کد مقاله | کد نشریه | سال انتشار | مقاله انگلیسی | نسخه تمام متن |
---|---|---|---|---|
494698 | 862802 | 2016 | 13 صفحه PDF | دانلود رایگان |
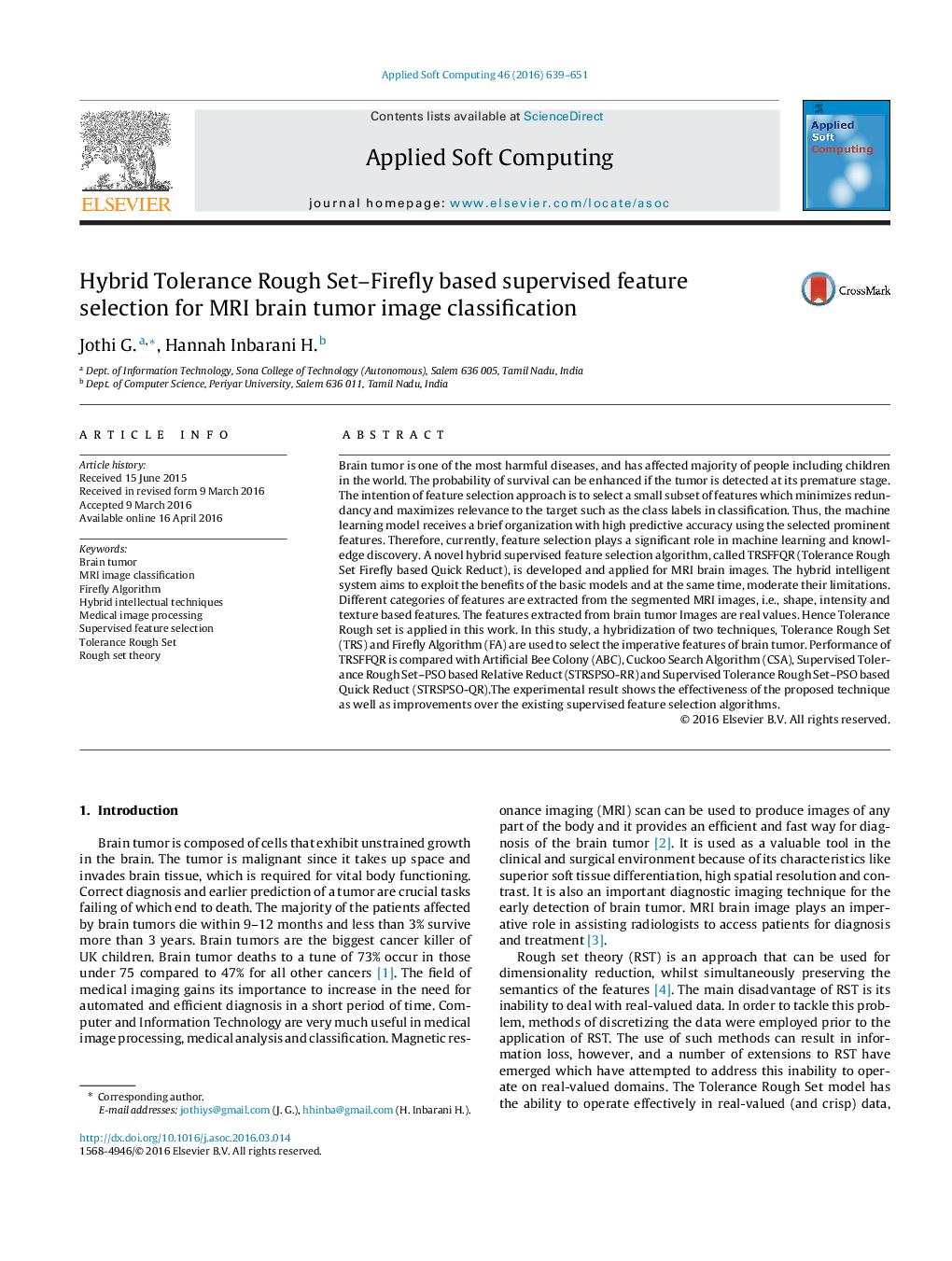
Brain tumor is one of the most harmful diseases, and has affected majority of people including children in the world. The probability of survival can be enhanced if the tumor is detected at its premature stage. The intention of feature selection approach is to select a small subset of features which minimizes redundancy and maximizes relevance to the target such as the class labels in classification. Thus, the machine learning model receives a brief organization with high predictive accuracy using the selected prominent features. Therefore, currently, feature selection plays a significant role in machine learning and knowledge discovery. A novel hybrid supervised feature selection algorithm, called TRSFFQR (Tolerance Rough Set Firefly based Quick Reduct), is developed and applied for MRI brain images. The hybrid intelligent system aims to exploit the benefits of the basic models and at the same time, moderate their limitations. Different categories of features are extracted from the segmented MRI images, i.e., shape, intensity and texture based features. The features extracted from brain tumor Images are real values. Hence Tolerance Rough set is applied in this work. In this study, a hybridization of two techniques, Tolerance Rough Set (TRS) and Firefly Algorithm (FA) are used to select the imperative features of brain tumor. Performance of TRSFFQR is compared with Artificial Bee Colony (ABC), Cuckoo Search Algorithm (CSA), Supervised Tolerance Rough Set–PSO based Relative Reduct (STRSPSO-RR) and Supervised Tolerance Rough Set–PSO based Quick Reduct (STRSPSO-QR).The experimental result shows the effectiveness of the proposed technique as well as improvements over the existing supervised feature selection algorithms.
Journal: Applied Soft Computing - Volume 46, September 2016, Pages 639–651