کد مقاله | کد نشریه | سال انتشار | مقاله انگلیسی | نسخه تمام متن |
---|---|---|---|---|
5002808 | 1368457 | 2016 | 6 صفحه PDF | دانلود رایگان |
عنوان انگلیسی مقاله ISI
Identification of Mental Workload Using Imbalanced EEG Data and DySMOTE-based Neural Network Approach
دانلود مقاله + سفارش ترجمه
دانلود مقاله ISI انگلیسی
رایگان برای ایرانیان
کلمات کلیدی
موضوعات مرتبط
مهندسی و علوم پایه
سایر رشته های مهندسی
مکانیک محاسباتی
پیش نمایش صفحه اول مقاله
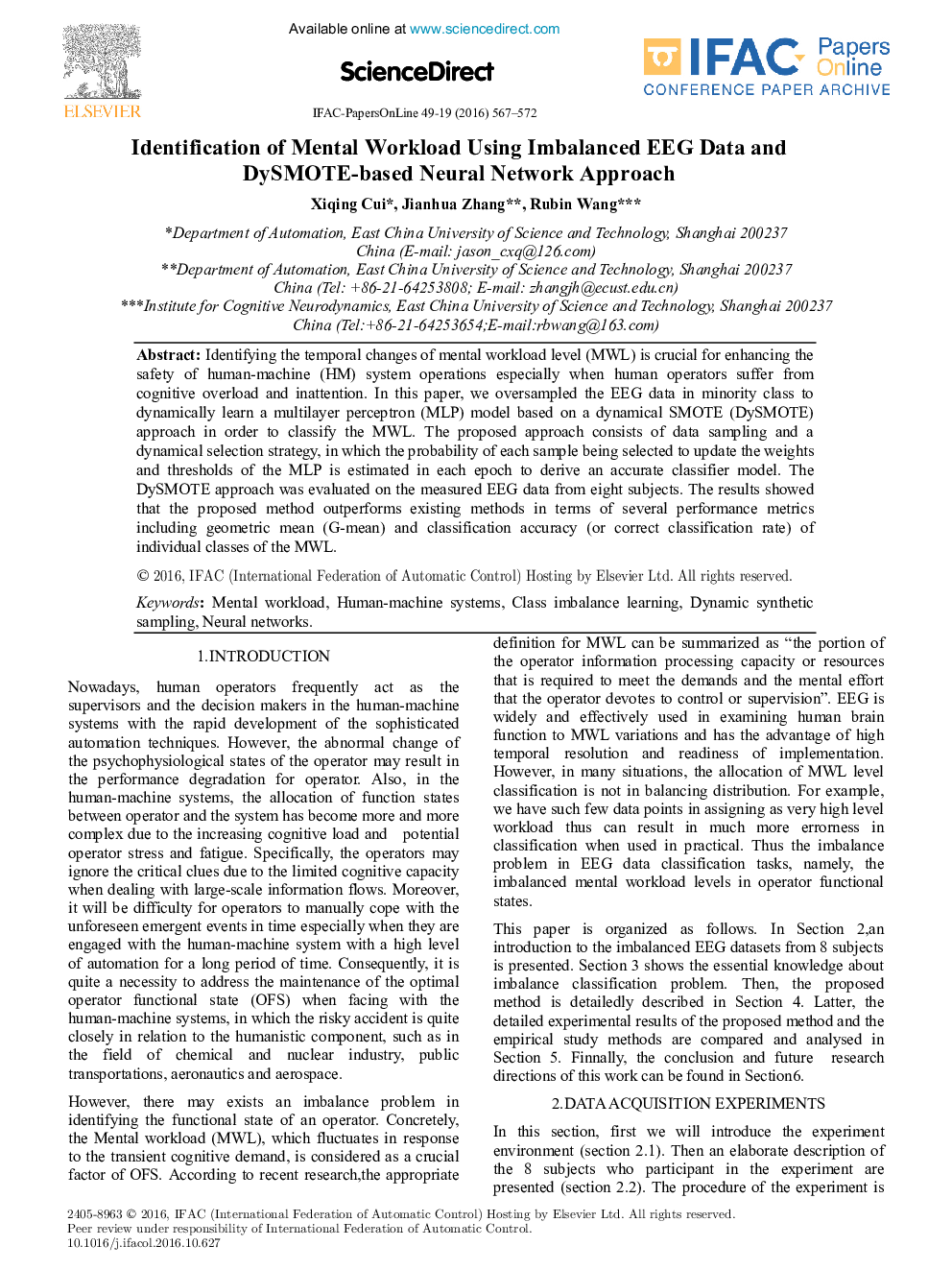
چکیده انگلیسی
Identifying the temporal changes of mental workload level (MWL) is crucial for enhancing the safety of human-machine (HM) system operations especially when human operators suffer from cognitive overload and inattention. In this paper, we oversampled the EEG data in minority class to dynamically learn a multilayer perceptron (MLP) model based on a dynamical SMOTE (DySMOTE) approach in order to classify the MWL. The proposed approach consists of data sampling and a dynamical selection strategy, in which the probability of each sample being selected to update the weights and thresholds of the MLP is estimated in each epoch to derive an accurate classifier model. The DySMOTE approach was evaluated on the measured EEG data from eight subjects. The results showed that the proposed method outperforms existing methods in terms of several performance metrics including geometric mean (G-mean) and classification accuracy (or correct classification rate) of individual classes of the MWL.
ناشر
Database: Elsevier - ScienceDirect (ساینس دایرکت)
Journal: IFAC-PapersOnLine - Volume 49, Issue 19, 2016, Pages 567-572
Journal: IFAC-PapersOnLine - Volume 49, Issue 19, 2016, Pages 567-572
نویسندگان
Xiqing Cui, Jianhua Zhang, Rubin Wang,