کد مقاله | کد نشریه | سال انتشار | مقاله انگلیسی | نسخه تمام متن |
---|---|---|---|---|
4948357 | 1439611 | 2016 | 13 صفحه PDF | دانلود رایگان |
عنوان انگلیسی مقاله ISI
Color image classification via quaternion principal component analysis network
دانلود مقاله + سفارش ترجمه
دانلود مقاله ISI انگلیسی
رایگان برای ایرانیان
موضوعات مرتبط
مهندسی و علوم پایه
مهندسی کامپیوتر
هوش مصنوعی
پیش نمایش صفحه اول مقاله
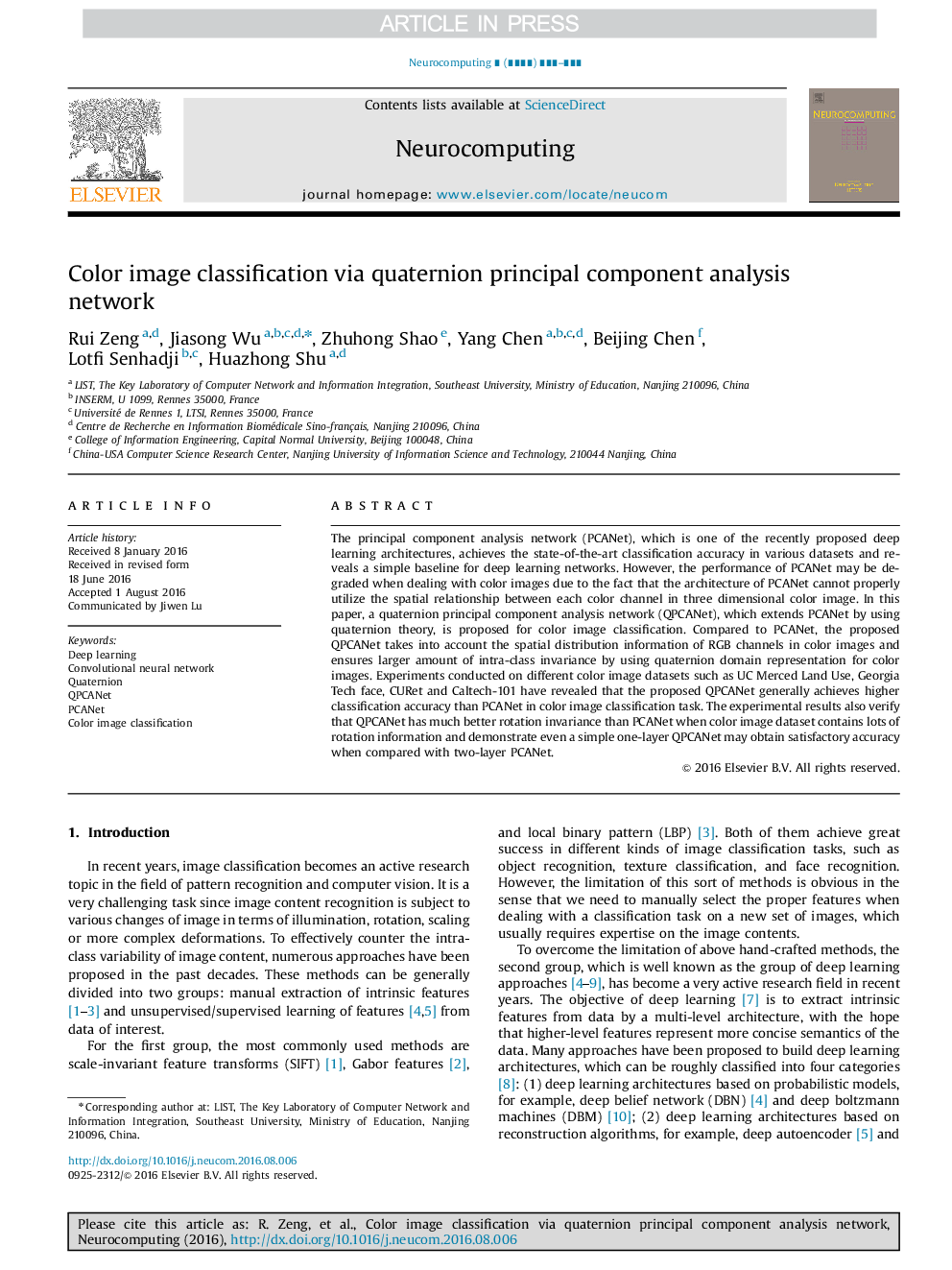
چکیده انگلیسی
The principal component analysis network (PCANet), which is one of the recently proposed deep learning architectures, achieves the state-of-the-art classification accuracy in various datasets and reveals a simple baseline for deep learning networks. However, the performance of PCANet may be degraded when dealing with color images due to the fact that the architecture of PCANet cannot properly utilize the spatial relationship between each color channel in three dimensional color image. In this paper, a quaternion principal component analysis network (QPCANet), which extends PCANet by using quaternion theory, is proposed for color image classification. Compared to PCANet, the proposed QPCANet takes into account the spatial distribution information of RGB channels in color images and ensures larger amount of intra-class invariance by using quaternion domain representation for color images. Experiments conducted on different color image datasets such as UC Merced Land Use, Georgia Tech face, CURet and Caltech-101 have revealed that the proposed QPCANet generally achieves higher classification accuracy than PCANet in color image classification task. The experimental results also verify that QPCANet has much better rotation invariance than PCANet when color image dataset contains lots of rotation information and demonstrate even a simple one-layer QPCANet may obtain satisfactory accuracy when compared with two-layer PCANet.
ناشر
Database: Elsevier - ScienceDirect (ساینس دایرکت)
Journal: Neurocomputing - Volume 216, 5 December 2016, Pages 416-428
Journal: Neurocomputing - Volume 216, 5 December 2016, Pages 416-428
نویسندگان
Rui Zeng, Jiasong Wu, Zhuhong Shao, Yang Chen, Beijing Chen, Lotfi Senhadji, Huazhong Shu,