کد مقاله | کد نشریه | سال انتشار | مقاله انگلیسی | نسخه تمام متن |
---|---|---|---|---|
4438190 | 1620397 | 2013 | 10 صفحه PDF | دانلود رایگان |
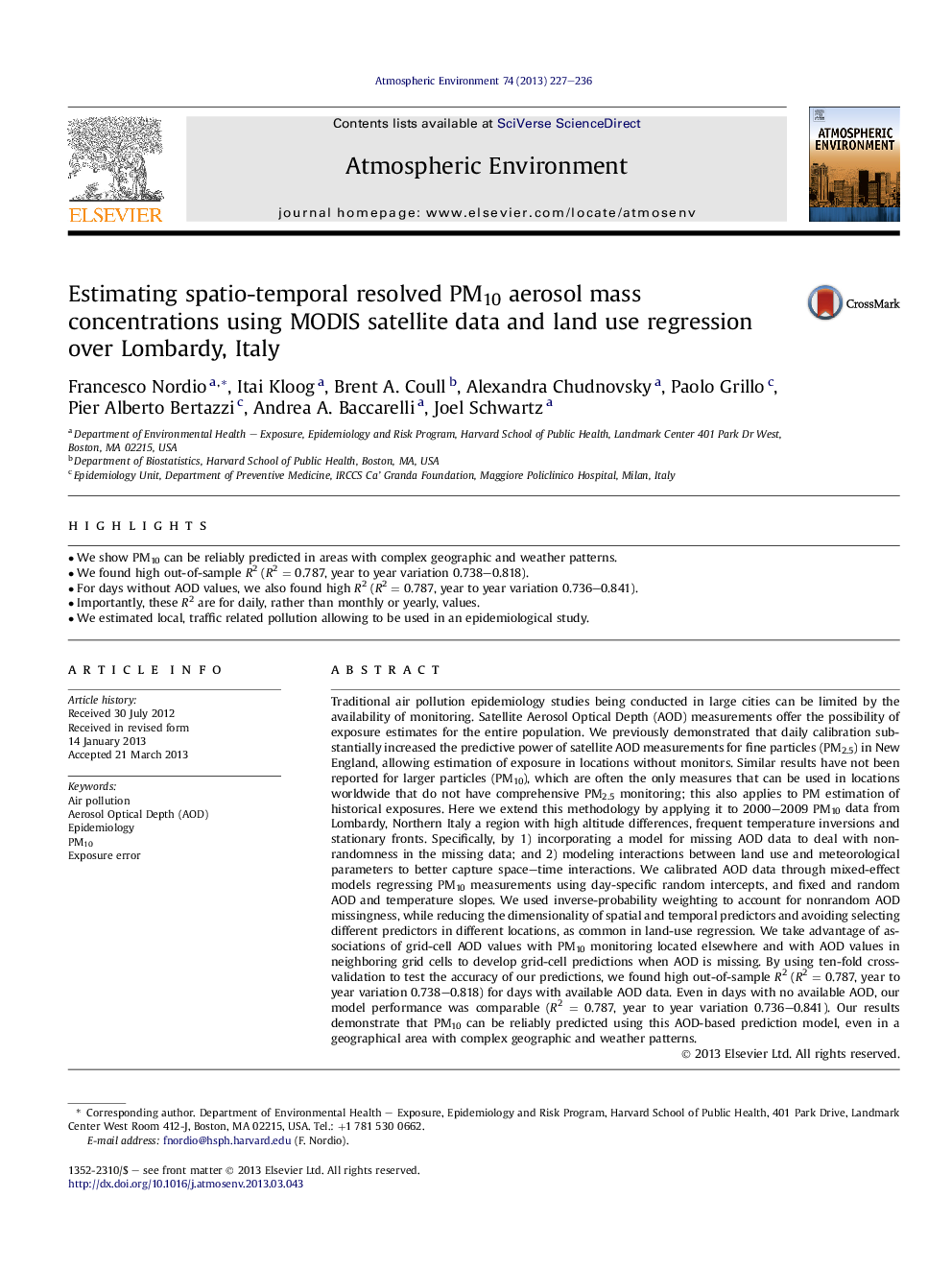
• We show PM10 can be reliably predicted in areas with complex geographic and weather patterns.
• We found high out-of-sample R2 (R2 = 0.787, year to year variation 0.738–0.818).
• For days without AOD values, we also found high R2 (R2 = 0.787, year to year variation 0.736–0.841).
• Importantly, these R2 are for daily, rather than monthly or yearly, values.
• We estimated local, traffic related pollution allowing to be used in an epidemiological study.
Traditional air pollution epidemiology studies being conducted in large cities can be limited by the availability of monitoring. Satellite Aerosol Optical Depth (AOD) measurements offer the possibility of exposure estimates for the entire population. We previously demonstrated that daily calibration substantially increased the predictive power of satellite AOD measurements for fine particles (PM2.5) in New England, allowing estimation of exposure in locations without monitors. Similar results have not been reported for larger particles (PM10), which are often the only measures that can be used in locations worldwide that do not have comprehensive PM2.5 monitoring; this also applies to PM estimation of historical exposures. Here we extend this methodology by applying it to 2000–2009 PM10 data from Lombardy, Northern Italy a region with high altitude differences, frequent temperature inversions and stationary fronts. Specifically, by 1) incorporating a model for missing AOD data to deal with non-randomness in the missing data; and 2) modeling interactions between land use and meteorological parameters to better capture space–time interactions. We calibrated AOD data through mixed-effect models regressing PM10 measurements using day-specific random intercepts, and fixed and random AOD and temperature slopes. We used inverse-probability weighting to account for nonrandom AOD missingness, while reducing the dimensionality of spatial and temporal predictors and avoiding selecting different predictors in different locations, as common in land-use regression. We take advantage of associations of grid-cell AOD values with PM10 monitoring located elsewhere and with AOD values in neighboring grid cells to develop grid-cell predictions when AOD is missing. By using ten-fold cross-validation to test the accuracy of our predictions, we found high out-of-sample R2 (R2 = 0.787, year to year variation 0.738–0.818) for days with available AOD data. Even in days with no available AOD, our model performance was comparable (R2 = 0.787, year to year variation 0.736–0.841). Our results demonstrate that PM10 can be reliably predicted using this AOD-based prediction model, even in a geographical area with complex geographic and weather patterns.
Journal: Atmospheric Environment - Volume 74, August 2013, Pages 227–236