کد مقاله | کد نشریه | سال انتشار | مقاله انگلیسی | نسخه تمام متن |
---|---|---|---|---|
6416401 | 1631140 | 2014 | 25 صفحه PDF | دانلود رایگان |
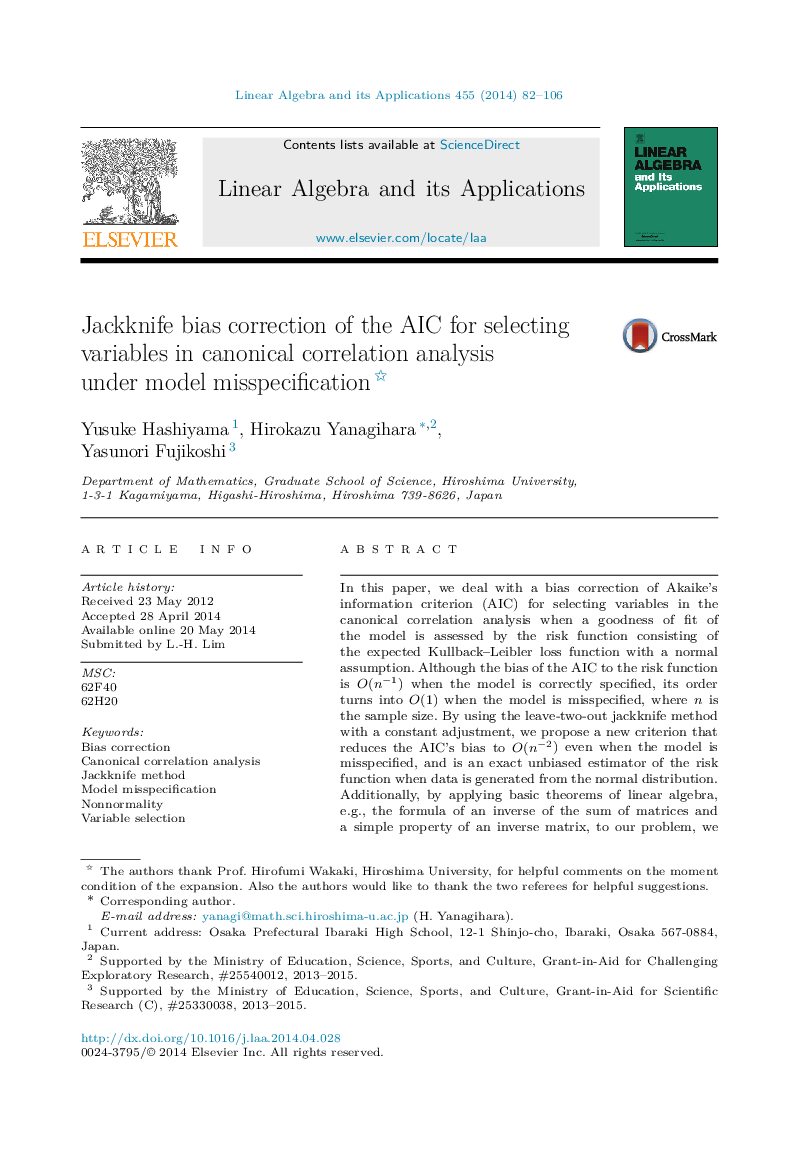
In this paper, we deal with a bias correction of Akaike's information criterion (AIC) for selecting variables in the canonical correlation analysis when a goodness of fit of the model is assessed by the risk function consisting of the expected Kullback-Leibler loss function with a normal assumption. Although the bias of the AIC to the risk function is O(nâ1) when the model is correctly specified, its order turns into O(1) when the model is misspecified, where n is the sample size. By using the leave-two-out jackknife method with a constant adjustment, we propose a new criterion that reduces the AIC's bias to O(nâ2) even when the model is misspecified, and is an exact unbiased estimator of the risk function when data is generated from the normal distribution. Additionally, by applying basic theorems of linear algebra, e.g., the formula of an inverse of the sum of matrices and a simple property of an inverse matrix, to our problem, we obtain strict conditions to guarantee the validity of the bias correction, and another expression of the proposed criterion to reduce computational time tremendously, which does not contain any jackknife estimators.
Journal: Linear Algebra and its Applications - Volume 455, 15 August 2014, Pages 82-106