کد مقاله | کد نشریه | سال انتشار | مقاله انگلیسی | نسخه تمام متن |
---|---|---|---|---|
527558 | 869335 | 2007 | 13 صفحه PDF | دانلود رایگان |
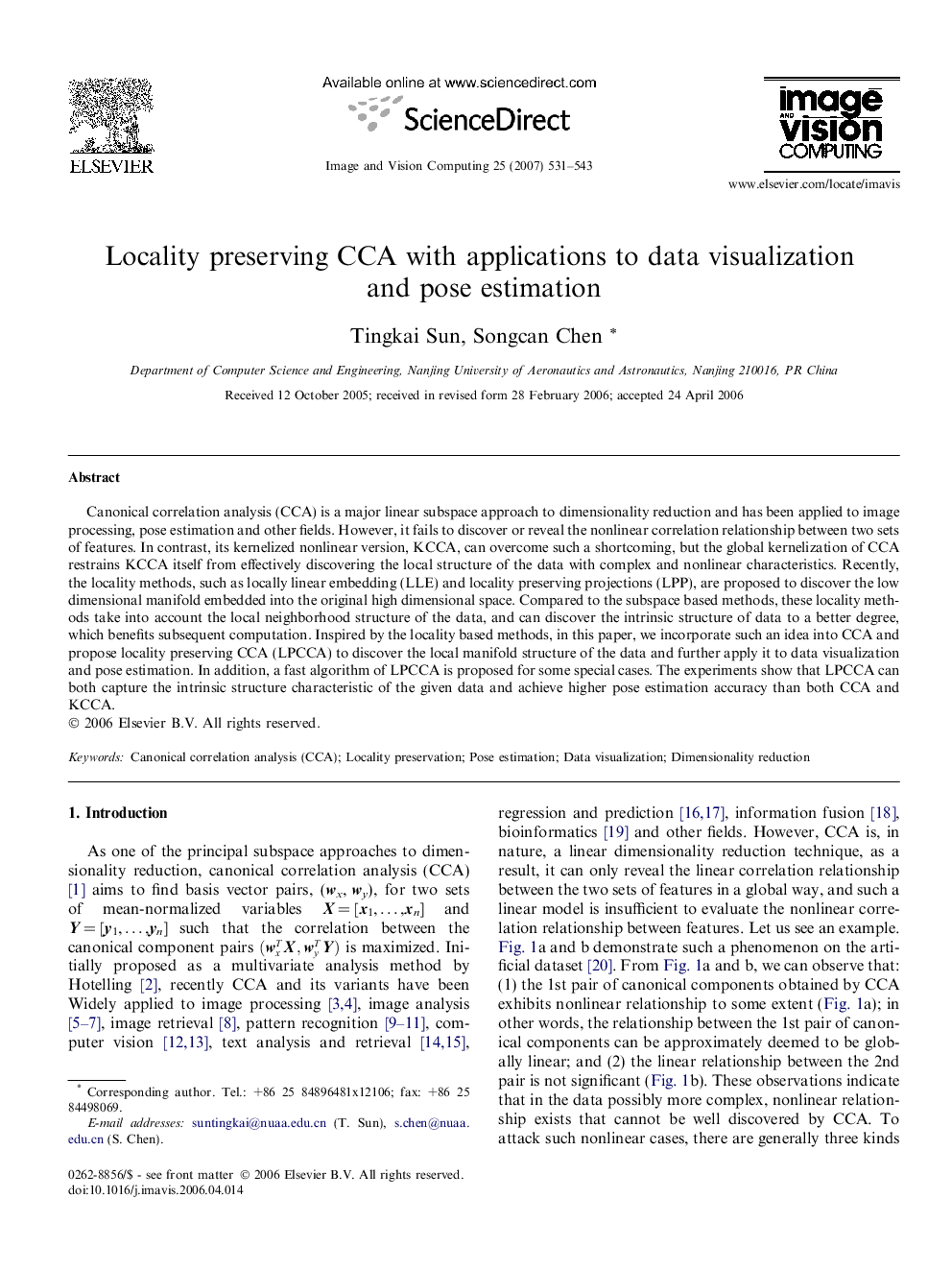
Canonical correlation analysis (CCA) is a major linear subspace approach to dimensionality reduction and has been applied to image processing, pose estimation and other fields. However, it fails to discover or reveal the nonlinear correlation relationship between two sets of features. In contrast, its kernelized nonlinear version, KCCA, can overcome such a shortcoming, but the global kernelization of CCA restrains KCCA itself from effectively discovering the local structure of the data with complex and nonlinear characteristics. Recently, the locality methods, such as locally linear embedding (LLE) and locality preserving projections (LPP), are proposed to discover the low dimensional manifold embedded into the original high dimensional space. Compared to the subspace based methods, these locality methods take into account the local neighborhood structure of the data, and can discover the intrinsic structure of data to a better degree, which benefits subsequent computation. Inspired by the locality based methods, in this paper, we incorporate such an idea into CCA and propose locality preserving CCA (LPCCA) to discover the local manifold structure of the data and further apply it to data visualization and pose estimation. In addition, a fast algorithm of LPCCA is proposed for some special cases. The experiments show that LPCCA can both capture the intrinsic structure characteristic of the given data and achieve higher pose estimation accuracy than both CCA and KCCA.
Journal: Image and Vision Computing - Volume 25, Issue 5, 1 May 2007, Pages 531–543