کد مقاله | کد نشریه | سال انتشار | مقاله انگلیسی | نسخه تمام متن |
---|---|---|---|---|
6949458 | 1451272 | 2015 | 13 صفحه PDF | دانلود رایگان |
عنوان انگلیسی مقاله ISI
Feature space discriminant analysis for hyperspectral data feature reduction
دانلود مقاله + سفارش ترجمه
دانلود مقاله ISI انگلیسی
رایگان برای ایرانیان
کلمات کلیدی
موضوعات مرتبط
مهندسی و علوم پایه
مهندسی کامپیوتر
سیستم های اطلاعاتی
پیش نمایش صفحه اول مقاله
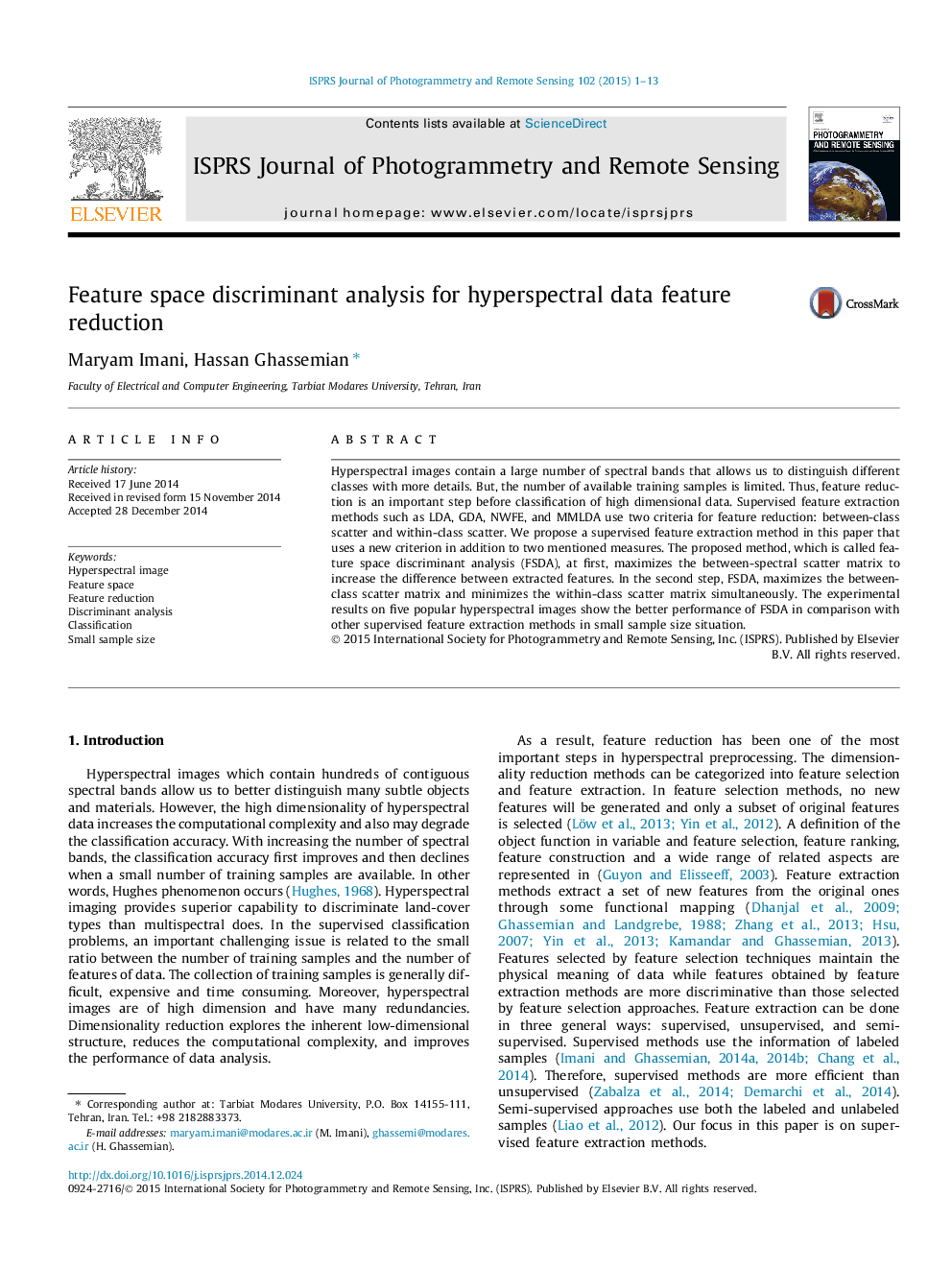
چکیده انگلیسی
Hyperspectral images contain a large number of spectral bands that allows us to distinguish different classes with more details. But, the number of available training samples is limited. Thus, feature reduction is an important step before classification of high dimensional data. Supervised feature extraction methods such as LDA, GDA, NWFE, and MMLDA use two criteria for feature reduction: between-class scatter and within-class scatter. We propose a supervised feature extraction method in this paper that uses a new criterion in addition to two mentioned measures. The proposed method, which is called feature space discriminant analysis (FSDA), at first, maximizes the between-spectral scatter matrix to increase the difference between extracted features. In the second step, FSDA, maximizes the between-class scatter matrix and minimizes the within-class scatter matrix simultaneously. The experimental results on five popular hyperspectral images show the better performance of FSDA in comparison with other supervised feature extraction methods in small sample size situation.
ناشر
Database: Elsevier - ScienceDirect (ساینس دایرکت)
Journal: ISPRS Journal of Photogrammetry and Remote Sensing - Volume 102, April 2015, Pages 1-13
Journal: ISPRS Journal of Photogrammetry and Remote Sensing - Volume 102, April 2015, Pages 1-13
نویسندگان
Maryam Imani, Hassan Ghassemian,