کد مقاله | کد نشریه | سال انتشار | مقاله انگلیسی | نسخه تمام متن |
---|---|---|---|---|
4948097 | 1439607 | 2017 | 8 صفحه PDF | دانلود رایگان |
عنوان انگلیسی مقاله ISI
Graph self-representation method for unsupervised feature selection
دانلود مقاله + سفارش ترجمه
دانلود مقاله ISI انگلیسی
رایگان برای ایرانیان
کلمات کلیدی
موضوعات مرتبط
مهندسی و علوم پایه
مهندسی کامپیوتر
هوش مصنوعی
پیش نمایش صفحه اول مقاله
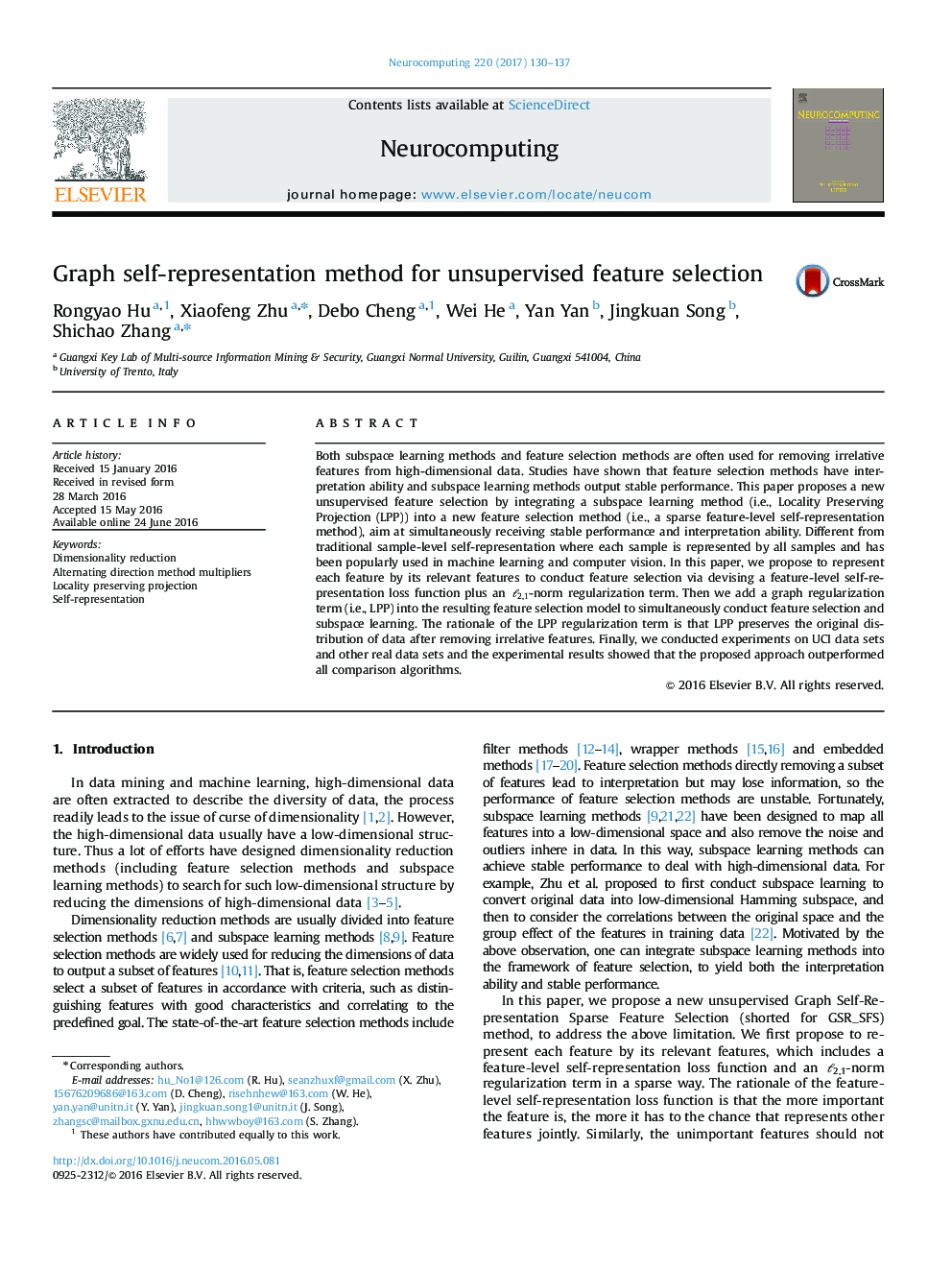
چکیده انگلیسی
Both subspace learning methods and feature selection methods are often used for removing irrelative features from high-dimensional data. Studies have shown that feature selection methods have interpretation ability and subspace learning methods output stable performance. This paper proposes a new unsupervised feature selection by integrating a subspace learning method (i.e., Locality Preserving Projection (LPP)) into a new feature selection method (i.e., a sparse feature-level self-representation method), aim at simultaneously receiving stable performance and interpretation ability. Different from traditional sample-level self-representation where each sample is represented by all samples and has been popularly used in machine learning and computer vision. In this paper, we propose to represent each feature by its relevant features to conduct feature selection via devising a feature-level self-representation loss function plus an â2,1-norm regularization term. Then we add a graph regularization term (i.e., LPP) into the resulting feature selection model to simultaneously conduct feature selection and subspace learning. The rationale of the LPP regularization term is that LPP preserves the original distribution of data after removing irrelative features. Finally, we conducted experiments on UCI data sets and other real data sets and the experimental results showed that the proposed approach outperformed all comparison algorithms.
ناشر
Database: Elsevier - ScienceDirect (ساینس دایرکت)
Journal: Neurocomputing - Volume 220, 12 January 2017, Pages 130-137
Journal: Neurocomputing - Volume 220, 12 January 2017, Pages 130-137
نویسندگان
Rongyao Hu, Xiaofeng Zhu, Debo Cheng, Wei He, Yan Yan, Jingkuan Song, Shichao Zhang,