کد مقاله | کد نشریه | سال انتشار | مقاله انگلیسی | نسخه تمام متن |
---|---|---|---|---|
536917 | 870646 | 2013 | 17 صفحه PDF | دانلود رایگان |
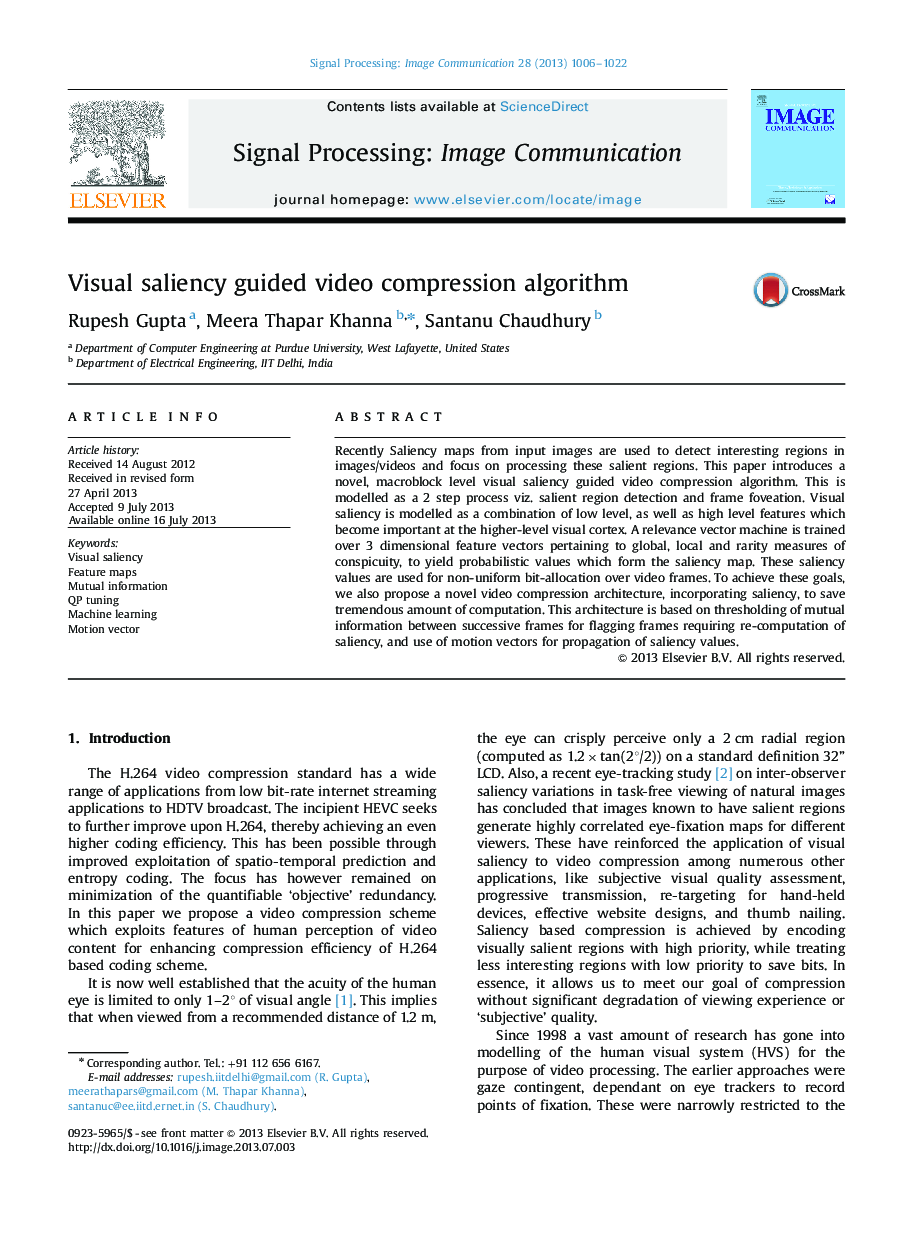
• A two-stage novel video compression architecture is proposed for video compression.
• Machine learning scheme over three dimensional features is used for saliency computation.
• Saliency computation at macroblock level saves computation.
• Thresholding of mutual information between successive frames indicates the frames requiring re-computation of saliency.
• The motion vectors propagate the saliency values for macroblocks in P frames.
Recently Saliency maps from input images are used to detect interesting regions in images/videos and focus on processing these salient regions. This paper introduces a novel, macroblock level visual saliency guided video compression algorithm. This is modelled as a 2 step process viz. salient region detection and frame foveation. Visual saliency is modelled as a combination of low level, as well as high level features which become important at the higher-level visual cortex. A relevance vector machine is trained over 3 dimensional feature vectors pertaining to global, local and rarity measures of conspicuity, to yield probabilistic values which form the saliency map. These saliency values are used for non-uniform bit-allocation over video frames. To achieve these goals, we also propose a novel video compression architecture, incorporating saliency, to save tremendous amount of computation. This architecture is based on thresholding of mutual information between successive frames for flagging frames requiring re-computation of saliency, and use of motion vectors for propagation of saliency values.
Journal: Signal Processing: Image Communication - Volume 28, Issue 9, October 2013, Pages 1006–1022