کد مقاله | کد نشریه | سال انتشار | مقاله انگلیسی | نسخه تمام متن |
---|---|---|---|---|
6866653 | 678246 | 2014 | 9 صفحه PDF | دانلود رایگان |
عنوان انگلیسی مقاله ISI
Convergence of online gradient method for feedforward neural networks with smoothing L1/2 regularization penalty
دانلود مقاله + سفارش ترجمه
دانلود مقاله ISI انگلیسی
رایگان برای ایرانیان
کلمات کلیدی
موضوعات مرتبط
مهندسی و علوم پایه
مهندسی کامپیوتر
هوش مصنوعی
پیش نمایش صفحه اول مقاله
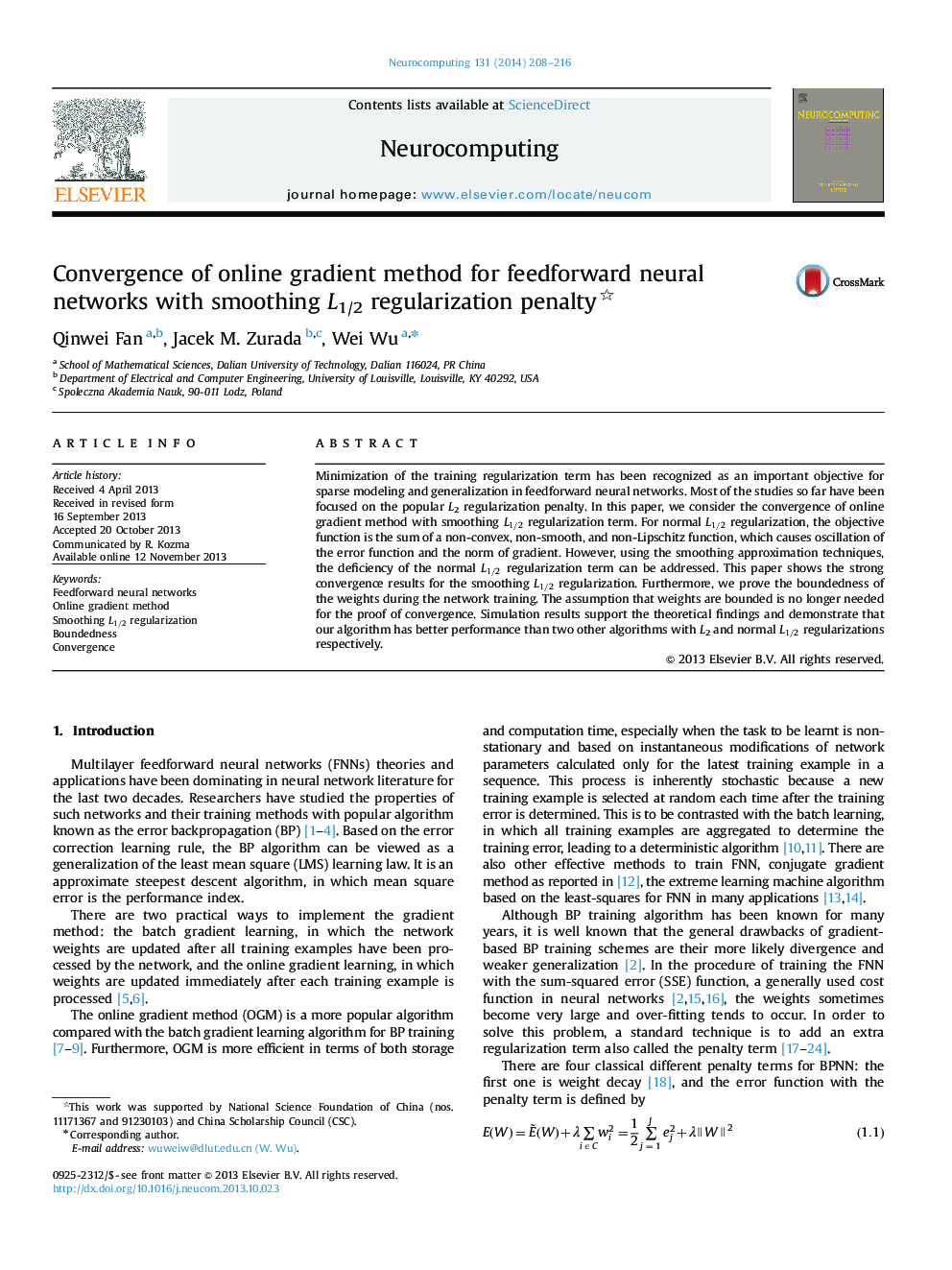
چکیده انگلیسی
Minimization of the training regularization term has been recognized as an important objective for sparse modeling and generalization in feedforward neural networks. Most of the studies so far have been focused on the popular L2 regularization penalty. In this paper, we consider the convergence of online gradient method with smoothing L1/2 regularization term. For normal L1/2 regularization, the objective function is the sum of a non-convex, non-smooth, and non-Lipschitz function, which causes oscillation of the error function and the norm of gradient. However, using the smoothing approximation techniques, the deficiency of the normal L1/2 regularization term can be addressed. This paper shows the strong convergence results for the smoothing L1/2 regularization. Furthermore, we prove the boundedness of the weights during the network training. The assumption that weights are bounded is no longer needed for the proof of convergence. Simulation results support the theoretical findings and demonstrate that our algorithm has better performance than two other algorithms with L2 and normal L1/2 regularizations respectively.
ناشر
Database: Elsevier - ScienceDirect (ساینس دایرکت)
Journal: Neurocomputing - Volume 131, 5 May 2014, Pages 208-216
Journal: Neurocomputing - Volume 131, 5 May 2014, Pages 208-216
نویسندگان
Qinwei Fan, Jacek M. Zurada, Wei Wu,