کد مقاله | کد نشریه | سال انتشار | مقاله انگلیسی | نسخه تمام متن |
---|---|---|---|---|
532243 | 869926 | 2009 | 13 صفحه PDF | دانلود رایگان |
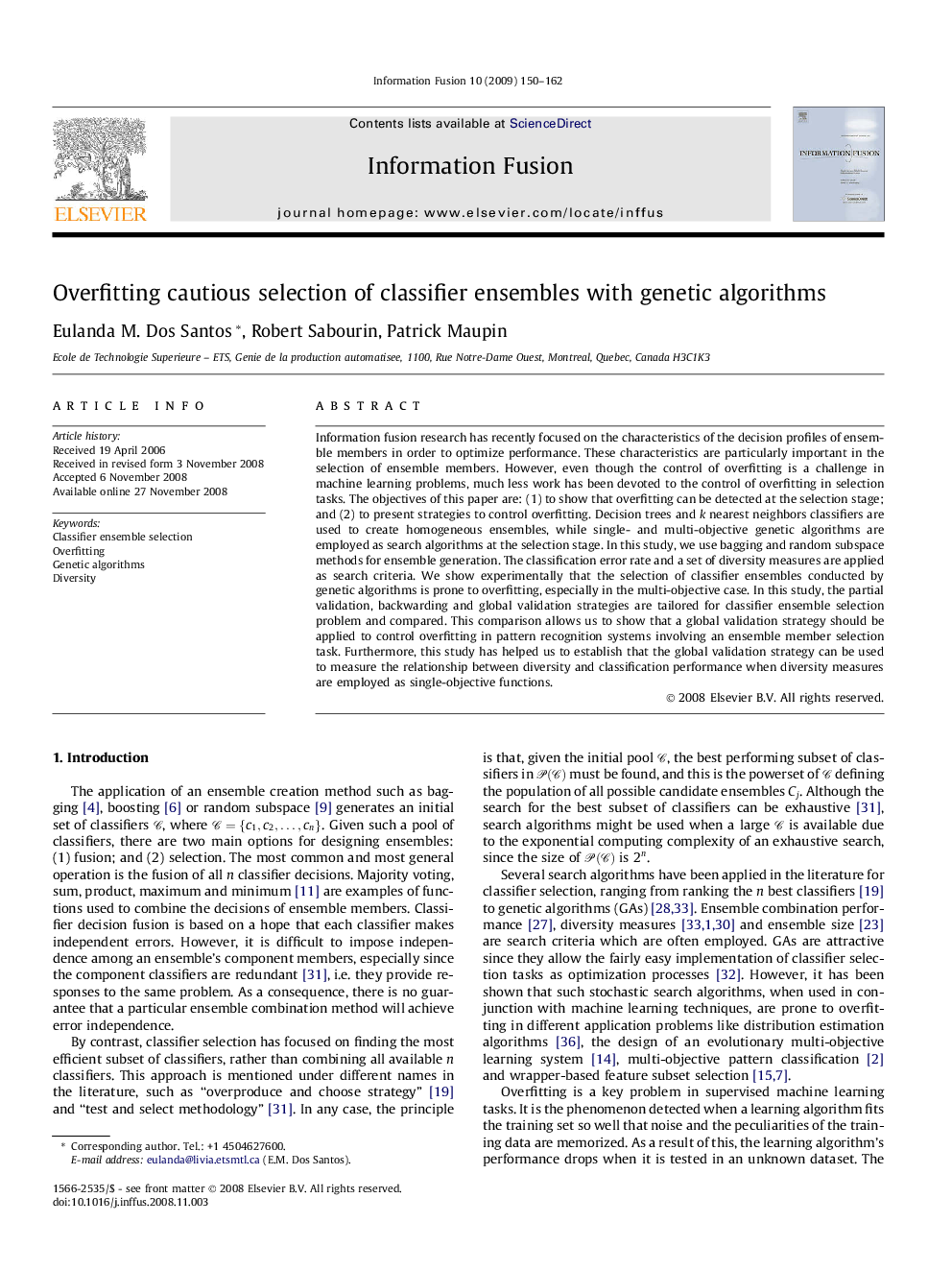
Information fusion research has recently focused on the characteristics of the decision profiles of ensemble members in order to optimize performance. These characteristics are particularly important in the selection of ensemble members. However, even though the control of overfitting is a challenge in machine learning problems, much less work has been devoted to the control of overfitting in selection tasks. The objectives of this paper are: (1) to show that overfitting can be detected at the selection stage; and (2) to present strategies to control overfitting. Decision trees and k nearest neighbors classifiers are used to create homogeneous ensembles, while single- and multi-objective genetic algorithms are employed as search algorithms at the selection stage. In this study, we use bagging and random subspace methods for ensemble generation. The classification error rate and a set of diversity measures are applied as search criteria. We show experimentally that the selection of classifier ensembles conducted by genetic algorithms is prone to overfitting, especially in the multi-objective case. In this study, the partial validation, backwarding and global validation strategies are tailored for classifier ensemble selection problem and compared. This comparison allows us to show that a global validation strategy should be applied to control overfitting in pattern recognition systems involving an ensemble member selection task. Furthermore, this study has helped us to establish that the global validation strategy can be used to measure the relationship between diversity and classification performance when diversity measures are employed as single-objective functions.
Journal: Information Fusion - Volume 10, Issue 2, April 2009, Pages 150–162