کد مقاله | کد نشریه | سال انتشار | مقاله انگلیسی | نسخه تمام متن |
---|---|---|---|---|
494995 | 862812 | 2015 | 15 صفحه PDF | دانلود رایگان |
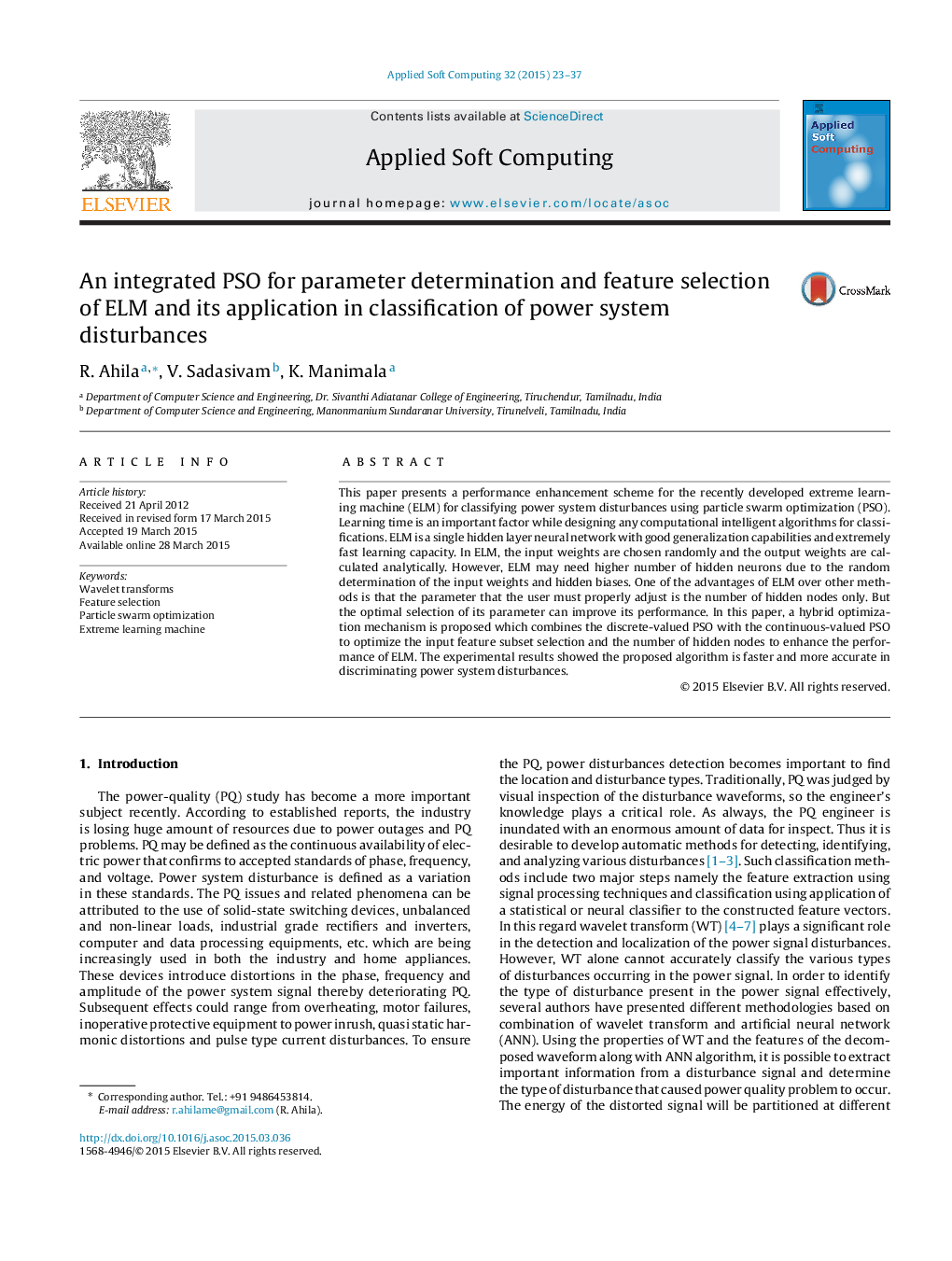
• A new learning method for power system disturbances is introduced using extreme learning machine.
• Simultaneously optimize the feature subset and model selection for the ELM using PSO.
• Proposed method can improve convergence accuracy and generalization performance of ELM.
This paper presents a performance enhancement scheme for the recently developed extreme learning machine (ELM) for classifying power system disturbances using particle swarm optimization (PSO). Learning time is an important factor while designing any computational intelligent algorithms for classifications. ELM is a single hidden layer neural network with good generalization capabilities and extremely fast learning capacity. In ELM, the input weights are chosen randomly and the output weights are calculated analytically. However, ELM may need higher number of hidden neurons due to the random determination of the input weights and hidden biases. One of the advantages of ELM over other methods is that the parameter that the user must properly adjust is the number of hidden nodes only. But the optimal selection of its parameter can improve its performance. In this paper, a hybrid optimization mechanism is proposed which combines the discrete-valued PSO with the continuous-valued PSO to optimize the input feature subset selection and the number of hidden nodes to enhance the performance of ELM. The experimental results showed the proposed algorithm is faster and more accurate in discriminating power system disturbances.
Figure optionsDownload as PowerPoint slide
Journal: Applied Soft Computing - Volume 32, July 2015, Pages 23–37