کد مقاله | کد نشریه | سال انتشار | مقاله انگلیسی | نسخه تمام متن |
---|---|---|---|---|
537398 | 870815 | 2015 | 14 صفحه PDF | دانلود رایگان |
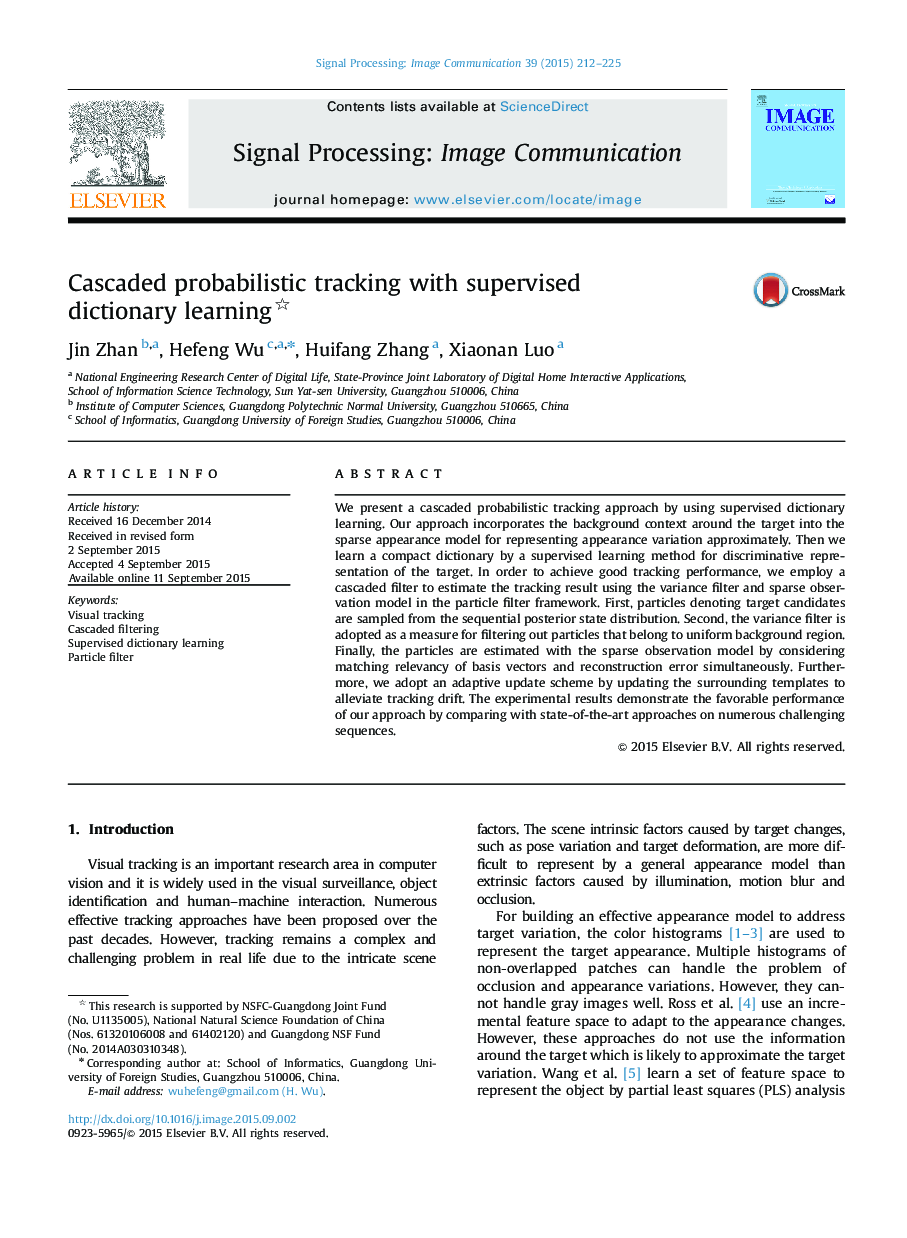
• Use surrounding templates to represent appearance variation approximately.
• Learn a compact and discriminative dictionary by supervised learning method.
• Combine variance filter and observation model in cascaded probabilistic tracking.
• Adopt an adaptive update scheme to update the surrounding templates.
We present a cascaded probabilistic tracking approach by using supervised dictionary learning. Our approach incorporates the background context around the target into the sparse appearance model for representing appearance variation approximately. Then we learn a compact dictionary by a supervised learning method for discriminative representation of the target. In order to achieve good tracking performance, we employ a cascaded filter to estimate the tracking result using the variance filter and sparse observation model in the particle filter framework. First, particles denoting target candidates are sampled from the sequential posterior state distribution. Second, the variance filter is adopted as a measure for filtering out particles that belong to uniform background region. Finally, the particles are estimated with the sparse observation model by considering matching relevancy of basis vectors and reconstruction error simultaneously. Furthermore, we adopt an adaptive update scheme by updating the surrounding templates to alleviate tracking drift. The experimental results demonstrate the favorable performance of our approach by comparing with state-of-the-art approaches on numerous challenging sequences.
Journal: Signal Processing: Image Communication - Volume 39, Part A, November 2015, Pages 212–225