کد مقاله | کد نشریه | سال انتشار | مقاله انگلیسی | نسخه تمام متن |
---|---|---|---|---|
412546 | 679652 | 2012 | 9 صفحه PDF | دانلود رایگان |
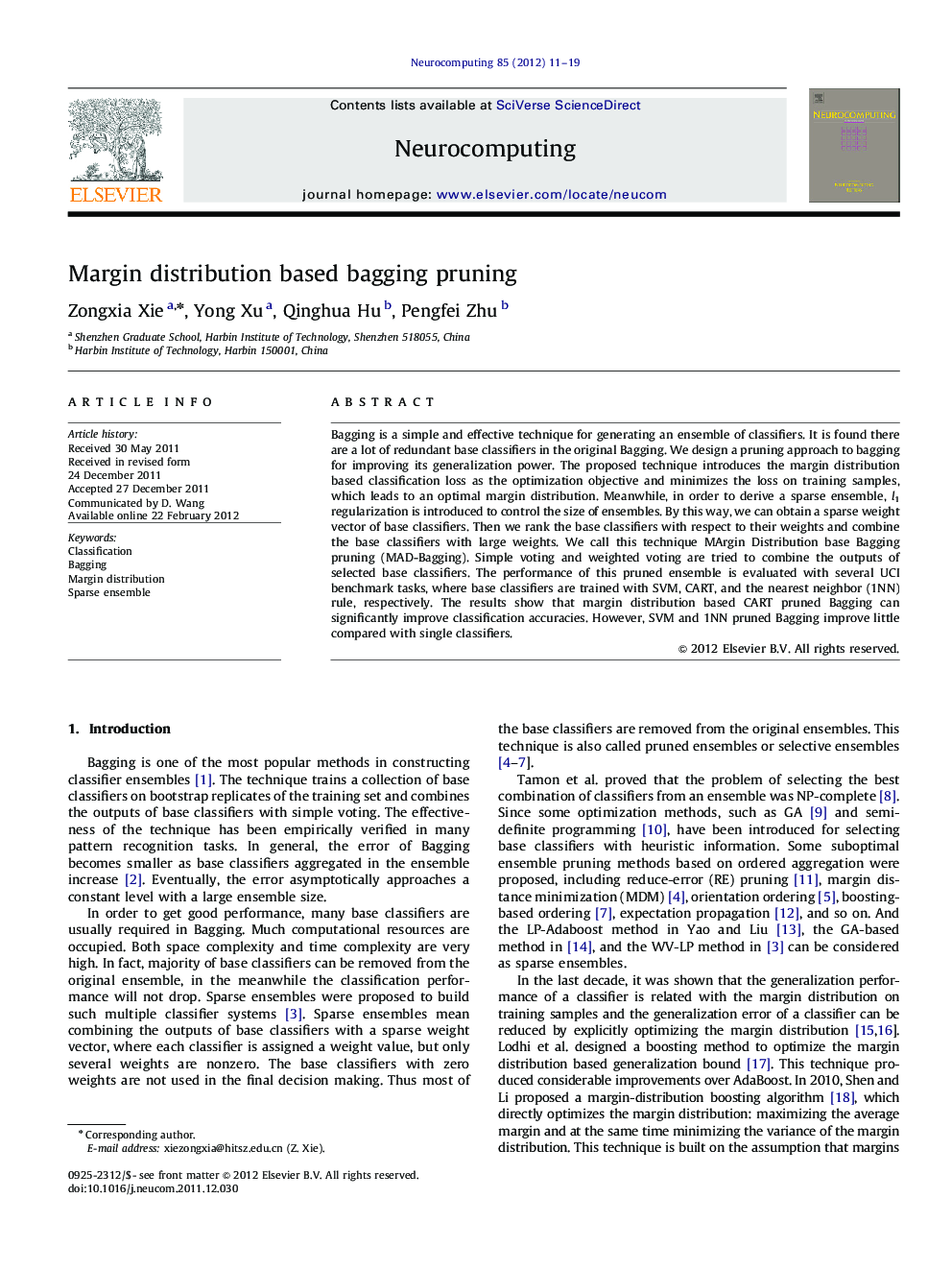
Bagging is a simple and effective technique for generating an ensemble of classifiers. It is found there are a lot of redundant base classifiers in the original Bagging. We design a pruning approach to bagging for improving its generalization power. The proposed technique introduces the margin distribution based classification loss as the optimization objective and minimizes the loss on training samples, which leads to an optimal margin distribution. Meanwhile, in order to derive a sparse ensemble, l1 regularization is introduced to control the size of ensembles. By this way, we can obtain a sparse weight vector of base classifiers. Then we rank the base classifiers with respect to their weights and combine the base classifiers with large weights. We call this technique MArgin Distribution base Bagging pruning (MAD-Bagging). Simple voting and weighted voting are tried to combine the outputs of selected base classifiers. The performance of this pruned ensemble is evaluated with several UCI benchmark tasks, where base classifiers are trained with SVM, CART, and the nearest neighbor (1NN) rule, respectively. The results show that margin distribution based CART pruned Bagging can significantly improve classification accuracies. However, SVM and 1NN pruned Bagging improve little compared with single classifiers.
Journal: Neurocomputing - Volume 85, 15 May 2012, Pages 11–19