کد مقاله | کد نشریه | سال انتشار | مقاله انگلیسی | نسخه تمام متن |
---|---|---|---|---|
6540683 | 158863 | 2015 | 11 صفحه PDF | دانلود رایگان |
عنوان انگلیسی مقاله ISI
Hyperspectral imaging combined with multivariate analysis and band math for detection of common defects on peaches (Prunus persica)
دانلود مقاله + سفارش ترجمه
دانلود مقاله ISI انگلیسی
رایگان برای ایرانیان
کلمات کلیدی
موضوعات مرتبط
مهندسی و علوم پایه
مهندسی کامپیوتر
نرم افزارهای علوم کامپیوتر
پیش نمایش صفحه اول مقاله
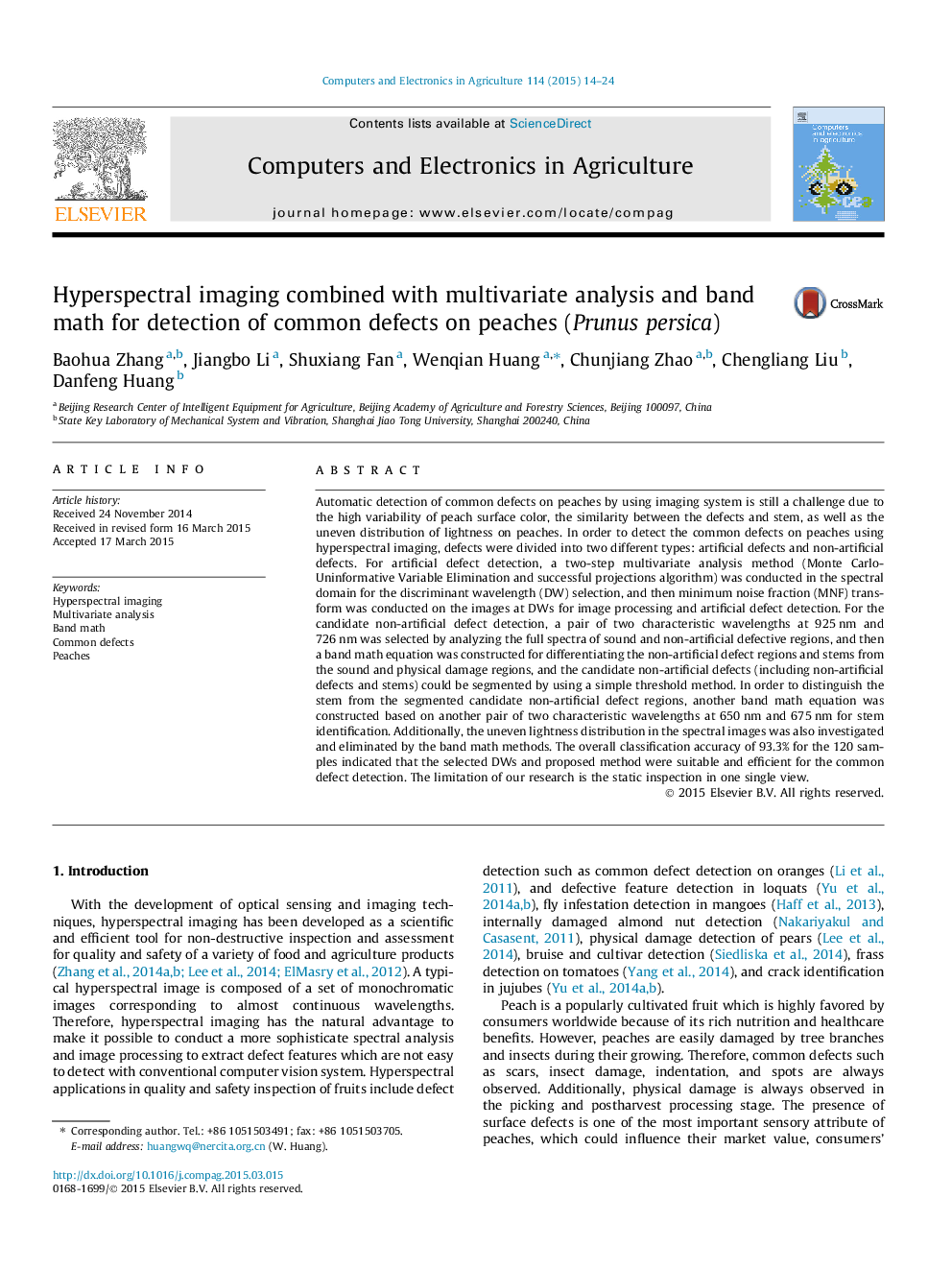
چکیده انگلیسی
Automatic detection of common defects on peaches by using imaging system is still a challenge due to the high variability of peach surface color, the similarity between the defects and stem, as well as the uneven distribution of lightness on peaches. In order to detect the common defects on peaches using hyperspectral imaging, defects were divided into two different types: artificial defects and non-artificial defects. For artificial defect detection, a two-step multivariate analysis method (Monte Carlo-Uninformative Variable Elimination and successful projections algorithm) was conducted in the spectral domain for the discriminant wavelength (DW) selection, and then minimum noise fraction (MNF) transform was conducted on the images at DWs for image processing and artificial defect detection. For the candidate non-artificial defect detection, a pair of two characteristic wavelengths at 925Â nm and 726Â nm was selected by analyzing the full spectra of sound and non-artificial defective regions, and then a band math equation was constructed for differentiating the non-artificial defect regions and stems from the sound and physical damage regions, and the candidate non-artificial defects (including non-artificial defects and stems) could be segmented by using a simple threshold method. In order to distinguish the stem from the segmented candidate non-artificial defect regions, another band math equation was constructed based on another pair of two characteristic wavelengths at 650Â nm and 675Â nm for stem identification. Additionally, the uneven lightness distribution in the spectral images was also investigated and eliminated by the band math methods. The overall classification accuracy of 93.3% for the 120 samples indicated that the selected DWs and proposed method were suitable and efficient for the common defect detection. The limitation of our research is the static inspection in one single view.
ناشر
Database: Elsevier - ScienceDirect (ساینس دایرکت)
Journal: Computers and Electronics in Agriculture - Volume 114, June 2015, Pages 14-24
Journal: Computers and Electronics in Agriculture - Volume 114, June 2015, Pages 14-24
نویسندگان
Baohua Zhang, Jiangbo Li, Shuxiang Fan, Wenqian Huang, Chunjiang Zhao, Chengliang Liu, Danfeng Huang,