کد مقاله | کد نشریه | سال انتشار | مقاله انگلیسی | نسخه تمام متن |
---|---|---|---|---|
6884859 | 1444356 | 2018 | 22 صفحه PDF | دانلود رایگان |
عنوان انگلیسی مقاله ISI
Device-free human localization and tracking with UHF passive RFID tags: A data-driven approach
دانلود مقاله + سفارش ترجمه
دانلود مقاله ISI انگلیسی
رایگان برای ایرانیان
کلمات کلیدی
موضوعات مرتبط
مهندسی و علوم پایه
مهندسی کامپیوتر
شبکه های کامپیوتری و ارتباطات
پیش نمایش صفحه اول مقاله
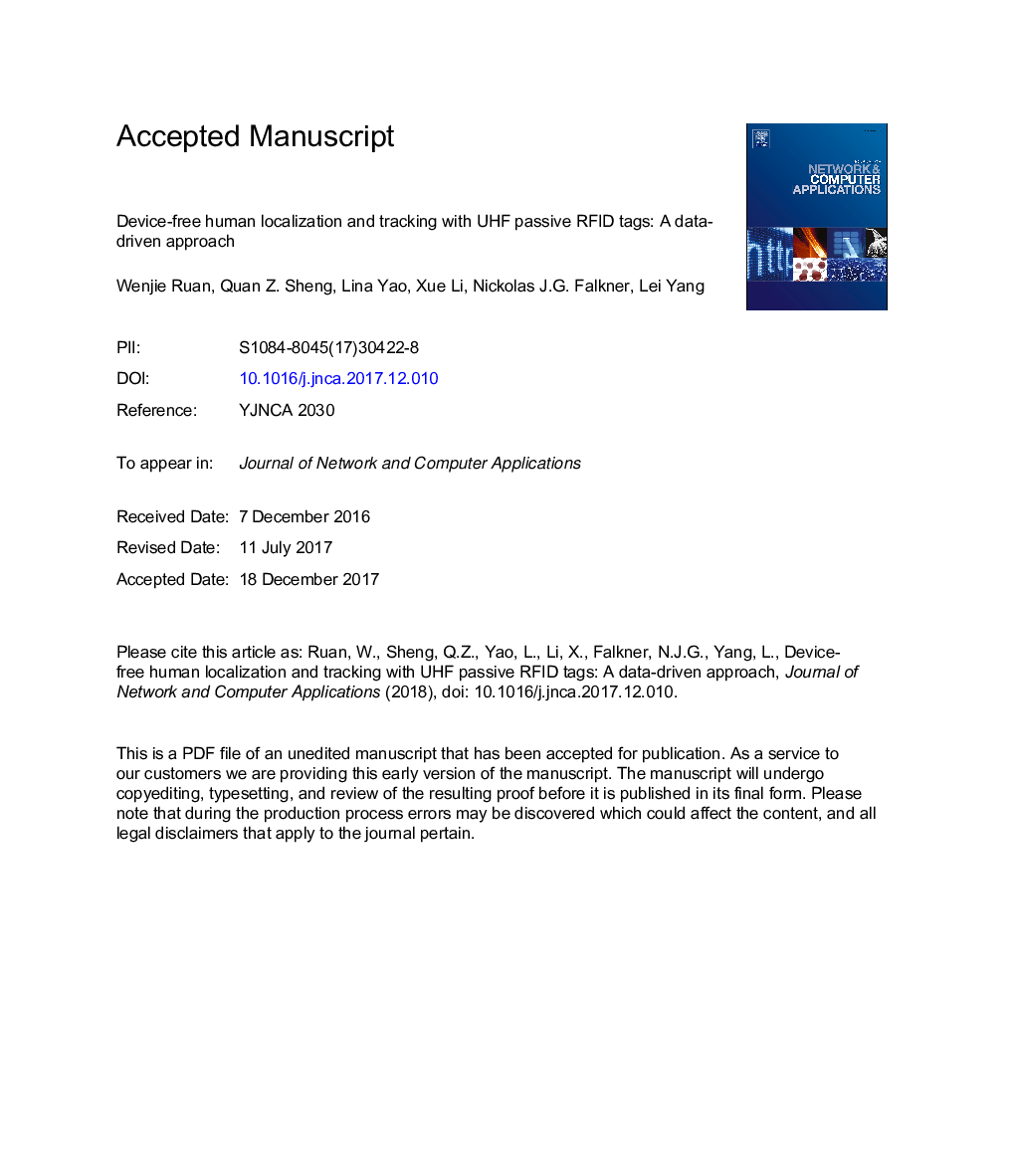
چکیده انگلیسی
Localizing and tracking human movement in a device-free and passive manner is promising in two aspects: i) it neither requires users to wear any sensors or devices, ii) nor it needs them to consciously cooperate during the localization. Such indoor localization technique underpins many real-world applications such as shopping navigation, intruder detection, surveillance care of seniors etc. However, current passive localization techniques either need expensive/sophisticated hardware such as ultra-wideband radar or infrared sensors, or have an issue of invasion of privacy such as camera-based techniques, or need regular maintenance such as the replacement of batteries. In this paper, we build a novel data-driven localization and tracking system upon a set of commercial ultra-high frequency passive radio-frequency identification tags in an indoor environment. Specifically, we formulate human localization problem as finding a location with the maximum posterior probability given the observed received signal strength indicator from passive radio-frequency identification tags. In this regard, we design a series of localization schemes to capture the posterior probability by taking the advance of supervised-learning models including Gaussian Mixture Model, k Nearest Neighbor and Kernel-based Learning. For tracking a moving target, we mathematically model the task as searching a location sequence with the most likelihood, in which we first augment the probabilistic estimation learned in localization to construct the Emission Matrix and propose two human mobility models to approximate the Transmission Matrix in the Hidden Markov Model. The proposed tracking model is able to transfer the pattern learned in localization into tracking but also reduce the location-state candidates at each transmission iteration, which increases both the computation efficiency and tracking accuracy. The extensive experiments in two real-world scenarios reveal that our approach can achieve up to 94% localization accuracy and an average 0.64Â m tracking error, outperforming other state-of-the-art radio-frequency identification based indoor localization systems.
ناشر
Database: Elsevier - ScienceDirect (ساینس دایرکت)
Journal: Journal of Network and Computer Applications - Volume 104, 15 February 2018, Pages 78-96
Journal: Journal of Network and Computer Applications - Volume 104, 15 February 2018, Pages 78-96
نویسندگان
Wenjie Ruan, Quan Z. Sheng, Lina Yao, Xue Li, Nickolas J.G. Falkner, Lei Yang,