کد مقاله | کد نشریه | سال انتشار | مقاله انگلیسی | نسخه تمام متن |
---|---|---|---|---|
4968793 | 1449744 | 2017 | 19 صفحه PDF | دانلود رایگان |
عنوان انگلیسی مقاله ISI
Non-convex online robust PCA: Enhance sparsity via âp-norm minimization
دانلود مقاله + سفارش ترجمه
دانلود مقاله ISI انگلیسی
رایگان برای ایرانیان
کلمات کلیدی
موضوعات مرتبط
مهندسی و علوم پایه
مهندسی کامپیوتر
چشم انداز کامپیوتر و تشخیص الگو
پیش نمایش صفحه اول مقاله
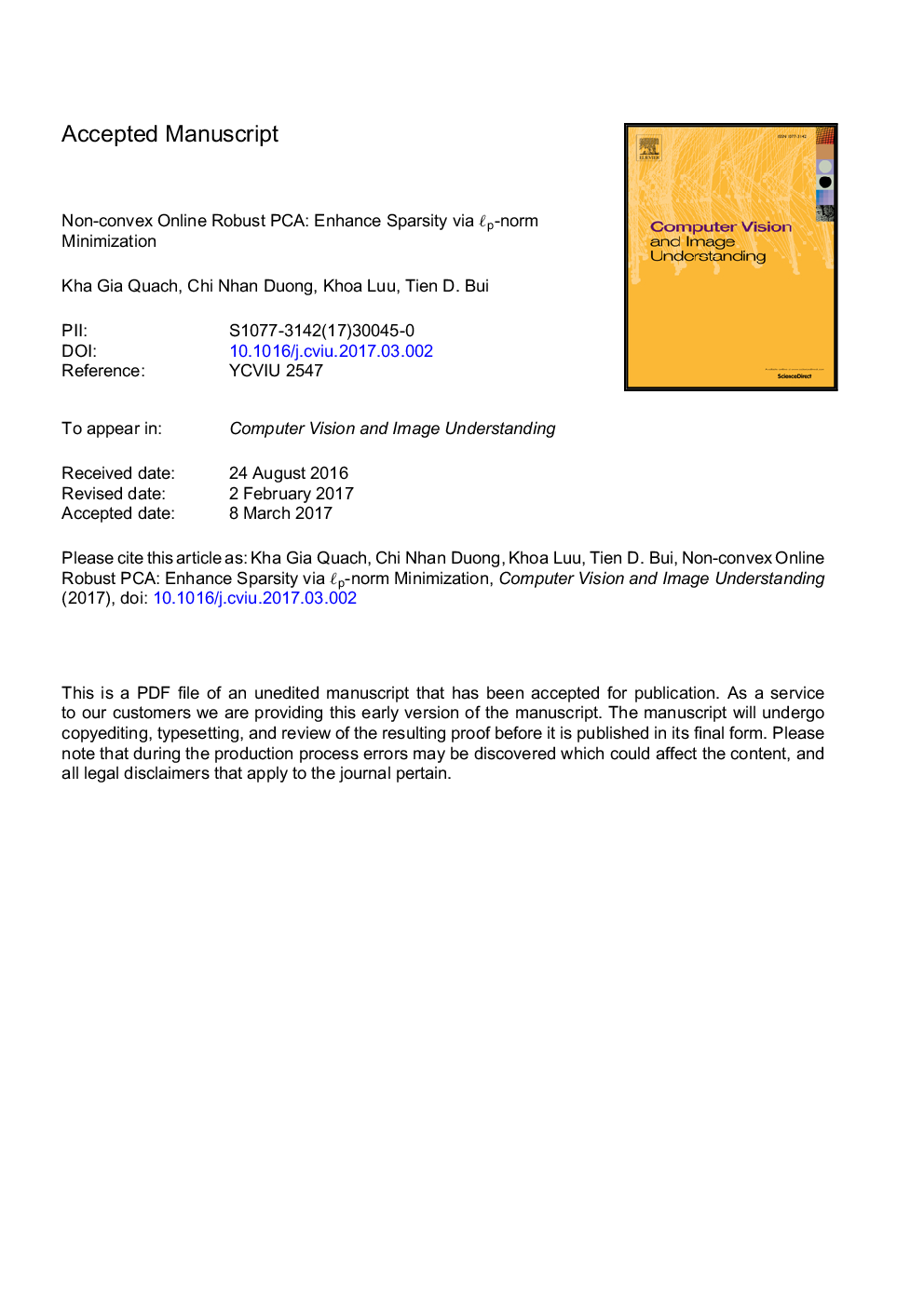
چکیده انگلیسی
Compressive sensing, matrix rank optimization and Robust PCA-based matrix decomposition have an increasing number of non-convex approaches for optimizing the ideal â0-norm sparsity. This paper presents a novel online non-convex âp-norm based Robust PCA (OLP-RPCA) approach, where 0 < p < 1. OLP-RPCA is developed from the offline version LP-RPCA. Our LP-RPCA method uses a new objective function in the Alternating Direction Method of Multipliers (ADMM) framework to efficiently solve the Robust PCA problem. More importantly, our OLP-RPCA method can achieve real-time performance on large-scale data without parallelizing or implementing on a graphics processing unit. We mathematically and empirically show that the computational complexity of our OLP-RPCA algorithm is linear in both the sample dimension and the number of samples. The proposed approaches are evaluated in various applications including Gaussian/non-Gaussian image denoising, face modeling, real-time background subtraction and video inpainting and compared against numerous state-of-the-art low-rank minimization methods to demonstrate the robustness of our algorithms.
ناشر
Database: Elsevier - ScienceDirect (ساینس دایرکت)
Journal: Computer Vision and Image Understanding - Volume 158, May 2017, Pages 126-140
Journal: Computer Vision and Image Understanding - Volume 158, May 2017, Pages 126-140
نویسندگان
Kha Gia Quach, Chi Nhan Duong, Khoa Luu, Tien D. Bui,