کد مقاله | کد نشریه | سال انتشار | مقاله انگلیسی | نسخه تمام متن |
---|---|---|---|---|
4947775 | 1439590 | 2017 | 10 صفحه PDF | دانلود رایگان |
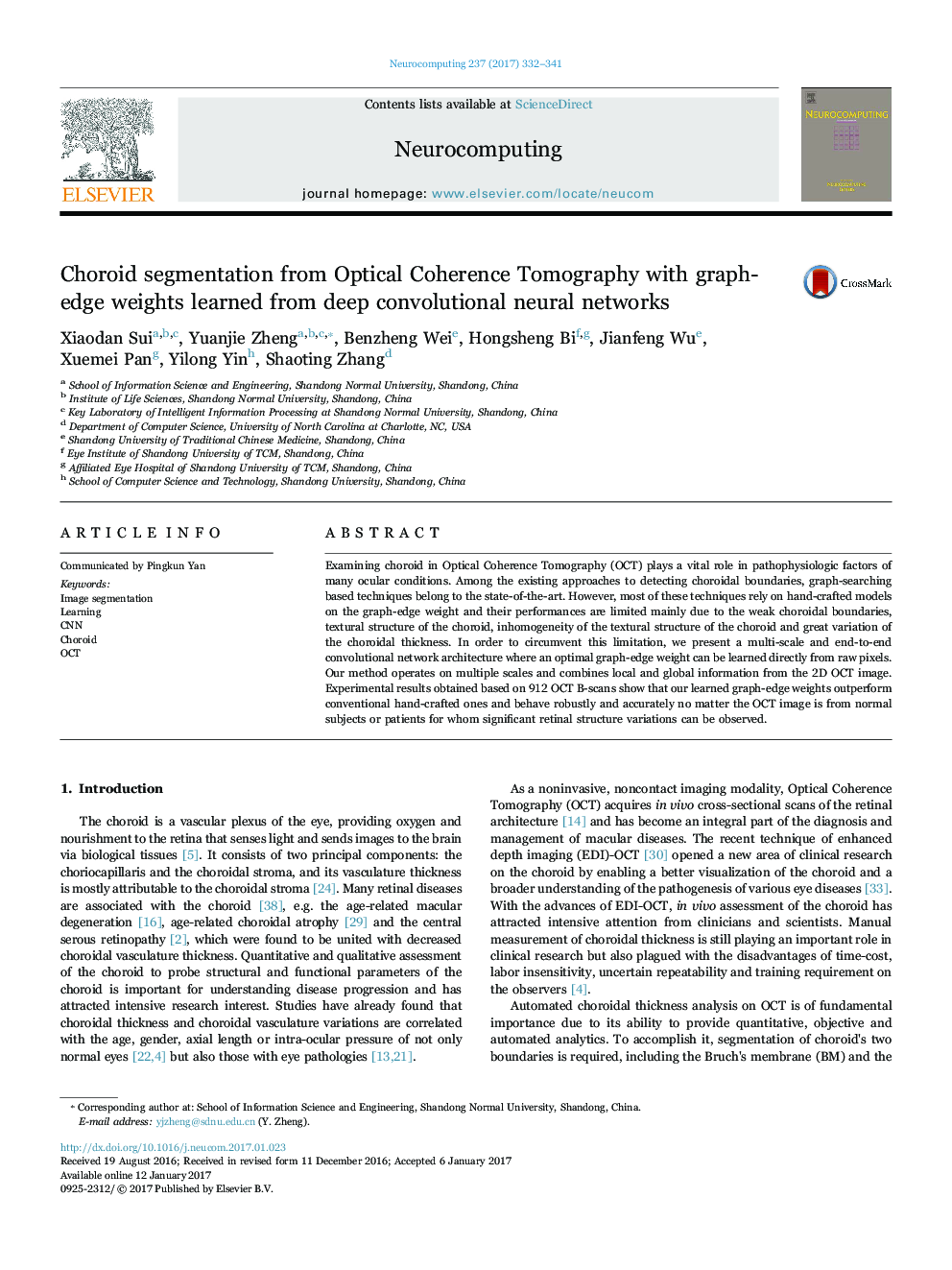
Examining choroid in Optical Coherence Tomography (OCT) plays a vital role in pathophysiologic factors of many ocular conditions. Among the existing approaches to detecting choroidal boundaries, graph-searching based techniques belong to the state-of-the-art. However, most of these techniques rely on hand-crafted models on the graph-edge weight and their performances are limited mainly due to the weak choroidal boundaries, textural structure of the choroid, inhomogeneity of the textural structure of the choroid and great variation of the choroidal thickness. In order to circumvent this limitation, we present a multi-scale and end-to-end convolutional network architecture where an optimal graph-edge weight can be learned directly from raw pixels. Our method operates on multiple scales and combines local and global information from the 2D OCT image. Experimental results obtained based on 912 OCT B-scans show that our learned graph-edge weights outperform conventional hand-crafted ones and behave robustly and accurately no matter the OCT image is from normal subjects or patients for whom significant retinal structure variations can be observed.
Journal: Neurocomputing - Volume 237, 10 May 2017, Pages 332-341