کد مقاله | کد نشریه | سال انتشار | مقاله انگلیسی | نسخه تمام متن |
---|---|---|---|---|
4525972 | 1323805 | 2011 | 11 صفحه PDF | دانلود رایگان |
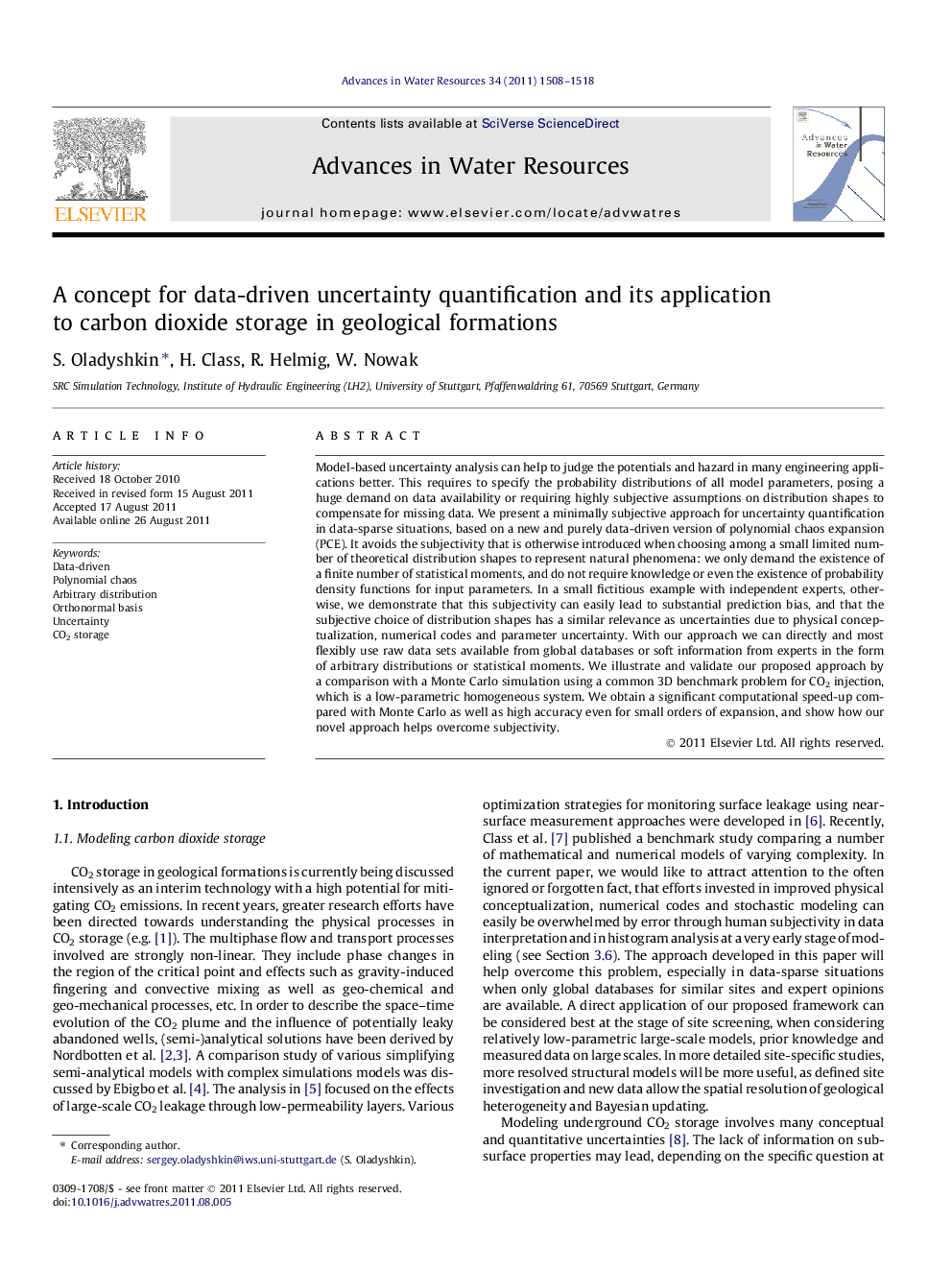
Model-based uncertainty analysis can help to judge the potentials and hazard in many engineering applications better. This requires to specify the probability distributions of all model parameters, posing a huge demand on data availability or requiring highly subjective assumptions on distribution shapes to compensate for missing data. We present a minimally subjective approach for uncertainty quantification in data-sparse situations, based on a new and purely data-driven version of polynomial chaos expansion (PCE). It avoids the subjectivity that is otherwise introduced when choosing among a small limited number of theoretical distribution shapes to represent natural phenomena: we only demand the existence of a finite number of statistical moments, and do not require knowledge or even the existence of probability density functions for input parameters. In a small fictitious example with independent experts, otherwise, we demonstrate that this subjectivity can easily lead to substantial prediction bias, and that the subjective choice of distribution shapes has a similar relevance as uncertainties due to physical conceptualization, numerical codes and parameter uncertainty. With our approach we can directly and most flexibly use raw data sets available from global databases or soft information from experts in the form of arbitrary distributions or statistical moments. We illustrate and validate our proposed approach by a comparison with a Monte Carlo simulation using a common 3D benchmark problem for CO2 injection, which is a low-parametric homogeneous system. We obtain a significant computational speed-up compared with Monte Carlo as well as high accuracy even for small orders of expansion, and show how our novel approach helps overcome subjectivity.
► Subjectivity can easily lead to substantial prediction bias.
► Stochastic modeling approaches must be able to directly employ raw data.
► Data-driven approach leads to minimally subjective approach for probabilistic risk assessment.
► Purely data-driven version of polynomial chaos expansion applied to carbon dioxide storage.
► Significant computational speed-up compared to Monte Carlo.
Journal: Advances in Water Resources - Volume 34, Issue 11, November 2011, Pages 1508–1518