کد مقاله | کد نشریه | سال انتشار | مقاله انگلیسی | نسخه تمام متن |
---|---|---|---|---|
5722132 | 1608117 | 2017 | 7 صفحه PDF | دانلود رایگان |
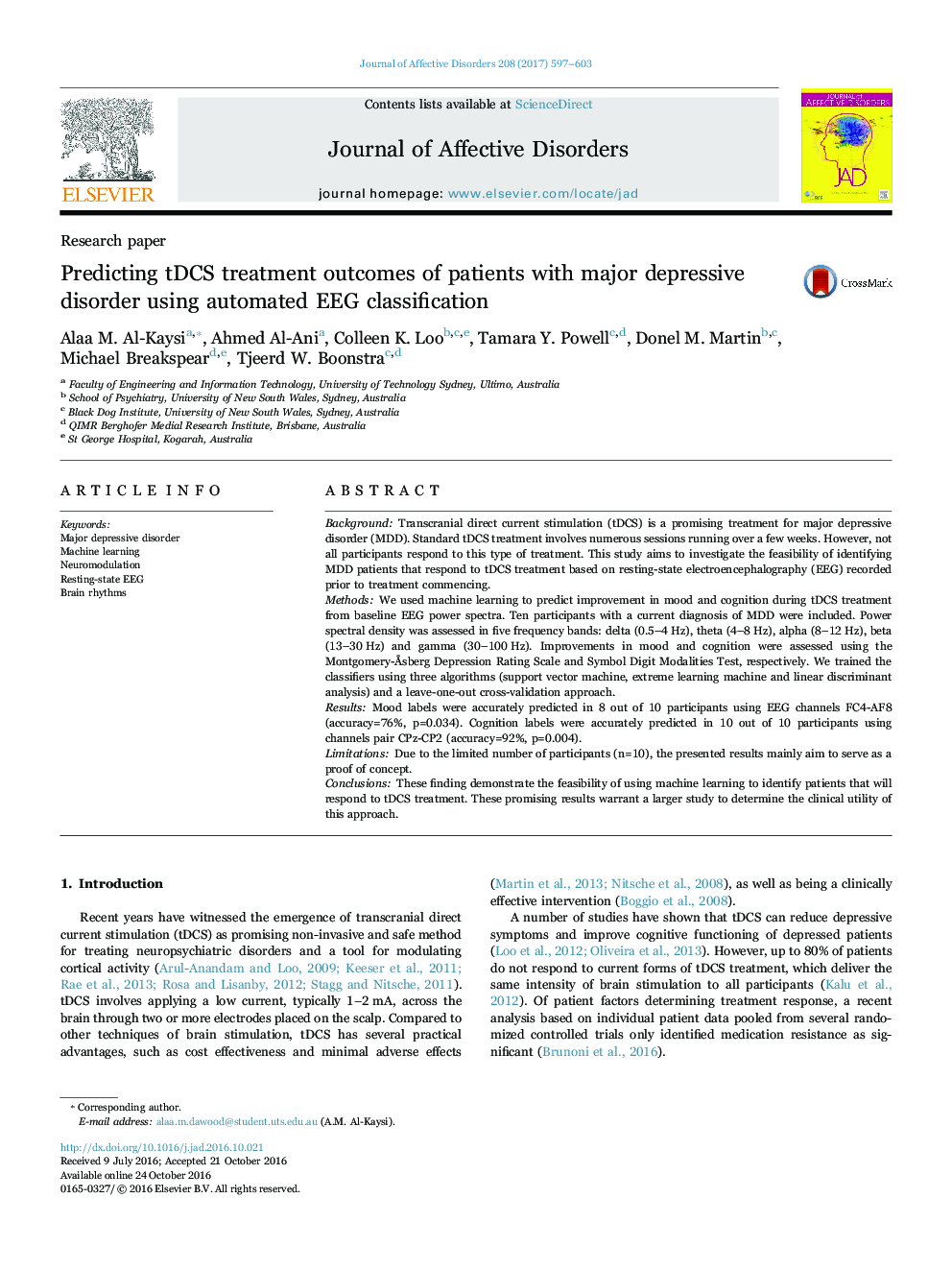
- We used machine learning to predict tDCS treatment outcomes in patients with MDD.
- Resting-state EEG was recorded before the start of tDCS treatment.
- Improvement in mood and cognition was assessed following tDCS treatment.
- Mood was accurately predicted in 8 of 10 and cognition labels in 10 out of 10 participants.
- These results indicate the predictive value of resting-state EEG recorded at baseline.
BackgroundTranscranial direct current stimulation (tDCS) is a promising treatment for major depressive disorder (MDD). Standard tDCS treatment involves numerous sessions running over a few weeks. However, not all participants respond to this type of treatment. This study aims to investigate the feasibility of identifying MDD patients that respond to tDCS treatment based on resting-state electroencephalography (EEG) recorded prior to treatment commencing.MethodsWe used machine learning to predict improvement in mood and cognition during tDCS treatment from baseline EEG power spectra. Ten participants with a current diagnosis of MDD were included. Power spectral density was assessed in five frequency bands: delta (0.5-4Â Hz), theta (4-8Â Hz), alpha (8-12Â Hz), beta (13-30Â Hz) and gamma (30-100Â Hz). Improvements in mood and cognition were assessed using the Montgomery-Ã sberg Depression Rating Scale and Symbol Digit Modalities Test, respectively. We trained the classifiers using three algorithms (support vector machine, extreme learning machine and linear discriminant analysis) and a leave-one-out cross-validation approach.ResultsMood labels were accurately predicted in 8 out of 10 participants using EEG channels FC4-AF8 (accuracy=76%, p=0.034). Cognition labels were accurately predicted in 10 out of 10 participants using channels pair CPz-CP2 (accuracy=92%, p=0.004).LimitationsDue to the limited number of participants (n=10), the presented results mainly aim to serve as a proof of concept.ConclusionsThese finding demonstrate the feasibility of using machine learning to identify patients that will respond to tDCS treatment. These promising results warrant a larger study to determine the clinical utility of this approach.
Journal: Journal of Affective Disorders - Volume 208, 15 January 2017, Pages 597-603