کد مقاله | کد نشریه | سال انتشار | مقاله انگلیسی | نسخه تمام متن |
---|---|---|---|---|
6863503 | 678093 | 2012 | 16 صفحه PDF | دانلود رایگان |
عنوان انگلیسی مقاله ISI
A bio-inspired kinematic controller for obstacle avoidance during reaching tasks with real robots
دانلود مقاله + سفارش ترجمه
دانلود مقاله ISI انگلیسی
رایگان برای ایرانیان
کلمات کلیدی
موضوعات مرتبط
مهندسی و علوم پایه
مهندسی کامپیوتر
هوش مصنوعی
پیش نمایش صفحه اول مقاله
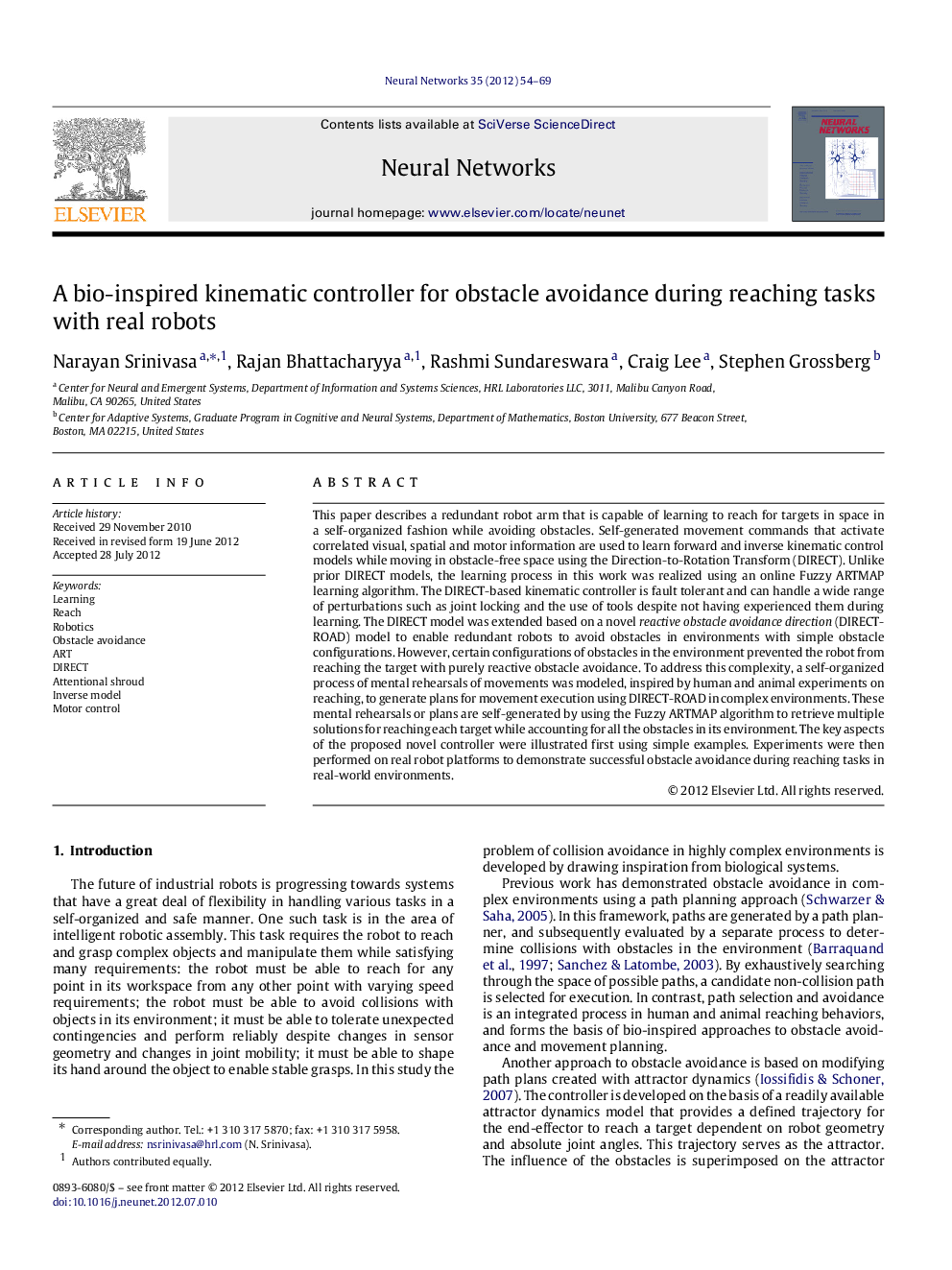
چکیده انگلیسی
This paper describes a redundant robot arm that is capable of learning to reach for targets in space in a self-organized fashion while avoiding obstacles. Self-generated movement commands that activate correlated visual, spatial and motor information are used to learn forward and inverse kinematic control models while moving in obstacle-free space using the Direction-to-Rotation Transform (DIRECT). Unlike prior DIRECT models, the learning process in this work was realized using an online Fuzzy ARTMAP learning algorithm. The DIRECT-based kinematic controller is fault tolerant and can handle a wide range of perturbations such as joint locking and the use of tools despite not having experienced them during learning. The DIRECT model was extended based on a novel reactive obstacle avoidance direction (DIRECT-ROAD) model to enable redundant robots to avoid obstacles in environments with simple obstacle configurations. However, certain configurations of obstacles in the environment prevented the robot from reaching the target with purely reactive obstacle avoidance. To address this complexity, a self-organized process of mental rehearsals of movements was modeled, inspired by human and animal experiments on reaching, to generate plans for movement execution using DIRECT-ROAD in complex environments. These mental rehearsals or plans are self-generated by using the Fuzzy ARTMAP algorithm to retrieve multiple solutions for reaching each target while accounting for all the obstacles in its environment. The key aspects of the proposed novel controller were illustrated first using simple examples. Experiments were then performed on real robot platforms to demonstrate successful obstacle avoidance during reaching tasks in real-world environments.
ناشر
Database: Elsevier - ScienceDirect (ساینس دایرکت)
Journal: Neural Networks - Volume 35, November 2012, Pages 54-69
Journal: Neural Networks - Volume 35, November 2012, Pages 54-69
نویسندگان
Narayan Srinivasa, Rajan Bhattacharyya, Rashmi Sundareswara, Craig Lee, Stephen Grossberg,