کد مقاله | کد نشریه | سال انتشار | مقاله انگلیسی | نسخه تمام متن |
---|---|---|---|---|
6941442 | 1450112 | 2018 | 43 صفحه PDF | دانلود رایگان |
عنوان انگلیسی مقاله ISI
SGCRSR: Sequential gradient constrained regression for single image super-resolution
دانلود مقاله + سفارش ترجمه
دانلود مقاله ISI انگلیسی
رایگان برای ایرانیان
کلمات کلیدی
موضوعات مرتبط
مهندسی و علوم پایه
مهندسی کامپیوتر
چشم انداز کامپیوتر و تشخیص الگو
پیش نمایش صفحه اول مقاله
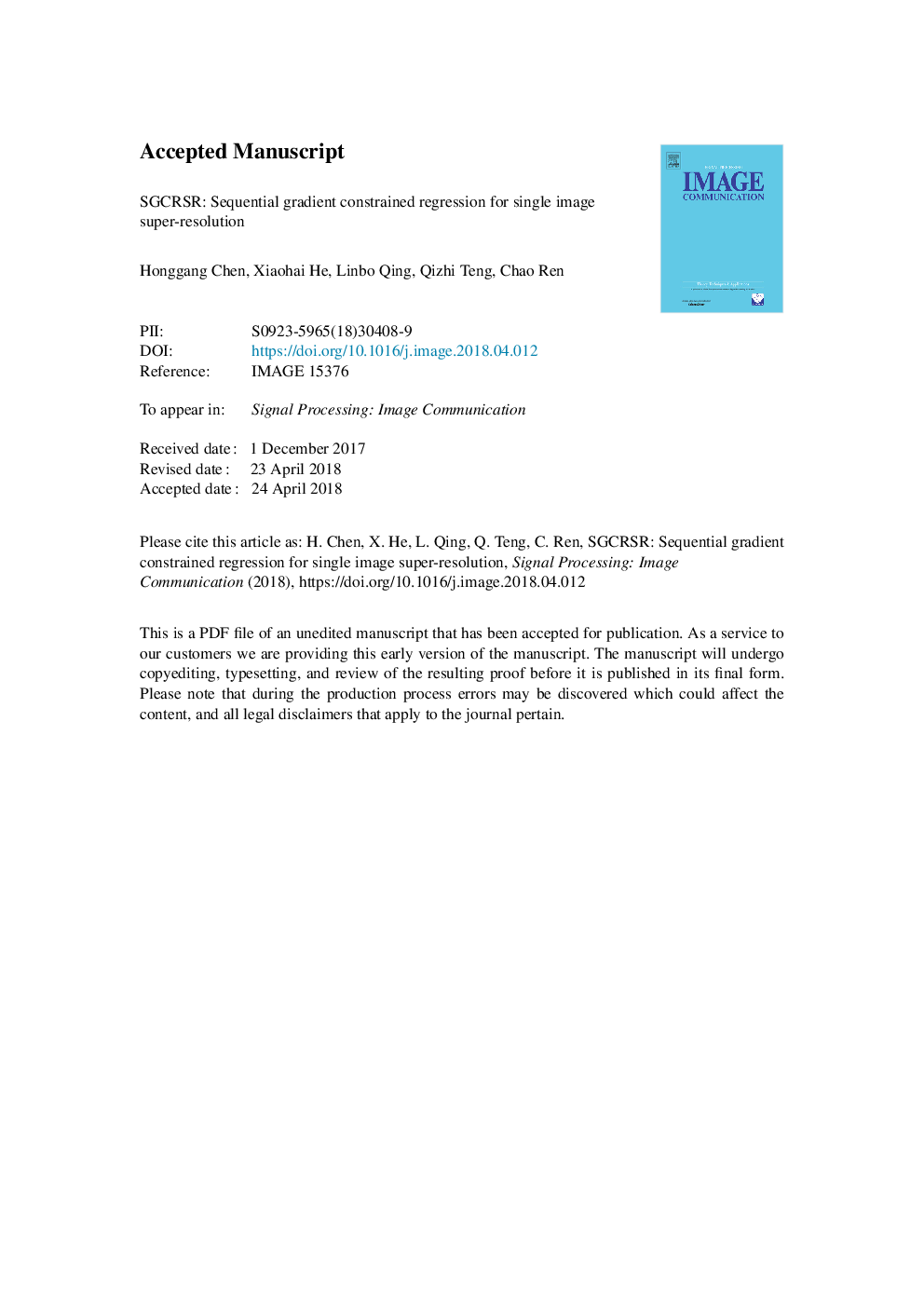
چکیده انگلیسی
Single image super-resolution (SISR), which aims to produce an image with higher resolution and better visual quality from the given single low-resolution (LR) image, has attracted extensive attention in recent years. In particular, the regression-based SISR approaches, which learn the mapping between LR and high-resolution (HR) patch pairs, are efficient and effective as a whole. However, the super-resolved images produced by this kind of method often suffer from visual artifacts as no extra constraints or priors are enforced. To alleviate these shortcomings, we propose a Sequential Gradient Constrained Regression-based single image Super-Resolution (SGCRSR) framework, which provides an effective way to combine the conventional learning-based and reconstruction-based approaches. Firstly, we improve the performance of the well-known super-resolution (SR) method A+ by addressing its deficiencies in both training and testing stages and propose the enhanced A+ (EA+). Then, the EA+ model is applied in dual intensity-gradient domain to construct the Gradient Constrained Regression (GCR)-based SR unit. Finally, a GCR-based sequential SR framework, namely the SGCRSR, is established to improve the quality of super-resolved images gradually. Extensive experiments show that the proposed SGCRSR achieves better performance than several state-of-the-art SR algorithms.
ناشر
Database: Elsevier - ScienceDirect (ساینس دایرکت)
Journal: Signal Processing: Image Communication - Volume 66, August 2018, Pages 1-18
Journal: Signal Processing: Image Communication - Volume 66, August 2018, Pages 1-18
نویسندگان
Honggang Chen, Xiaohai He, Linbo Qing, Qizhi Teng, Chao Ren,