کد مقاله | کد نشریه | سال انتشار | مقاله انگلیسی | نسخه تمام متن |
---|---|---|---|---|
4945346 | 1438421 | 2017 | 21 صفحه PDF | دانلود رایگان |
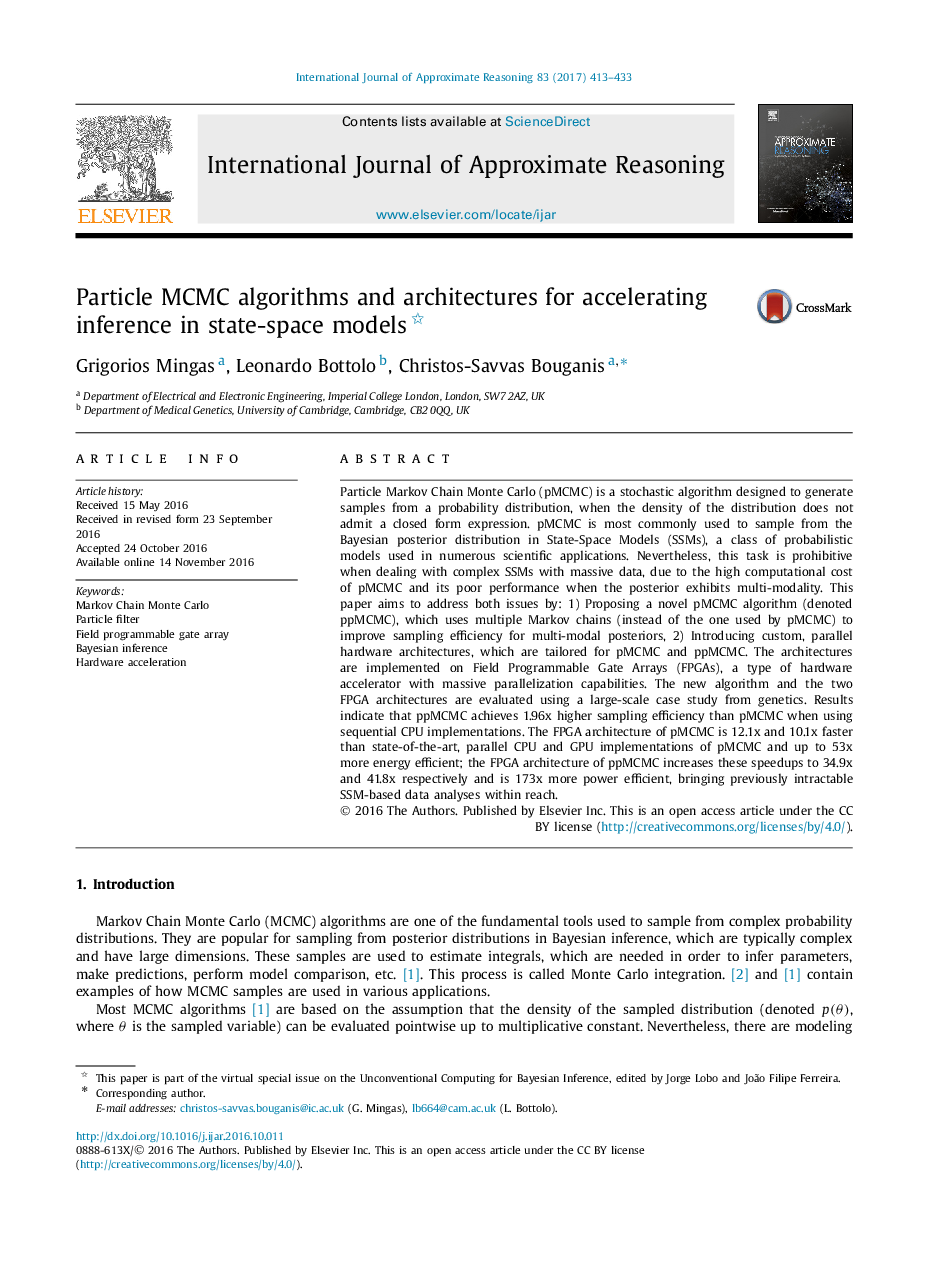
- Novel algorithmic and hardware techniques for fast SSM inference are proposed.
- New algorithm extends applicability of particle MCMC to multi-modal posteriors.
- FPGA architectures exploit particle and chain parallelism to accelerate sampling.
- 42x speedup vs. state-of-the-art CPU/GPU samplers is achieved for large problems.
Particle Markov Chain Monte Carlo (pMCMC) is a stochastic algorithm designed to generate samples from a probability distribution, when the density of the distribution does not admit a closed form expression. pMCMC is most commonly used to sample from the Bayesian posterior distribution in State-Space Models (SSMs), a class of probabilistic models used in numerous scientific applications. Nevertheless, this task is prohibitive when dealing with complex SSMs with massive data, due to the high computational cost of pMCMC and its poor performance when the posterior exhibits multi-modality. This paper aims to address both issues by: 1) Proposing a novel pMCMC algorithm (denoted ppMCMC), which uses multiple Markov chains (instead of the one used by pMCMC) to improve sampling efficiency for multi-modal posteriors, 2) Introducing custom, parallel hardware architectures, which are tailored for pMCMC and ppMCMC. The architectures are implemented on Field Programmable Gate Arrays (FPGAs), a type of hardware accelerator with massive parallelization capabilities. The new algorithm and the two FPGA architectures are evaluated using a large-scale case study from genetics. Results indicate that ppMCMC achieves 1.96x higher sampling efficiency than pMCMC when using sequential CPU implementations. The FPGA architecture of pMCMC is 12.1x and 10.1x faster than state-of-the-art, parallel CPU and GPU implementations of pMCMC and up to 53x more energy efficient; the FPGA architecture of ppMCMC increases these speedups to 34.9x and 41.8x respectively and is 173x more power efficient, bringing previously intractable SSM-based data analyses within reach.
Journal: International Journal of Approximate Reasoning - Volume 83, April 2017, Pages 413-433