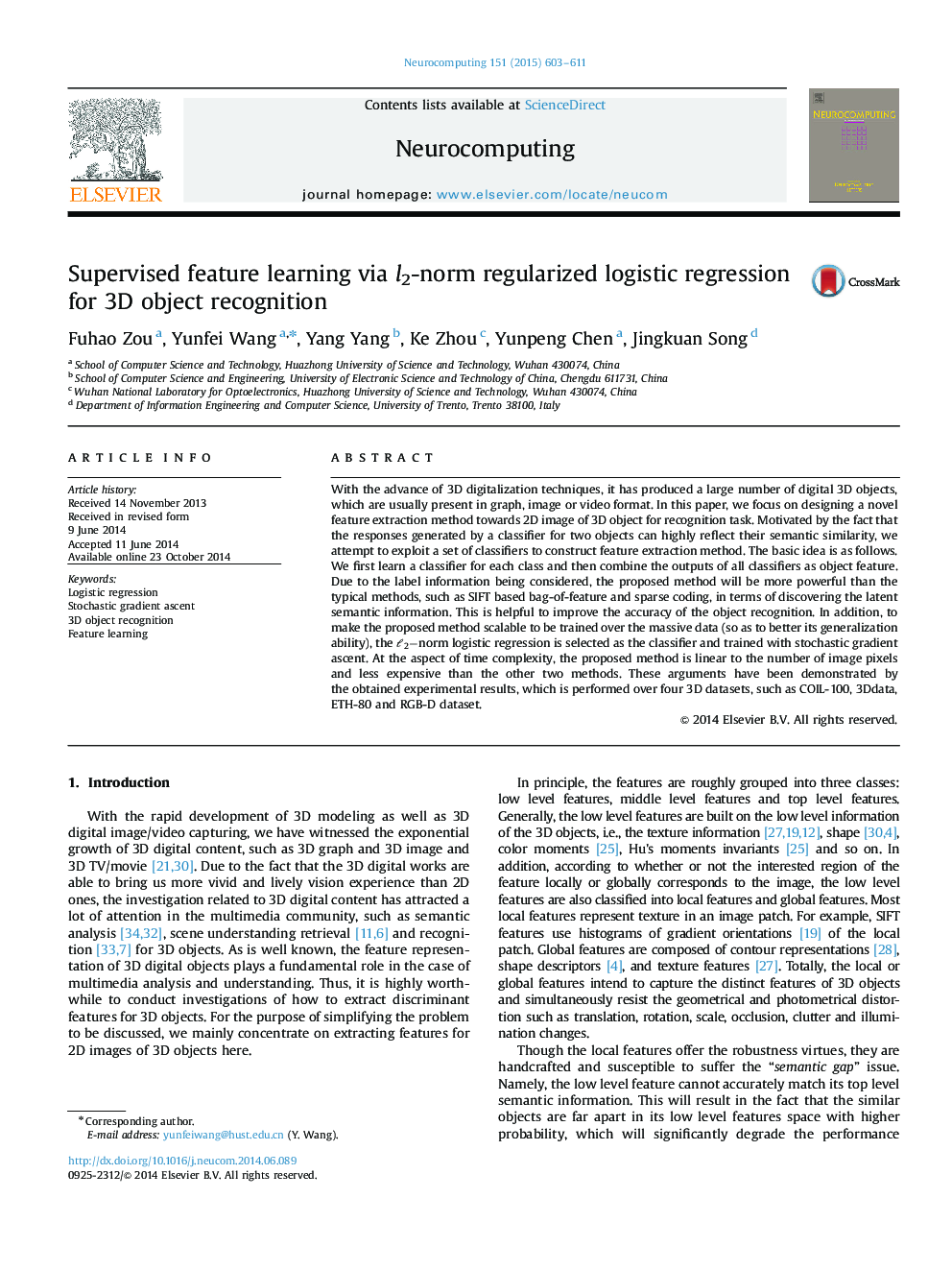
• We exploit a set of logistic regression classifier to construct feature extraction method.
• The proposed method can be scalable to be trained over massive dataset.
• The complexity of the proposed method is linear to the number of pixels of 3D object.
• The proposed method is competitive with the state-of-the-art methods in accuracy.
With the advance of 3D digitalization techniques, it has produced a large number of digital 3D objects, which are usually present in graph, image or video format. In this paper, we focus on designing a novel feature extraction method towards 2D image of 3D object for recognition task. Motivated by the fact that the responses generated by a classifier for two objects can highly reflect their semantic similarity, we attempt to exploit a set of classifiers to construct feature extraction method. The basic idea is as follows. We first learn a classifier for each class and then combine the outputs of all classifiers as object feature. Due to the label information being considered, the proposed method will be more powerful than the typical methods, such as SIFT based bag-of-feature and sparse coding, in terms of discovering the latent semantic information. This is helpful to improve the accuracy of the object recognition. In addition, to make the proposed method scalable to be trained over the massive data (so as to better its generalization ability), the ℓ2-normℓ2-norm logistic regression is selected as the classifier and trained with stochastic gradient ascent. At the aspect of time complexity, the proposed method is linear to the number of image pixels and less expensive than the other two methods. These arguments have been demonstrated by the obtained experimental results, which is performed over four 3D datasets, such as COIL-100, 3Ddata, ETH-80 and RGB-D dataset.
Journal: Neurocomputing - Volume 151, Part 2, 5 March 2015, Pages 603–611