کد مقاله | کد نشریه | سال انتشار | مقاله انگلیسی | نسخه تمام متن |
---|---|---|---|---|
4946477 | 1439291 | 2016 | 31 صفحه PDF | دانلود رایگان |
عنوان انگلیسی مقاله ISI
Global feature selection from microarray data using Lagrange multipliers
دانلود مقاله + سفارش ترجمه
دانلود مقاله ISI انگلیسی
رایگان برای ایرانیان
کلمات کلیدی
موضوعات مرتبط
مهندسی و علوم پایه
مهندسی کامپیوتر
هوش مصنوعی
پیش نمایش صفحه اول مقاله
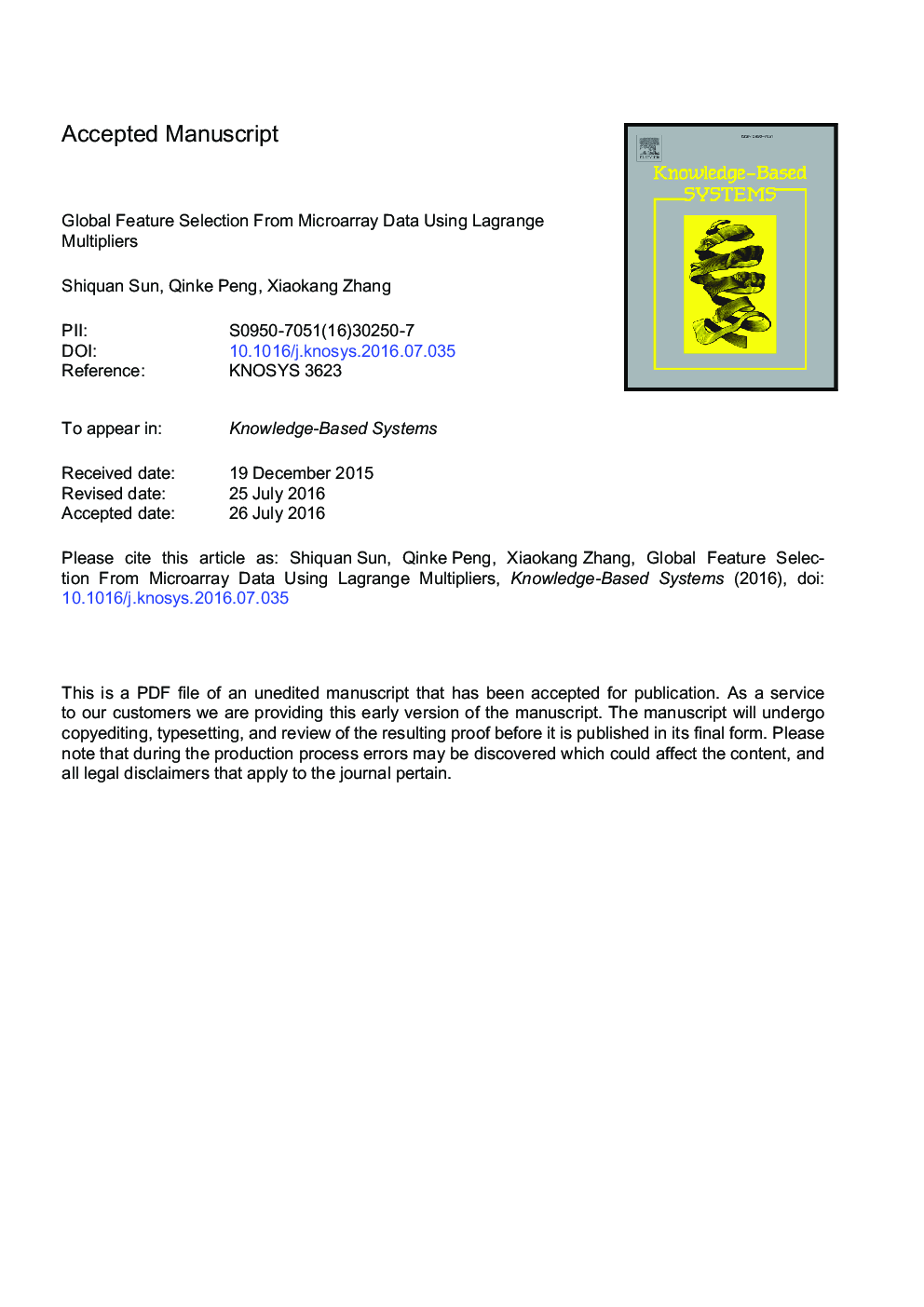
چکیده انگلیسی
In this paper, we present a global feature selection method based on semidefinite programming model which is relaxed from the quadratic programming model with maximizing feature relevance and minimizing feature redundancy. The main advantage of relaxation is that the matrix in mathematical model only requires symmetric matrix rather than positive (or semi) definite matrix. In semidefinite programming model, each feature has one constraint condition to restrict the objective function of feature selection problem. Herein, another trick in this paper is that we utilize Lagrange multiplier as proxy measurement to identify the discriminative features instead of solving a feasible solution for the original max-cut problem. The proposed method is compared with several popular feature selection methods on seven microarray data sets. The results demonstrate that our method outperforms the others on most data sets, especially for the two hard feature selection data sets, Beast(Wang) and Medulloblastoma.
ناشر
Database: Elsevier - ScienceDirect (ساینس دایرکت)
Journal: Knowledge-Based Systems - Volume 110, 15 October 2016, Pages 267-274
Journal: Knowledge-Based Systems - Volume 110, 15 October 2016, Pages 267-274
نویسندگان
Shiquan Sun, Qinke Peng, Xiaokang Zhang,