کد مقاله | کد نشریه | سال انتشار | مقاله انگلیسی | نسخه تمام متن |
---|---|---|---|---|
6864834 | 1439552 | 2018 | 33 صفحه PDF | دانلود رایگان |
عنوان انگلیسی مقاله ISI
Adaptive neural control for MIMO stochastic nonlinear pure-feedback systems with input saturation and full-state constraints
دانلود مقاله + سفارش ترجمه
دانلود مقاله ISI انگلیسی
رایگان برای ایرانیان
کلمات کلیدی
موضوعات مرتبط
مهندسی و علوم پایه
مهندسی کامپیوتر
هوش مصنوعی
پیش نمایش صفحه اول مقاله
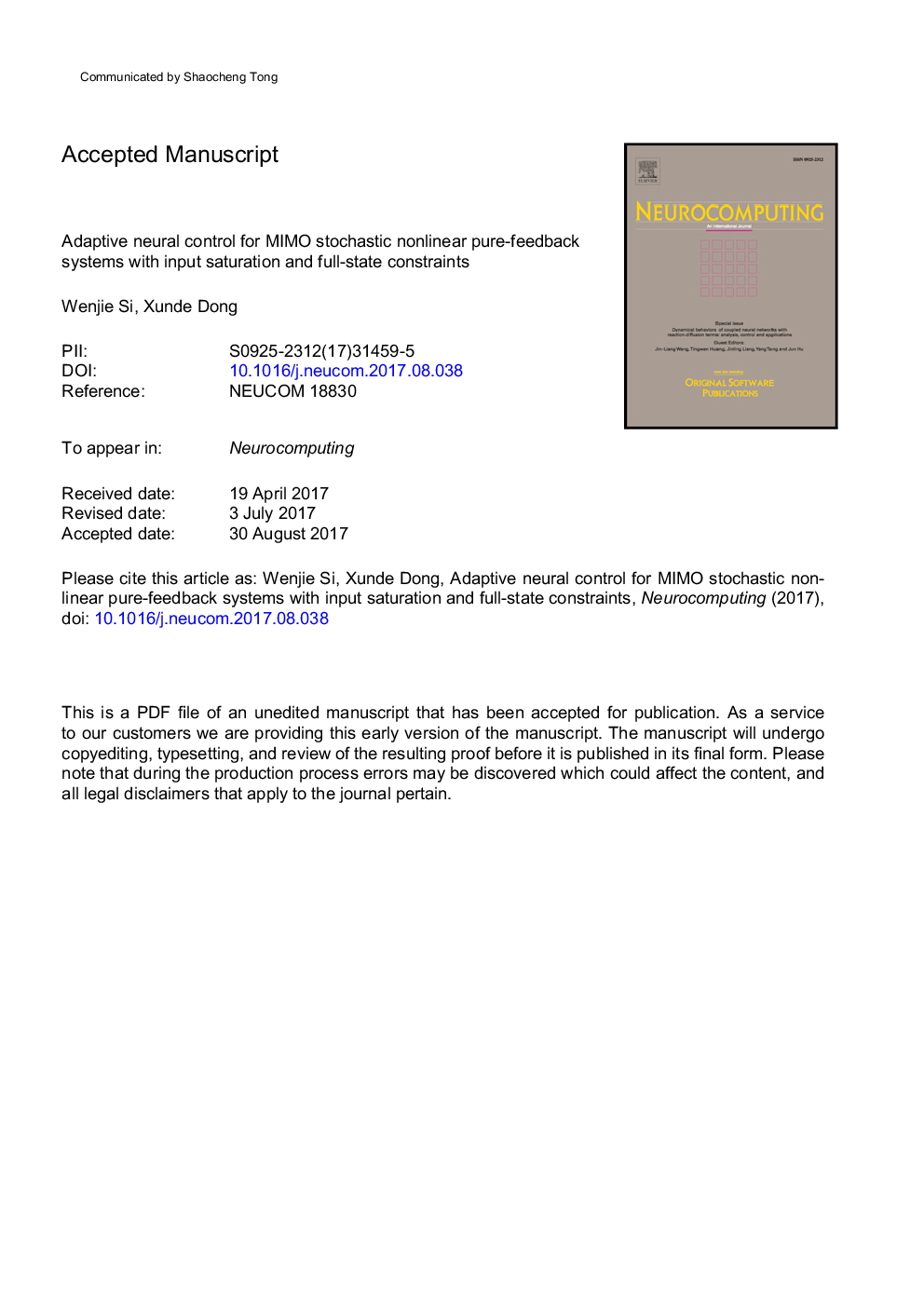
چکیده انگلیسی
This paper investigates an adaptive neural tracking control problem for a class of multi-input multi-output (MIMO) stochastic nonlinear pure-feedback systems with input saturation and full-state constraints. First, the implicit function and mean value theorems are used to overcome the difficulty in the control of pure-feedback systems. Second, the Gaussian error function is employed to represent a continuous differentiable asymmetric saturation model, the neural network is employed to approximate the unknown nonlinearity, and a barrier Lyapunov function is designed to ensure that the full-state variables are restricted. At last, based on Lyapunov stability theory, a robust adaptive neural control method is obtained, which decreases the number of learning parameters and thus reduces the computational burden. It is shown that the designed controller can guarantee that all the signals in the closed-loop system are 4-Moment (or 2 Moment) semi-globally uniformly ultimately bounded (SGUUB) and the tracking error converges to a small neighborhood of the origin. The simulation example is given to further illustrate the effectiveness of the proposed approach.
ناشر
Database: Elsevier - ScienceDirect (ساینس دایرکت)
Journal: Neurocomputing - Volume 275, 31 January 2018, Pages 298-307
Journal: Neurocomputing - Volume 275, 31 January 2018, Pages 298-307
نویسندگان
Wenjie Si, Xunde Dong,