کد مقاله | کد نشریه | سال انتشار | مقاله انگلیسی | نسخه تمام متن |
---|---|---|---|---|
5011408 | 1462593 | 2017 | 13 صفحه PDF | دانلود رایگان |
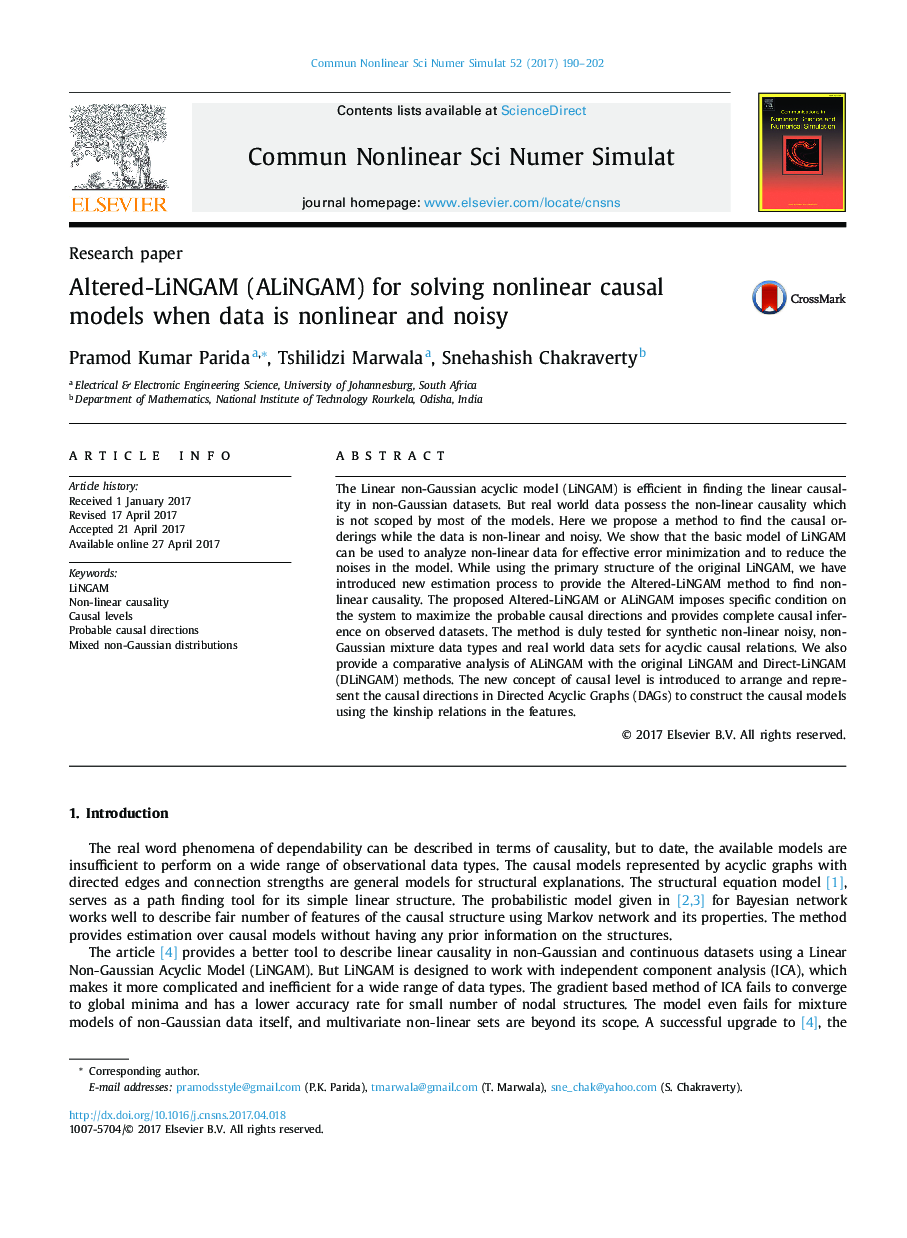
- ALiNGAM is proposed based on LiNGAM, estimates causal directions easily and efficiently in multivariate datasets using Least-Square estimation technique.
- A new criteria of Probable Causal Direction is introduced to find directions in feature sets. Causal level is introduced to arrange, and represent causal directions in graphical structure, to provide causal models.
- ALiNGAM is computationally effective in estimating directions in complex feature sets generated through mixed non-Gaussian distributions with both correlated and uncorrelated feature relations.
- ALiNGAM estimates most number of correct directions and less number of extra directions in the causal models, while other methods of same class produce opposite results.
- Proposed ALiNGAM preserves the criteria of Directed Acyclic Graph (DAG) using Probable Causal Direction and produces acyclic causal models.
The Linear non-Gaussian acyclic model (LiNGAM) is efficient in finding the linear causality in non-Gaussian datasets. But real world data possess the non-linear causality which is not scoped by most of the models. Here we propose a method to find the causal orderings while the data is non-linear and noisy. We show that the basic model of LiNGAM can be used to analyze non-linear data for effective error minimization and to reduce the noises in the model. While using the primary structure of the original LiNGAM, we have introduced new estimation process to provide the Altered-LiNGAM method to find non-linear causality. The proposed Altered-LiNGAM or ALiNGAM imposes specific condition on the system to maximize the probable causal directions and provides complete causal inference on observed datasets. The method is duly tested for synthetic non-linear noisy, non-Gaussian mixture data types and real world data sets for acyclic causal relations. We also provide a comparative analysis of ALiNGAM with the original LiNGAM and Direct-LiNGAM (DLiNGAM) methods. The new concept of causal level is introduced to arrange and represent the causal directions in Directed Acyclic Graphs (DAGs) to construct the causal models using the kinship relations in the features.
Journal: Communications in Nonlinear Science and Numerical Simulation - Volume 52, November 2017, Pages 190-202