کد مقاله | کد نشریه | سال انتشار | مقاله انگلیسی | نسخه تمام متن |
---|---|---|---|---|
6921221 | 864448 | 2015 | 11 صفحه PDF | دانلود رایگان |
عنوان انگلیسی مقاله ISI
Improving PLS-RFE based gene selection for microarray data classification
دانلود مقاله + سفارش ترجمه
دانلود مقاله ISI انگلیسی
رایگان برای ایرانیان
کلمات کلیدی
موضوعات مرتبط
مهندسی و علوم پایه
مهندسی کامپیوتر
نرم افزارهای علوم کامپیوتر
پیش نمایش صفحه اول مقاله
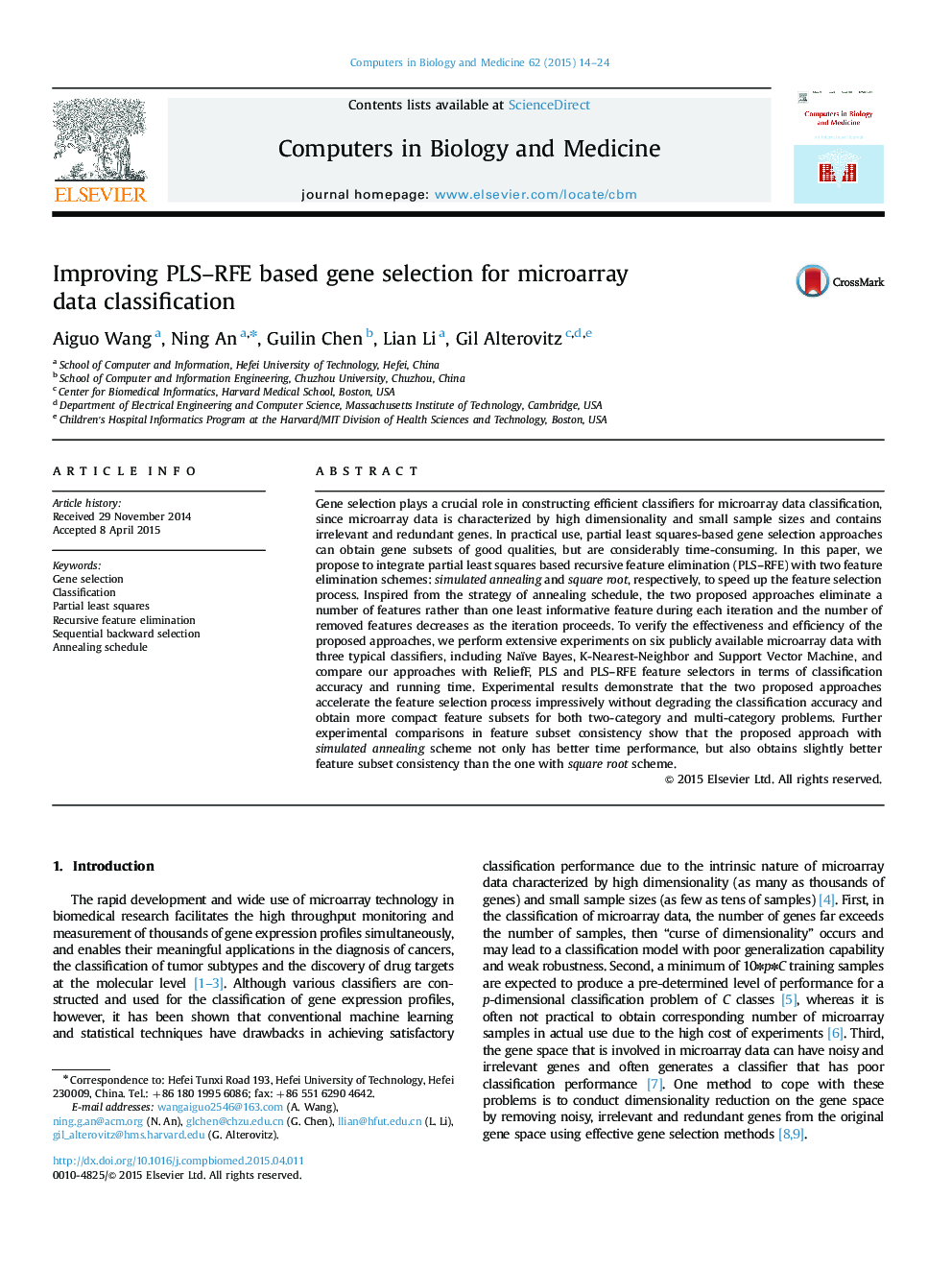
چکیده انگلیسی
Gene selection plays a crucial role in constructing efficient classifiers for microarray data classification, since microarray data is characterized by high dimensionality and small sample sizes and contains irrelevant and redundant genes. In practical use, partial least squares-based gene selection approaches can obtain gene subsets of good qualities, but are considerably time-consuming. In this paper, we propose to integrate partial least squares based recursive feature elimination (PLS-RFE) with two feature elimination schemes: simulated annealing and square root, respectively, to speed up the feature selection process. Inspired from the strategy of annealing schedule, the two proposed approaches eliminate a number of features rather than one least informative feature during each iteration and the number of removed features decreases as the iteration proceeds. To verify the effectiveness and efficiency of the proposed approaches, we perform extensive experiments on six publicly available microarray data with three typical classifiers, including Naïve Bayes, K-Nearest-Neighbor and Support Vector Machine, and compare our approaches with ReliefF, PLS and PLS-RFE feature selectors in terms of classification accuracy and running time. Experimental results demonstrate that the two proposed approaches accelerate the feature selection process impressively without degrading the classification accuracy and obtain more compact feature subsets for both two-category and multi-category problems. Further experimental comparisons in feature subset consistency show that the proposed approach with simulated annealing scheme not only has better time performance, but also obtains slightly better feature subset consistency than the one with square root scheme.
ناشر
Database: Elsevier - ScienceDirect (ساینس دایرکت)
Journal: Computers in Biology and Medicine - Volume 62, 1 July 2015, Pages 14-24
Journal: Computers in Biology and Medicine - Volume 62, 1 July 2015, Pages 14-24
نویسندگان
Aiguo Wang, Ning An, Guilin Chen, Lian Li, Gil Alterovitz,