کد مقاله | کد نشریه | سال انتشار | مقاله انگلیسی | نسخه تمام متن |
---|---|---|---|---|
527121 | 869290 | 2012 | 14 صفحه PDF | دانلود رایگان |
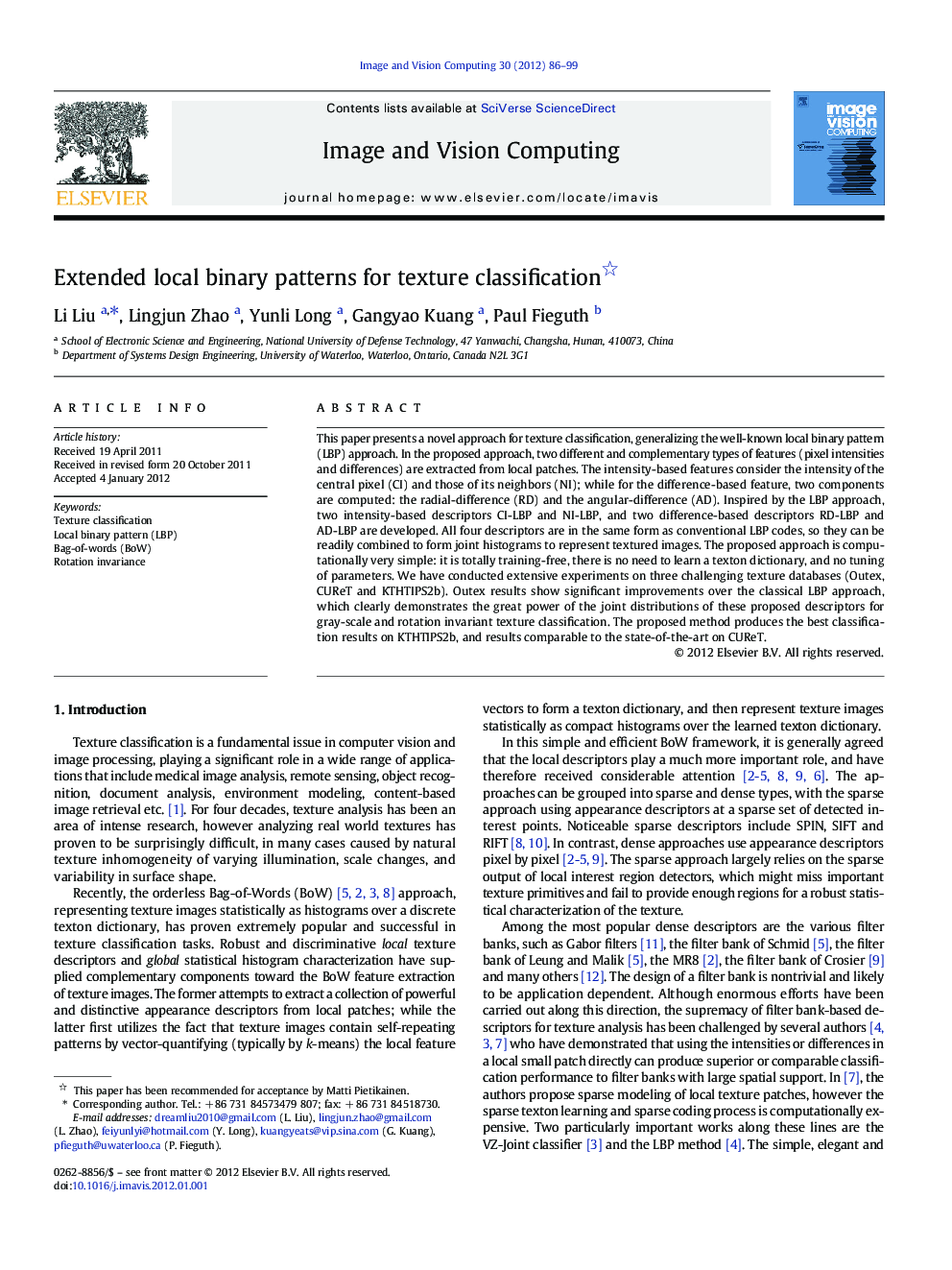
This paper presents a novel approach for texture classification, generalizing the well-known local binary pattern (LBP) approach. In the proposed approach, two different and complementary types of features (pixel intensities and differences) are extracted from local patches. The intensity-based features consider the intensity of the central pixel (CI) and those of its neighbors (NI); while for the difference-based feature, two components are computed: the radial-difference (RD) and the angular-difference (AD). Inspired by the LBP approach, two intensity-based descriptors CI-LBP and NI-LBP, and two difference-based descriptors RD-LBP and AD-LBP are developed. All four descriptors are in the same form as conventional LBP codes, so they can be readily combined to form joint histograms to represent textured images. The proposed approach is computationally very simple: it is totally training-free, there is no need to learn a texton dictionary, and no tuning of parameters. We have conducted extensive experiments on three challenging texture databases (Outex, CUReT and KTHTIPS2b). Outex results show significant improvements over the classical LBP approach, which clearly demonstrates the great power of the joint distributions of these proposed descriptors for gray-scale and rotation invariant texture classification. The proposed method produces the best classification results on KTHTIPS2b, and results comparable to the state-of-the-art on CUReT.
► Generalizing the well-known LBP approach.
► Propose four descriptors which are in the same form as the conventional LBP codes.
► Joint distributions of proposed descriptor prove very powerful.
► Proposed method: computationally very simple, totally training-free; no tuning of parameters.
► Our approach gives very high classification performance on six texture datasets.
Journal: Image and Vision Computing - Volume 30, Issue 2, February 2012, Pages 86–99