کد مقاله | کد نشریه | سال انتشار | مقاله انگلیسی | نسخه تمام متن |
---|---|---|---|---|
4942758 | 1437416 | 2017 | 14 صفحه PDF | دانلود رایگان |
عنوان انگلیسی مقاله ISI
DENSA: An effective negative selection algorithm with flexible boundaries for self-space and dynamic number of detectors
دانلود مقاله + سفارش ترجمه
دانلود مقاله ISI انگلیسی
رایگان برای ایرانیان
کلمات کلیدی
موضوعات مرتبط
مهندسی و علوم پایه
مهندسی کامپیوتر
هوش مصنوعی
پیش نمایش صفحه اول مقاله
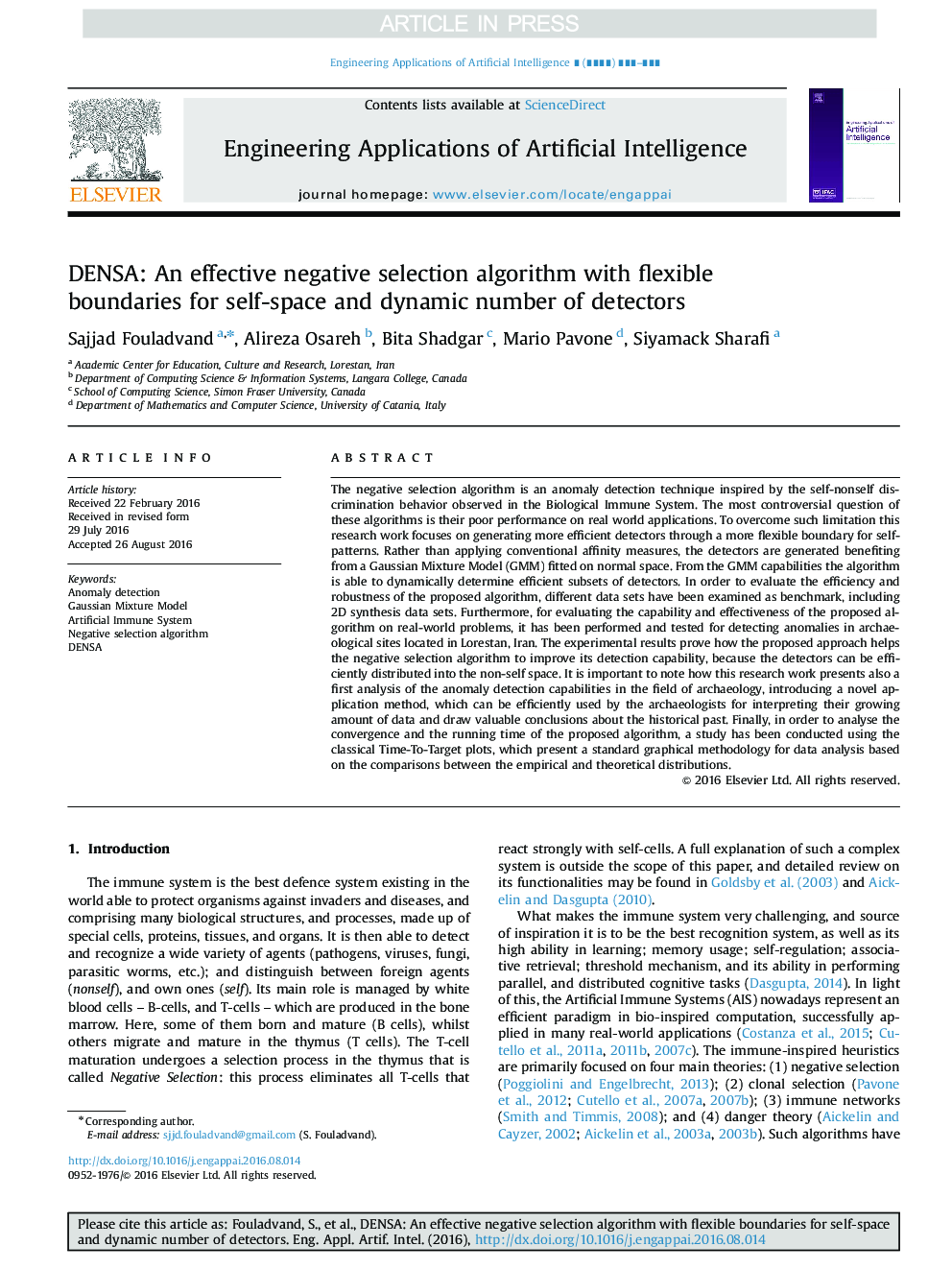
چکیده انگلیسی
The negative selection algorithm is an anomaly detection technique inspired by the self-nonself discrimination behavior observed in the Biological Immune System. The most controversial question of these algorithms is their poor performance on real world applications. To overcome such limitation this research work focuses on generating more efficient detectors through a more flexible boundary for self-patterns. Rather than applying conventional affinity measures, the detectors are generated benefiting from a Gaussian Mixture Model (GMM) fitted on normal space. From the GMM capabilities the algorithm is able to dynamically determine efficient subsets of detectors. In order to evaluate the efficiency and robustness of the proposed algorithm, different data sets have been examined as benchmark, including 2D synthesis data sets. Furthermore, for evaluating the capability and effectiveness of the proposed algorithm on real-world problems, it has been performed and tested for detecting anomalies in archaeological sites located in Lorestan, Iran. The experimental results prove how the proposed approach helps the negative selection algorithm to improve its detection capability, because the detectors can be efficiently distributed into the non-self space. It is important to note how this research work presents also a first analysis of the anomaly detection capabilities in the field of archaeology, introducing a novel application method, which can be efficiently used by the archaeologists for interpreting their growing amount of data and draw valuable conclusions about the historical past. Finally, in order to analyse the convergence and the running time of the proposed algorithm, a study has been conducted using the classical Time-To-Target plots, which present a standard graphical methodology for data analysis based on the comparisons between the empirical and theoretical distributions.
ناشر
Database: Elsevier - ScienceDirect (ساینس دایرکت)
Journal: Engineering Applications of Artificial Intelligence - Volume 62, June 2017, Pages 359-372
Journal: Engineering Applications of Artificial Intelligence - Volume 62, June 2017, Pages 359-372
نویسندگان
Sajjad Fouladvand, Alireza Osareh, Bita Shadgar, Mario Pavone, Siyamack Sharafi,