کد مقاله | کد نشریه | سال انتشار | مقاله انگلیسی | نسخه تمام متن |
---|---|---|---|---|
6397150 | 1330674 | 2013 | 8 صفحه PDF | دانلود رایگان |
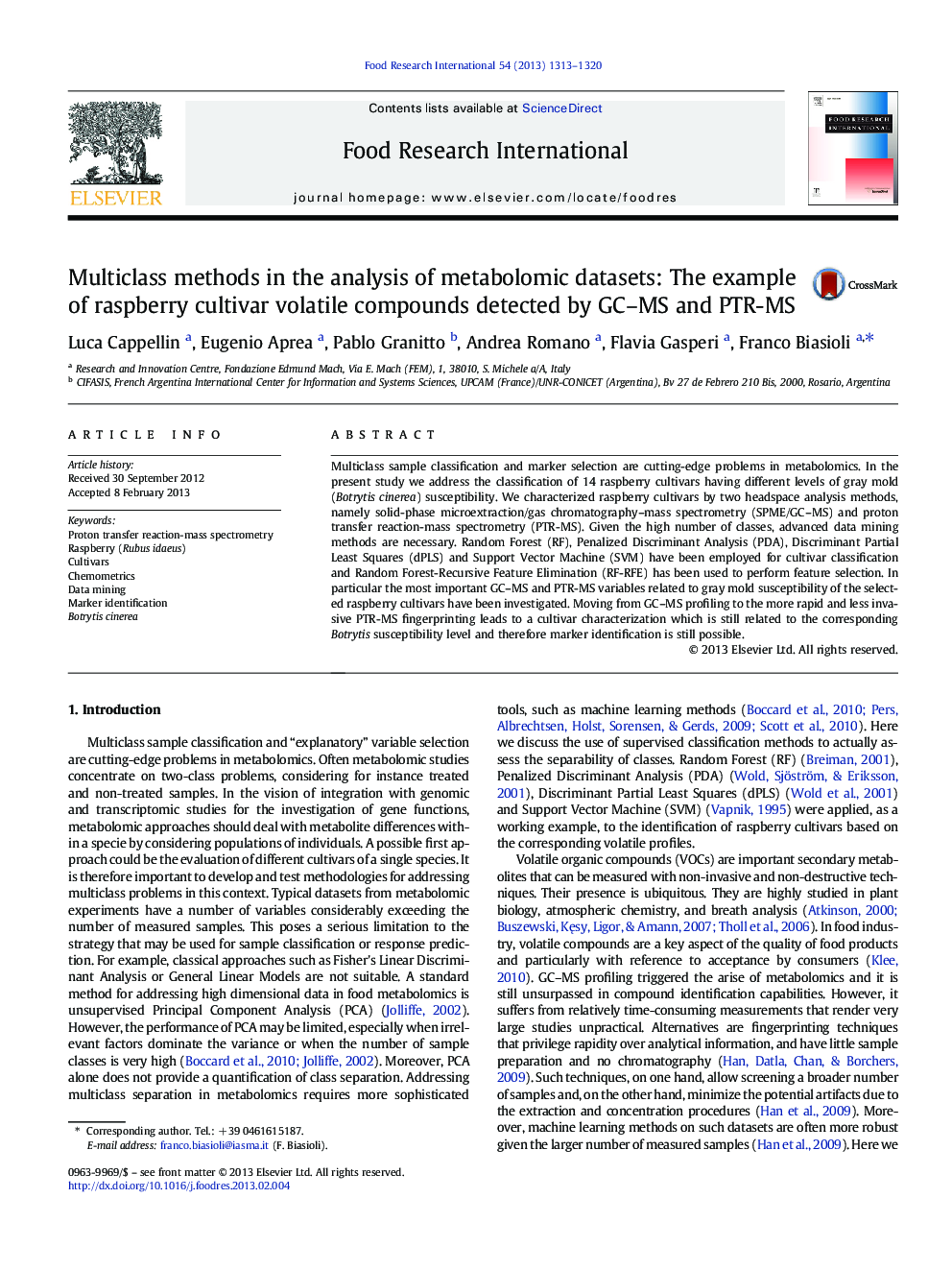
Multiclass sample classification and marker selection are cutting-edge problems in metabolomics. In the present study we address the classification of 14 raspberry cultivars having different levels of gray mold (Botrytis cinerea) susceptibility. We characterized raspberry cultivars by two headspace analysis methods, namely solid-phase microextraction/gas chromatography-mass spectrometry (SPME/GC-MS) and proton transfer reaction-mass spectrometry (PTR-MS). Given the high number of classes, advanced data mining methods are necessary. Random Forest (RF), Penalized Discriminant Analysis (PDA), Discriminant Partial Least Squares (dPLS) and Support Vector Machine (SVM) have been employed for cultivar classification and Random Forest-Recursive Feature Elimination (RF-RFE) has been used to perform feature selection. In particular the most important GC-MS and PTR-MS variables related to gray mold susceptibility of the selected raspberry cultivars have been investigated. Moving from GC-MS profiling to the more rapid and less invasive PTR-MS fingerprinting leads to a cultivar characterization which is still related to the corresponding Botrytis susceptibility level and therefore marker identification is still possible.
⺠The headspace of 14 raspberry cultivars was analyzed. ⺠Efficient handling of multiclass problems is presented. ⺠Good sample classification achieved with advanced data mining methods ⺠Successful marker selection for both SPME/GC-MS and PTR-MS datasets ⺠The level of Botrytis cinerea susceptibility can be predicted from VOC profile.
Journal: Food Research International - Volume 54, Issue 1, November 2013, Pages 1313-1320