کد مقاله | کد نشریه | سال انتشار | مقاله انگلیسی | نسخه تمام متن |
---|---|---|---|---|
527486 | 869328 | 2015 | 12 صفحه PDF | دانلود رایگان |
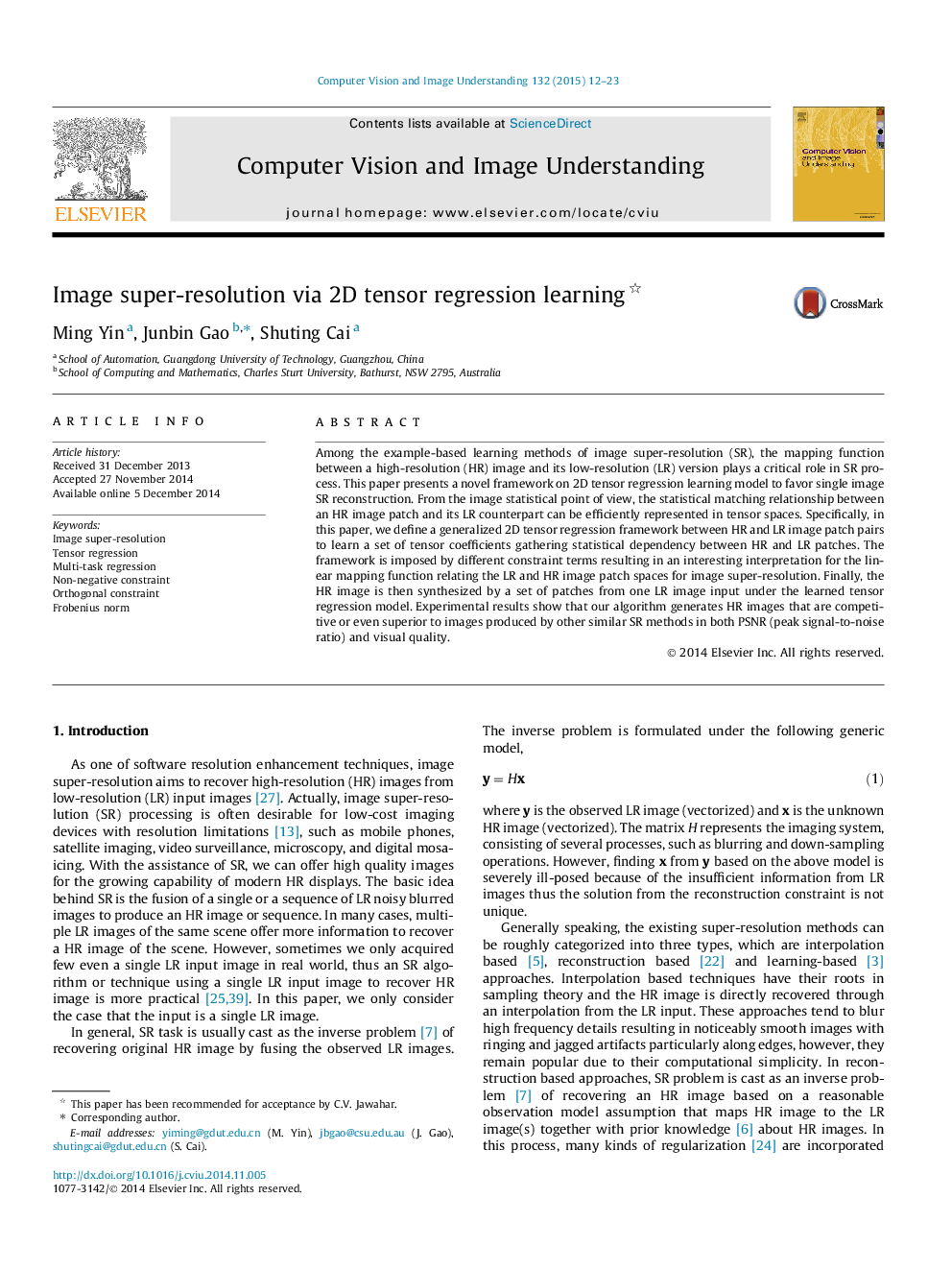
• Presenting a novel framework on 2D tensor regression learning model.
• Proposing three types of regularization to assist the model learning.
• Implementing the optimization algorithms for each specific model.
• Conducting comprehensive experiments on testing images.
• Demonstrating the high performance & efficiency of reconstruction.
Among the example-based learning methods of image super-resolution (SR), the mapping function between a high-resolution (HR) image and its low-resolution (LR) version plays a critical role in SR process. This paper presents a novel framework on 2D tensor regression learning model to favor single image SR reconstruction. From the image statistical point of view, the statistical matching relationship between an HR image patch and its LR counterpart can be efficiently represented in tensor spaces. Specifically, in this paper, we define a generalized 2D tensor regression framework between HR and LR image patch pairs to learn a set of tensor coefficients gathering statistical dependency between HR and LR patches. The framework is imposed by different constraint terms resulting in an interesting interpretation for the linear mapping function relating the LR and HR image patch spaces for image super-resolution. Finally, the HR image is then synthesized by a set of patches from one LR image input under the learned tensor regression model. Experimental results show that our algorithm generates HR images that are competitive or even superior to images produced by other similar SR methods in both PSNR (peak signal-to-noise ratio) and visual quality.
Journal: Computer Vision and Image Understanding - Volume 132, March 2015, Pages 12–23