کد مقاله | کد نشریه | سال انتشار | مقاله انگلیسی | نسخه تمام متن |
---|---|---|---|---|
528919 | 869617 | 2011 | 10 صفحه PDF | دانلود رایگان |
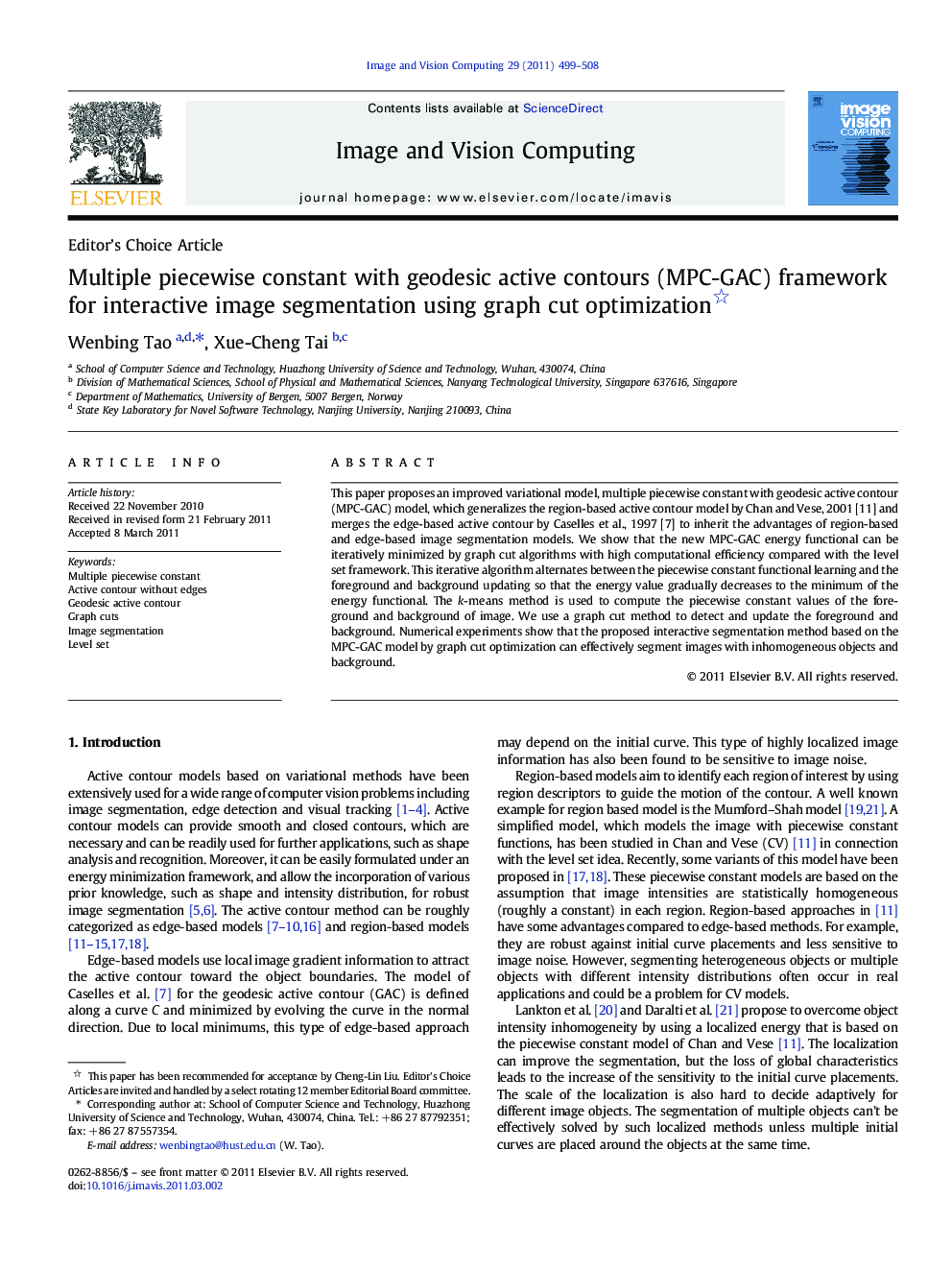
This paper proposes an improved variational model, multiple piecewise constant with geodesic active contour (MPC-GAC) model, which generalizes the region-based active contour model by Chan and Vese, 2001 [11] and merges the edge-based active contour by Caselles et al., 1997 [7] to inherit the advantages of region-based and edge-based image segmentation models. We show that the new MPC-GAC energy functional can be iteratively minimized by graph cut algorithms with high computational efficiency compared with the level set framework. This iterative algorithm alternates between the piecewise constant functional learning and the foreground and background updating so that the energy value gradually decreases to the minimum of the energy functional. The k-means method is used to compute the piecewise constant values of the foreground and background of image. We use a graph cut method to detect and update the foreground and background. Numerical experiments show that the proposed interactive segmentation method based on the MPC-GAC model by graph cut optimization can effectively segment images with inhomogeneous objects and background.
In Fig. (a) and (b) the “elephant” image is used to test the proposed MPC-GAC model. This image contains three elephant objects, the sky and the grassland background. We want to extract the elephants from the background. In Fig. (a) and (b), we test five cases where the clustering numbers are respectively set to n1 = n2 = 1, n1 = n2 = 3, n1 = n2 = 5, n1 = n2 = 7, and n1 = n2 = 9. In each case we compare the results with or without GAC. From the results we see that larger clustering number leads to better results. However, if we do not include GAC, some mis-segmentation has happened: 1) the details of the elephants such as the legs and tails are easily lost; or 2) some background information is labeled as the foreground, for example, the background near the legs of the elephant isn't separated out. The MPC-GAC model with n1 = n2 = 9 can segment the elephants with the most perfect details. The evolution of the curve by MPC-GAC model with n1 = n2 = 9 for the elephant images is shown in Fig. (c).Figure optionsDownload high-quality image (210 K)Download as PowerPoint slideHighlights
► We propose multiple piecewise constant (MPC) model.
► The new MPC-GAC model can be iteratively minimized by graph cut algorithms.
► Some experiment evaluation for CV, MPC, CV-GAC and MPC-GAC model.
Journal: Image and Vision Computing - Volume 29, Issue 8, July 2011, Pages 499–508