کد مقاله | کد نشریه | سال انتشار | مقاله انگلیسی | نسخه تمام متن |
---|---|---|---|---|
4465049 | 1621846 | 2012 | 7 صفحه PDF | دانلود رایگان |
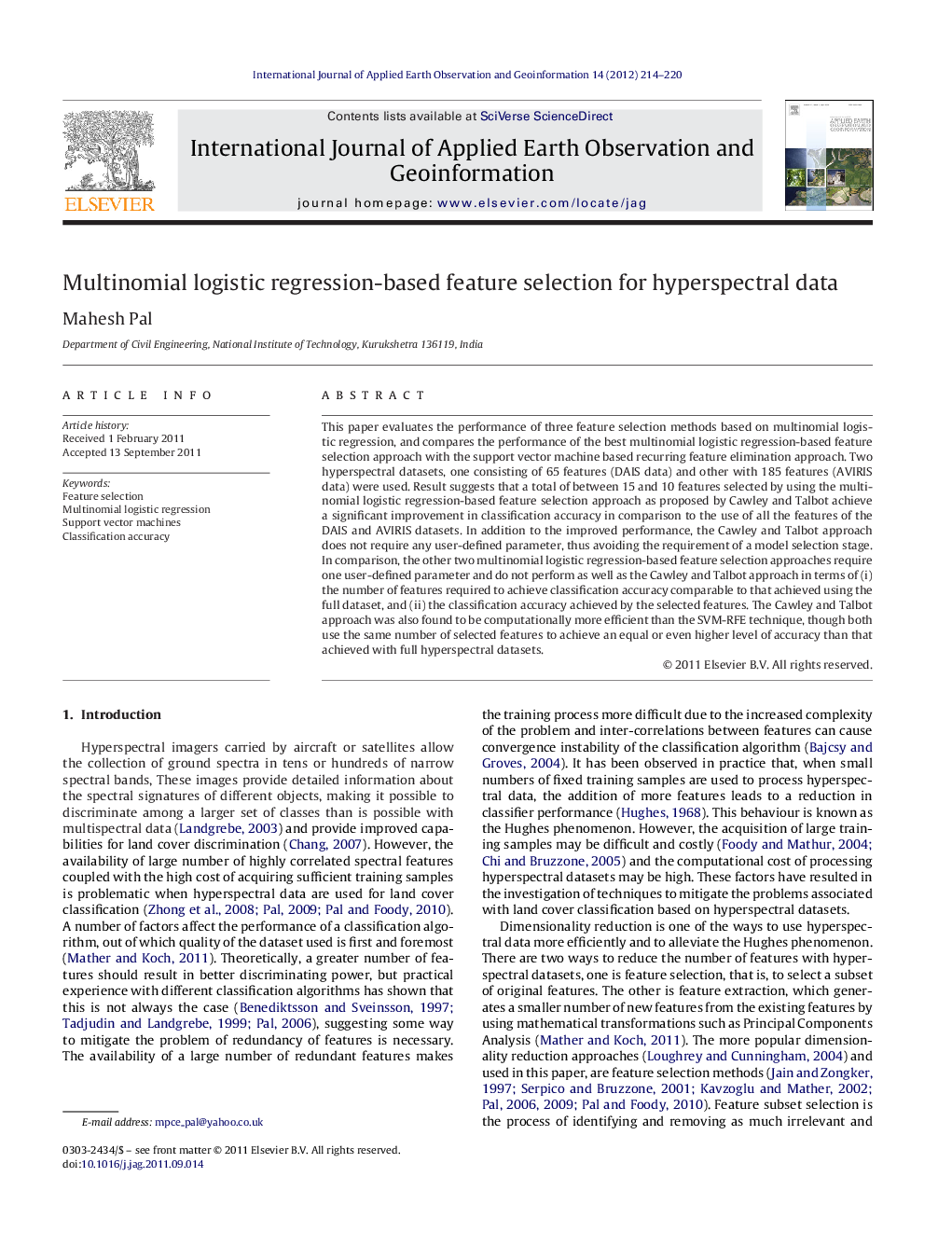
This paper evaluates the performance of three feature selection methods based on multinomial logistic regression, and compares the performance of the best multinomial logistic regression-based feature selection approach with the support vector machine based recurring feature elimination approach. Two hyperspectral datasets, one consisting of 65 features (DAIS data) and other with 185 features (AVIRIS data) were used. Result suggests that a total of between 15 and 10 features selected by using the multinomial logistic regression-based feature selection approach as proposed by Cawley and Talbot achieve a significant improvement in classification accuracy in comparison to the use of all the features of the DAIS and AVIRIS datasets. In addition to the improved performance, the Cawley and Talbot approach does not require any user-defined parameter, thus avoiding the requirement of a model selection stage. In comparison, the other two multinomial logistic regression-based feature selection approaches require one user-defined parameter and do not perform as well as the Cawley and Talbot approach in terms of (i) the number of features required to achieve classification accuracy comparable to that achieved using the full dataset, and (ii) the classification accuracy achieved by the selected features. The Cawley and Talbot approach was also found to be computationally more efficient than the SVM-RFE technique, though both use the same number of selected features to achieve an equal or even higher level of accuracy than that achieved with full hyperspectral datasets.
► We compare three multinomial logistic regression based feature selection approaches using hyperspectral data.
► The approach proposed by Cawley and Talbot (2006) works well with small set of selected features.
► Selected features achieve significant improvement in classification accuracy in comparison to full hyperspectral dataset.
► Proposed approach does not require model selection stage (i.e. require no user-defined parameter).
► Proposed approach works well in comparison to SVM-RFE in terms of computational cost.
Journal: International Journal of Applied Earth Observation and Geoinformation - Volume 14, Issue 1, February 2012, Pages 214–220