کد مقاله | کد نشریه | سال انتشار | مقاله انگلیسی | نسخه تمام متن |
---|---|---|---|---|
6268923 | 1614647 | 2014 | 8 صفحه PDF | دانلود رایگان |
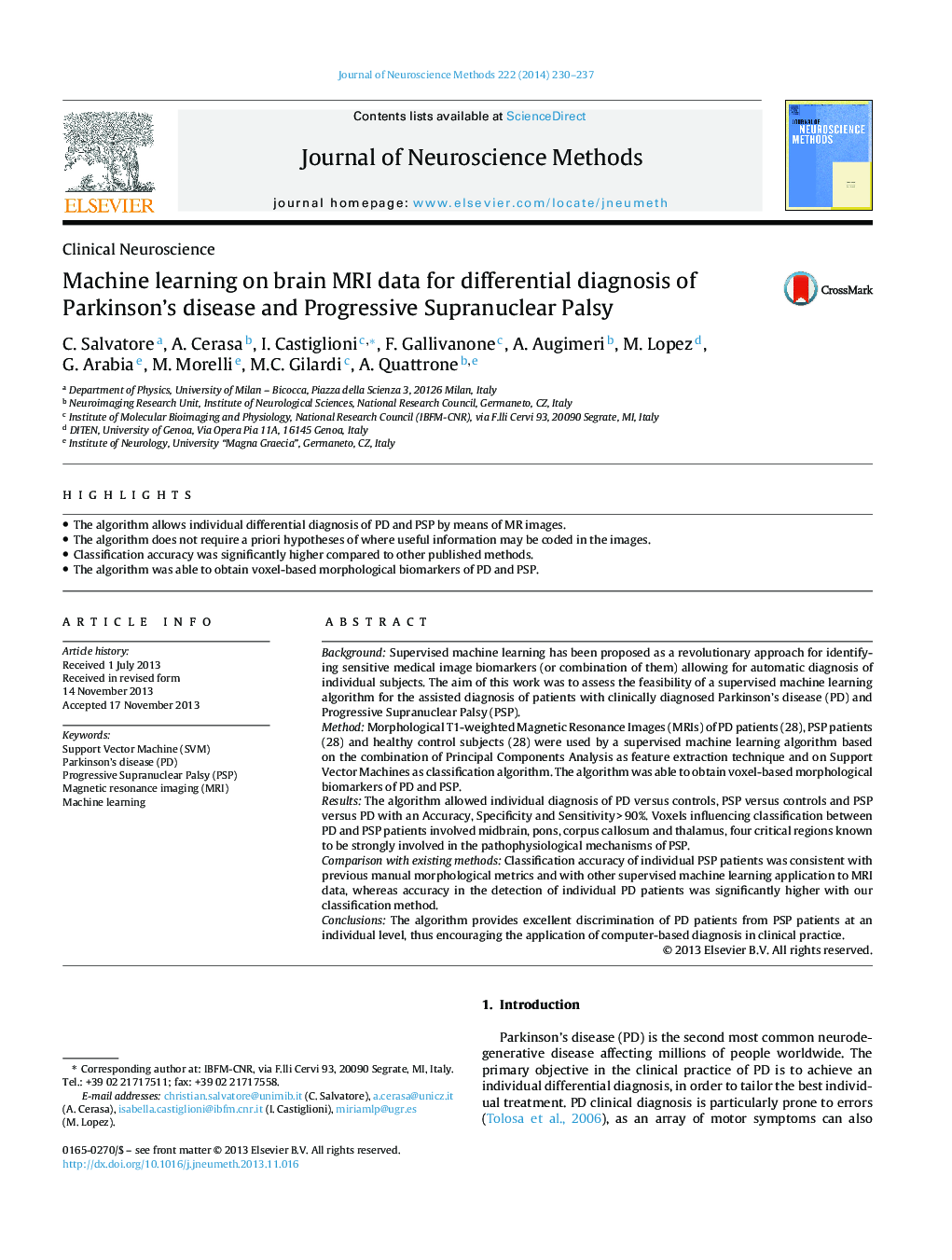
- The algorithm allows individual differential diagnosis of PD and PSP by means of MR images.
- The algorithm does not require a priori hypotheses of where useful information may be coded in the images.
- Classification accuracy was significantly higher compared to other published methods.
- The algorithm was able to obtain voxel-based morphological biomarkers of PD and PSP.
BackgroundSupervised machine learning has been proposed as a revolutionary approach for identifying sensitive medical image biomarkers (or combination of them) allowing for automatic diagnosis of individual subjects. The aim of this work was to assess the feasibility of a supervised machine learning algorithm for the assisted diagnosis of patients with clinically diagnosed Parkinson's disease (PD) and Progressive Supranuclear Palsy (PSP).MethodMorphological T1-weighted Magnetic Resonance Images (MRIs) of PD patients (28), PSP patients (28) and healthy control subjects (28) were used by a supervised machine learning algorithm based on the combination of Principal Components Analysis as feature extraction technique and on Support Vector Machines as classification algorithm. The algorithm was able to obtain voxel-based morphological biomarkers of PD and PSP.ResultsThe algorithm allowed individual diagnosis of PD versus controls, PSP versus controls and PSP versus PD with an Accuracy, Specificity and Sensitivity > 90%. Voxels influencing classification between PD and PSP patients involved midbrain, pons, corpus callosum and thalamus, four critical regions known to be strongly involved in the pathophysiological mechanisms of PSP.Comparison with existing methodsClassification accuracy of individual PSP patients was consistent with previous manual morphological metrics and with other supervised machine learning application to MRI data, whereas accuracy in the detection of individual PD patients was significantly higher with our classification method.ConclusionsThe algorithm provides excellent discrimination of PD patients from PSP patients at an individual level, thus encouraging the application of computer-based diagnosis in clinical practice.
Journal: Journal of Neuroscience Methods - Volume 222, 30 January 2014, Pages 230-237