کد مقاله | کد نشریه | سال انتشار | مقاله انگلیسی | نسخه تمام متن |
---|---|---|---|---|
4947652 | 1439593 | 2017 | 30 صفحه PDF | دانلود رایگان |
عنوان انگلیسی مقاله ISI
Unsupervised learning of sensor topologies for improving activity recognition in smart environments
دانلود مقاله + سفارش ترجمه
دانلود مقاله ISI انگلیسی
رایگان برای ایرانیان
کلمات کلیدی
موضوعات مرتبط
مهندسی و علوم پایه
مهندسی کامپیوتر
هوش مصنوعی
پیش نمایش صفحه اول مقاله
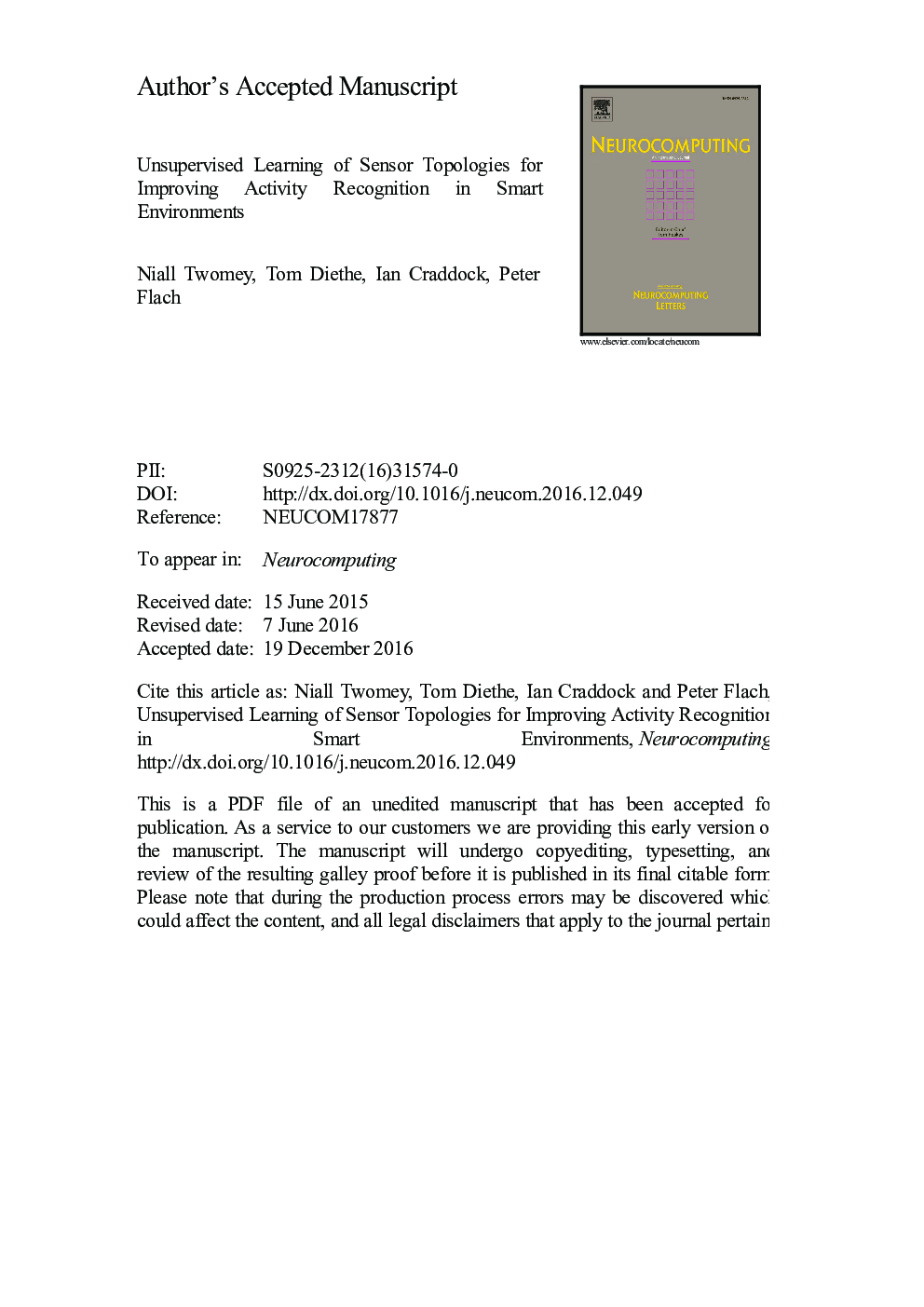
چکیده انگلیسی
There has been significant recent interest in sensing systems and 'smart environments', with a number of longitudinal studies in this area. Typically the goal of these studies is to develop methods to predict, at any one moment of time, the activity or activities that the resident(s) of the home are engaged in, which may in turn be used for determining normal or abnormal patterns of behaviour (e.g. in a health-care setting). Classification algorithms, such as Conditional Random Field (CRFs), typically consider sensor activations as features but these are often treated as if they were independent, which in general they are not. Our hypothesis is that learning patterns based on combinations of sensors will be more powerful than single sensors alone. The exhaustive approach - to take all possible combinations of sensors and learn classifier weights for each combination - is clearly computationally prohibitive. We show that through the application of signal processing and information-theoretic techniques we can learn about the sensor topology in the home (i.e. learn an adjacency matrix) which enables us to determine the combinations of sensors that will be useful for classification ahead of time. As a result we can achieve classification performance better than that of the exhaustive approach, whilst only incurring a small cost in terms of computational resources. We demonstrate our results on several datasets, showing that our method is robust in terms of variations in the layout and the number of residents in the house. Furthermore, we have incorporated the adjacency matrix into the CRF learning framework and have shown that it can improve performance over multiple baselines.
ناشر
Database: Elsevier - ScienceDirect (ساینس دایرکت)
Journal: Neurocomputing - Volume 234, 19 April 2017, Pages 93-106
Journal: Neurocomputing - Volume 234, 19 April 2017, Pages 93-106
نویسندگان
Niall Twomey, Tom Diethe, Ian Craddock, Peter Flach,