کد مقاله | کد نشریه | سال انتشار | مقاله انگلیسی | نسخه تمام متن |
---|---|---|---|---|
4947649 | 1439593 | 2017 | 20 صفحه PDF | دانلود رایگان |
عنوان انگلیسی مقاله ISI
â2,1 norm regularized multi-kernel based joint nonlinear feature selection and over-sampling for imbalanced data classification
دانلود مقاله + سفارش ترجمه
دانلود مقاله ISI انگلیسی
رایگان برای ایرانیان
کلمات کلیدی
موضوعات مرتبط
مهندسی و علوم پایه
مهندسی کامپیوتر
هوش مصنوعی
پیش نمایش صفحه اول مقاله
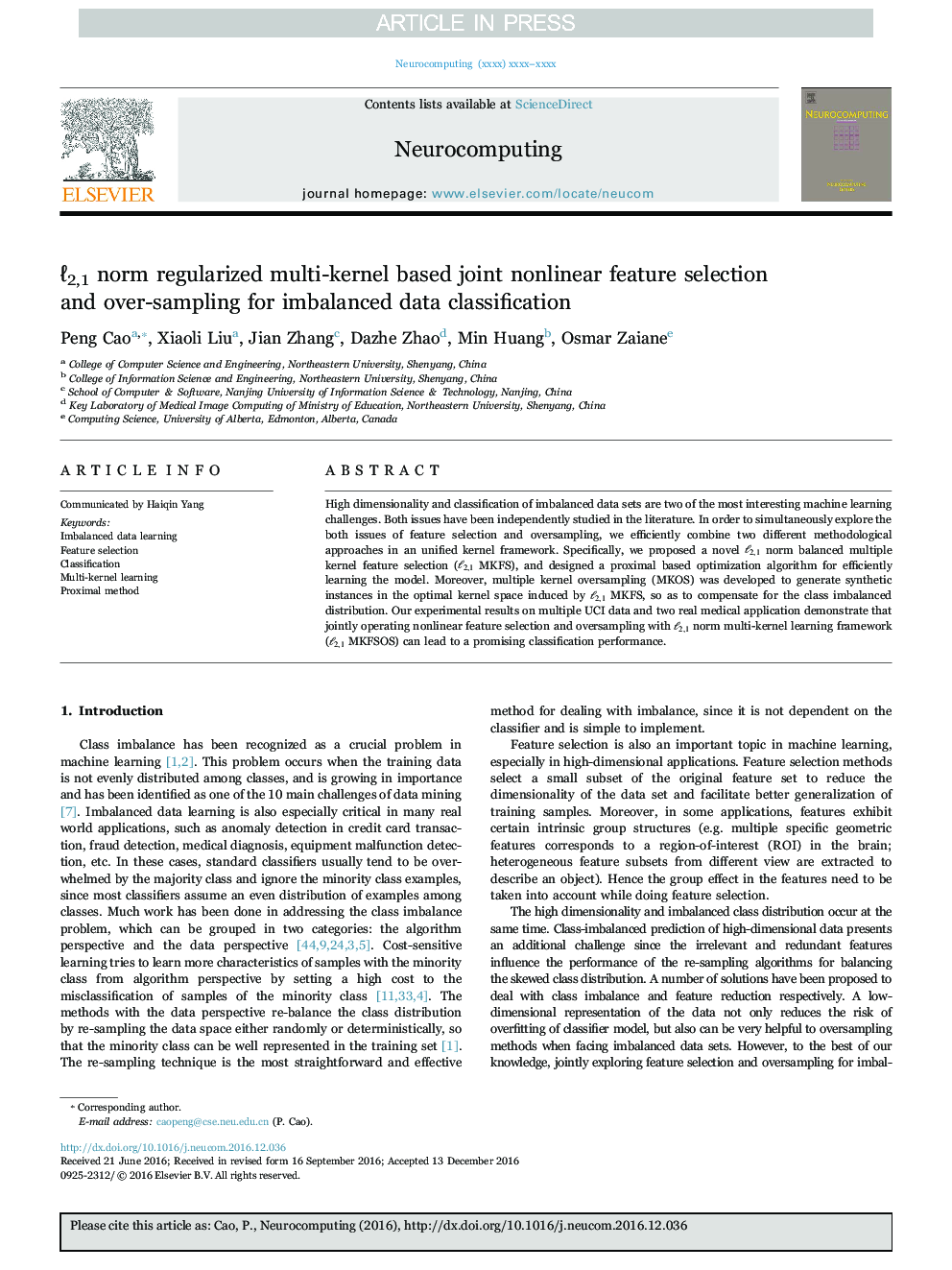
چکیده انگلیسی
High dimensionality and classification of imbalanced data sets are two of the most interesting machine learning challenges. Both issues have been independently studied in the literature. In order to simultaneously explore the both issues of feature selection and oversampling, we efficiently combine two different methodological approaches in an unified kernel framework. Specifically, we proposed a novel â2,1 norm balanced multiple kernel feature selection (â2,1 MKFS), and designed a proximal based optimization algorithm for efficiently learning the model. Moreover, multiple kernel oversampling (MKOS) was developed to generate synthetic instances in the optimal kernel space induced by â2,1 MKFS, so as to compensate for the class imbalanced distribution. Our experimental results on multiple UCI data and two real medical application demonstrate that jointly operating nonlinear feature selection and oversampling with â2,1 norm multi-kernel learning framework (â2,1 MKFSOS) can lead to a promising classification performance.
ناشر
Database: Elsevier - ScienceDirect (ساینس دایرکت)
Journal: Neurocomputing - Volume 234, 19 April 2017, Pages 38-57
Journal: Neurocomputing - Volume 234, 19 April 2017, Pages 38-57
نویسندگان
Peng Cao, Xiaoli Liu, Jian Zhang, Dazhe Zhao, Min Huang, Osmar Zaiane,