کد مقاله | کد نشریه | سال انتشار | مقاله انگلیسی | نسخه تمام متن |
---|---|---|---|---|
525100 | 868887 | 2014 | 16 صفحه PDF | دانلود رایگان |
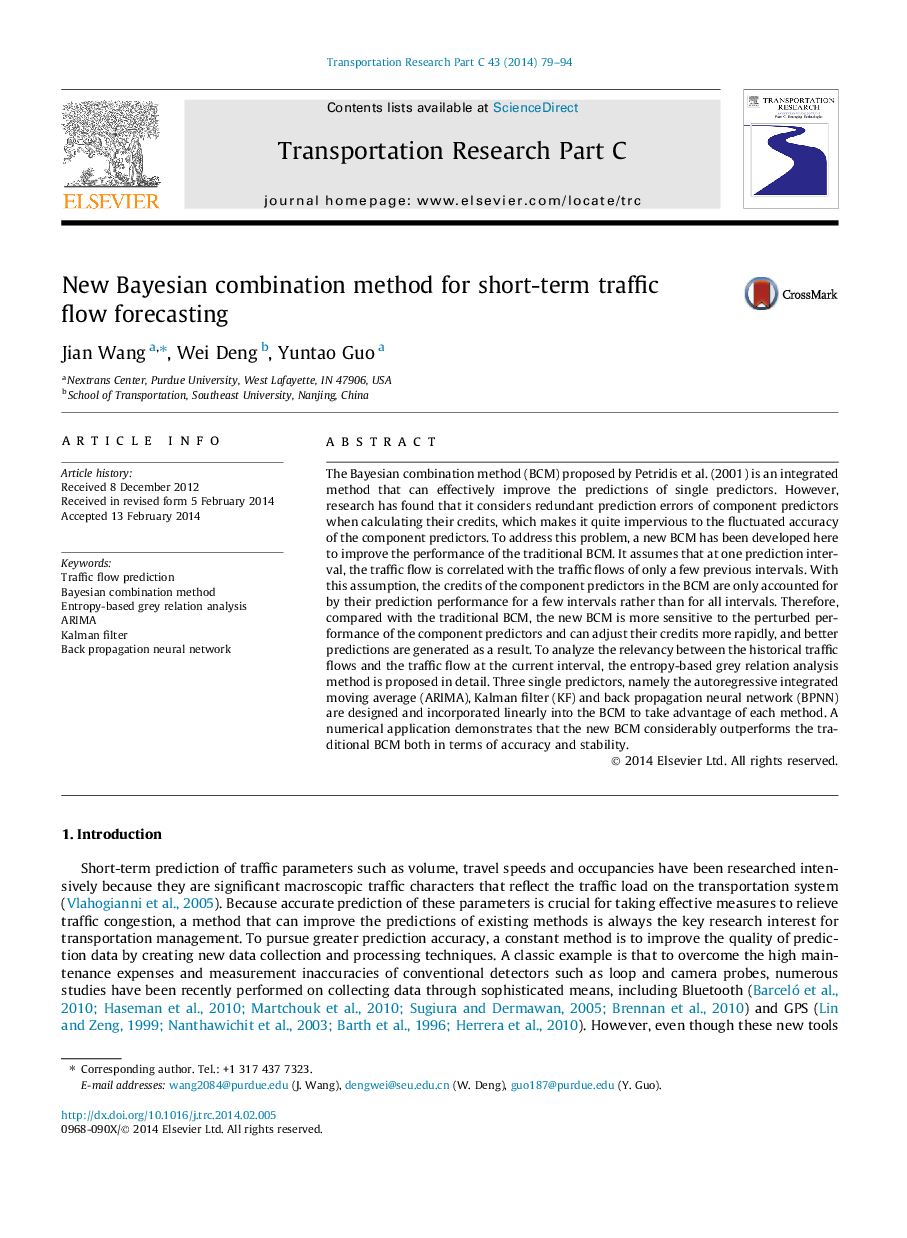
• The deficiency of the traditional Bayesian combination method (BCM) is explicitly presented.
• A new BCM is proposed to improve the deficiency of the traditional BCM.
• The entropy-based grey relation analysis method is put forward for correlation analysis.
• Three predictors are put forward and combined linearly into both traditional and new BCM.
• The new BCM outperforms the traditional BCM considerably both in terms of accuracy and stability.
The Bayesian combination method (BCM) proposed by Petridis et al. (2001) is an integrated method that can effectively improve the predictions of single predictors. However, research has found that it considers redundant prediction errors of component predictors when calculating their credits, which makes it quite impervious to the fluctuated accuracy of the component predictors. To address this problem, a new BCM has been developed here to improve the performance of the traditional BCM. It assumes that at one prediction interval, the traffic flow is correlated with the traffic flows of only a few previous intervals. With this assumption, the credits of the component predictors in the BCM are only accounted for by their prediction performance for a few intervals rather than for all intervals. Therefore, compared with the traditional BCM, the new BCM is more sensitive to the perturbed performance of the component predictors and can adjust their credits more rapidly, and better predictions are generated as a result. To analyze the relevancy between the historical traffic flows and the traffic flow at the current interval, the entropy-based grey relation analysis method is proposed in detail. Three single predictors, namely the autoregressive integrated moving average (ARIMA), Kalman filter (KF) and back propagation neural network (BPNN) are designed and incorporated linearly into the BCM to take advantage of each method. A numerical application demonstrates that the new BCM considerably outperforms the traditional BCM both in terms of accuracy and stability.
Journal: Transportation Research Part C: Emerging Technologies - Volume 43, Part 1, June 2014, Pages 79–94