کد مقاله | کد نشریه | سال انتشار | مقاله انگلیسی | نسخه تمام متن |
---|---|---|---|---|
528291 | 869550 | 2013 | 13 صفحه PDF | دانلود رایگان |
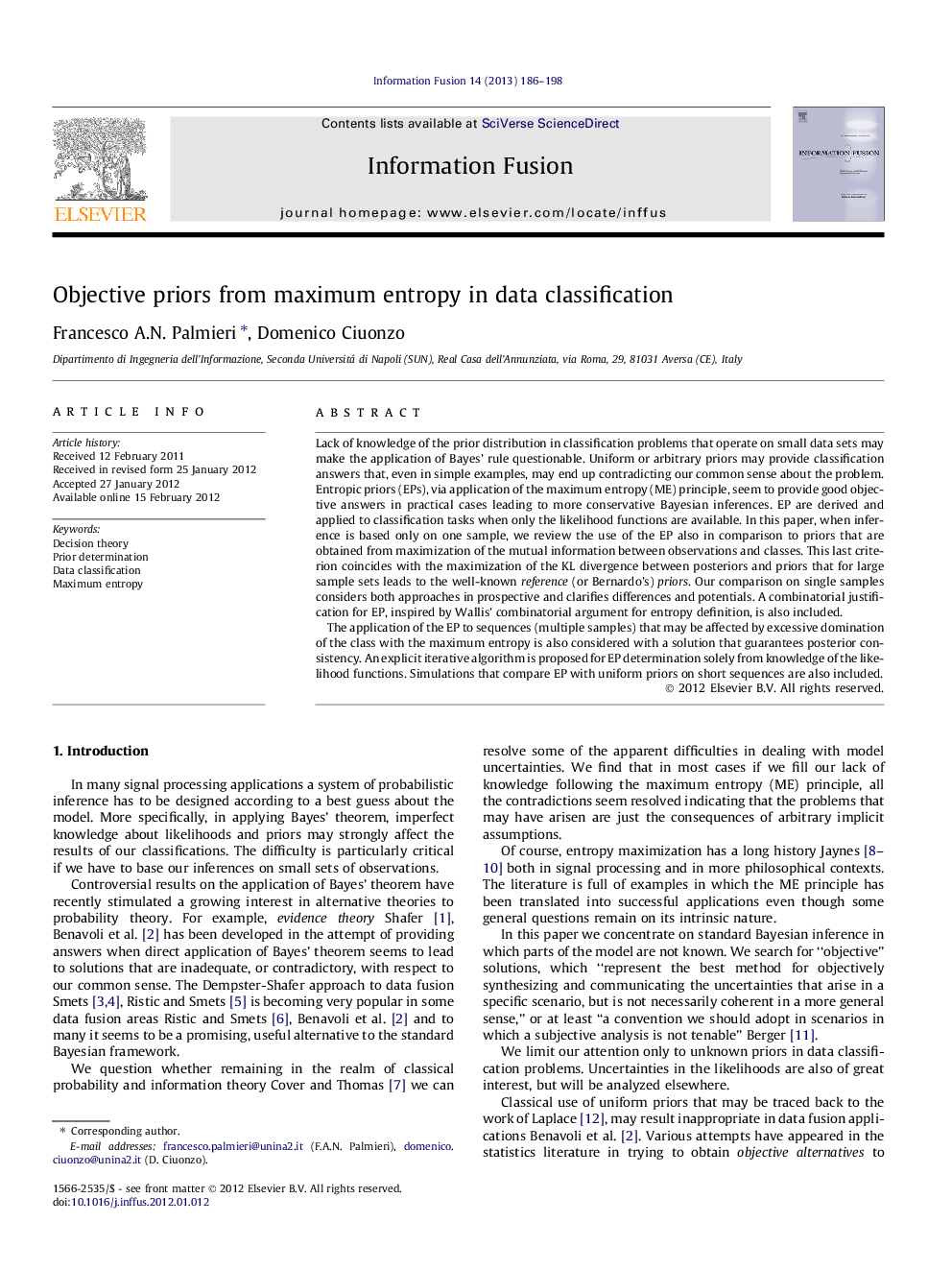
Lack of knowledge of the prior distribution in classification problems that operate on small data sets may make the application of Bayes’ rule questionable. Uniform or arbitrary priors may provide classification answers that, even in simple examples, may end up contradicting our common sense about the problem. Entropic priors (EPs), via application of the maximum entropy (ME) principle, seem to provide good objective answers in practical cases leading to more conservative Bayesian inferences. EP are derived and applied to classification tasks when only the likelihood functions are available. In this paper, when inference is based only on one sample, we review the use of the EP also in comparison to priors that are obtained from maximization of the mutual information between observations and classes. This last criterion coincides with the maximization of the KL divergence between posteriors and priors that for large sample sets leads to the well-known reference (or Bernardo’s) priors. Our comparison on single samples considers both approaches in prospective and clarifies differences and potentials. A combinatorial justification for EP, inspired by Wallis’ combinatorial argument for entropy definition, is also included.The application of the EP to sequences (multiple samples) that may be affected by excessive domination of the class with the maximum entropy is also considered with a solution that guarantees posterior consistency. An explicit iterative algorithm is proposed for EP determination solely from knowledge of the likelihood functions. Simulations that compare EP with uniform priors on short sequences are also included.
Journal: Information Fusion - Volume 14, Issue 2, April 2013, Pages 186–198