کد مقاله | کد نشریه | سال انتشار | مقاله انگلیسی | نسخه تمام متن |
---|---|---|---|---|
526207 | 869078 | 2011 | 14 صفحه PDF | دانلود رایگان |
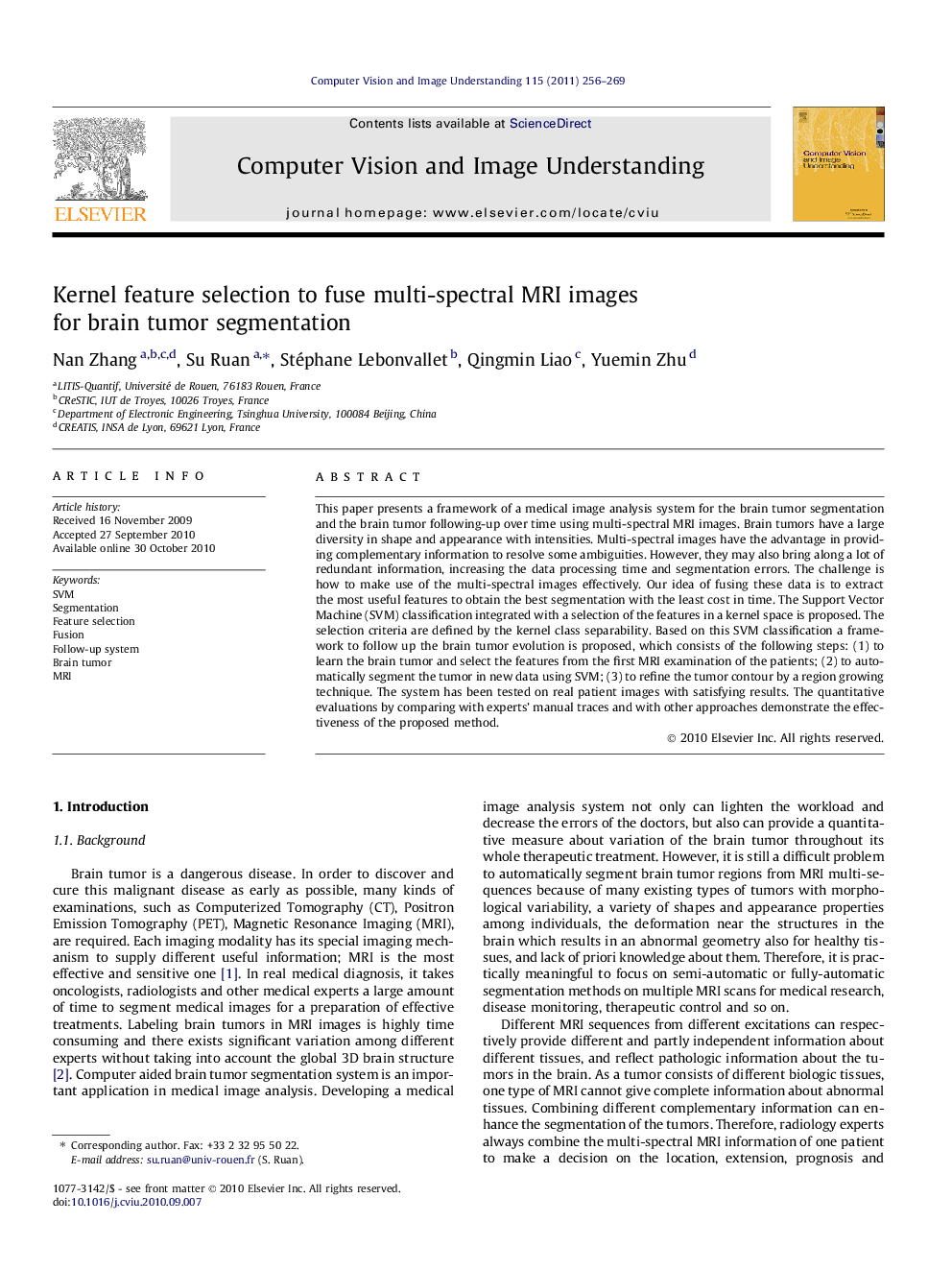
This paper presents a framework of a medical image analysis system for the brain tumor segmentation and the brain tumor following-up over time using multi-spectral MRI images. Brain tumors have a large diversity in shape and appearance with intensities. Multi-spectral images have the advantage in providing complementary information to resolve some ambiguities. However, they may also bring along a lot of redundant information, increasing the data processing time and segmentation errors. The challenge is how to make use of the multi-spectral images effectively. Our idea of fusing these data is to extract the most useful features to obtain the best segmentation with the least cost in time. The Support Vector Machine (SVM) classification integrated with a selection of the features in a kernel space is proposed. The selection criteria are defined by the kernel class separability. Based on this SVM classification a framework to follow up the brain tumor evolution is proposed, which consists of the following steps: (1) to learn the brain tumor and select the features from the first MRI examination of the patients; (2) to automatically segment the tumor in new data using SVM; (3) to refine the tumor contour by a region growing technique. The system has been tested on real patient images with satisfying results. The quantitative evaluations by comparing with experts’ manual traces and with other approaches demonstrate the effectiveness of the proposed method.
Research Highlights
► Fusion of multi-spectral MRI images is based on extraction of the most useful features from these images to obtain the best segmentation.
► The feature selection is carried out in a kernel space with selection criteria defined by the kernel class separability.
► Tumor segmentation is carried out by coupling a Support Vector Machine (SVM) classification with the feature selection.
► A medical image analysis system is proposed to follow up quasi-automatically and quantitatively the brain tumor evolution over time during a therapeutic period.
Journal: Computer Vision and Image Understanding - Volume 115, Issue 2, February 2011, Pages 256–269