کد مقاله | کد نشریه | سال انتشار | مقاله انگلیسی | نسخه تمام متن |
---|---|---|---|---|
558731 | 1451740 | 2015 | 12 صفحه PDF | دانلود رایگان |
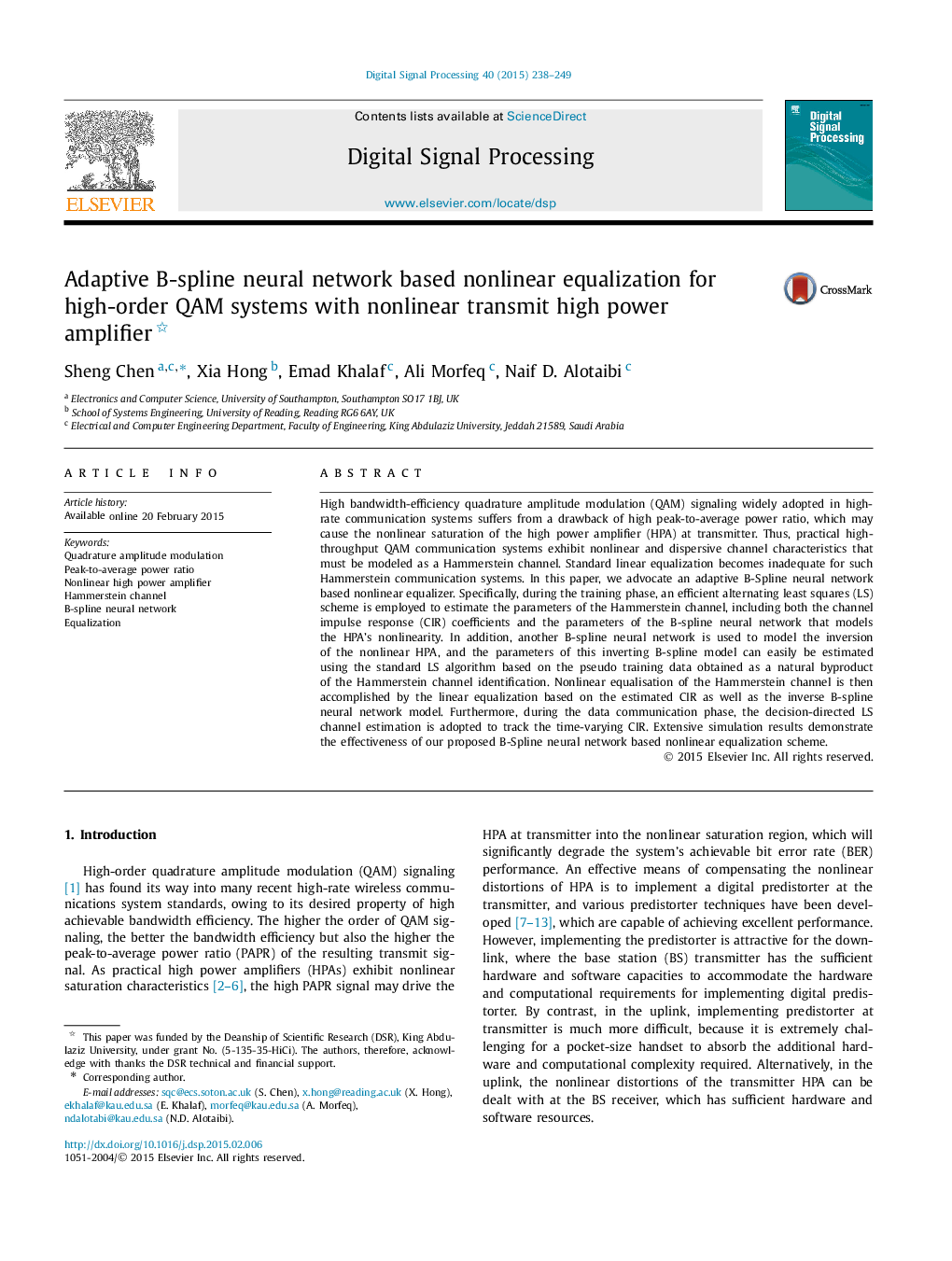
• Complex-valued B-spline nonlinear equalizer for Hammerstain channels.
• Alternative least squares algorithm for Hammerstain channel modeling.
• Pseudo training data based least squares algorithm for B-spline inverting model.
• Decision-directed adaptation to track the time-varying channel.
High bandwidth-efficiency quadrature amplitude modulation (QAM) signaling widely adopted in high-rate communication systems suffers from a drawback of high peak-to-average power ratio, which may cause the nonlinear saturation of the high power amplifier (HPA) at transmitter. Thus, practical high-throughput QAM communication systems exhibit nonlinear and dispersive channel characteristics that must be modeled as a Hammerstein channel. Standard linear equalization becomes inadequate for such Hammerstein communication systems. In this paper, we advocate an adaptive B-Spline neural network based nonlinear equalizer. Specifically, during the training phase, an efficient alternating least squares (LS) scheme is employed to estimate the parameters of the Hammerstein channel, including both the channel impulse response (CIR) coefficients and the parameters of the B-spline neural network that models the HPA's nonlinearity. In addition, another B-spline neural network is used to model the inversion of the nonlinear HPA, and the parameters of this inverting B-spline model can easily be estimated using the standard LS algorithm based on the pseudo training data obtained as a natural byproduct of the Hammerstein channel identification. Nonlinear equalisation of the Hammerstein channel is then accomplished by the linear equalization based on the estimated CIR as well as the inverse B-spline neural network model. Furthermore, during the data communication phase, the decision-directed LS channel estimation is adopted to track the time-varying CIR. Extensive simulation results demonstrate the effectiveness of our proposed B-Spline neural network based nonlinear equalization scheme.
Journal: Digital Signal Processing - Volume 40, May 2015, Pages 238–249