کد مقاله | کد نشریه | سال انتشار | مقاله انگلیسی | نسخه تمام متن |
---|---|---|---|---|
705243 | 891313 | 2011 | 7 صفحه PDF | دانلود رایگان |
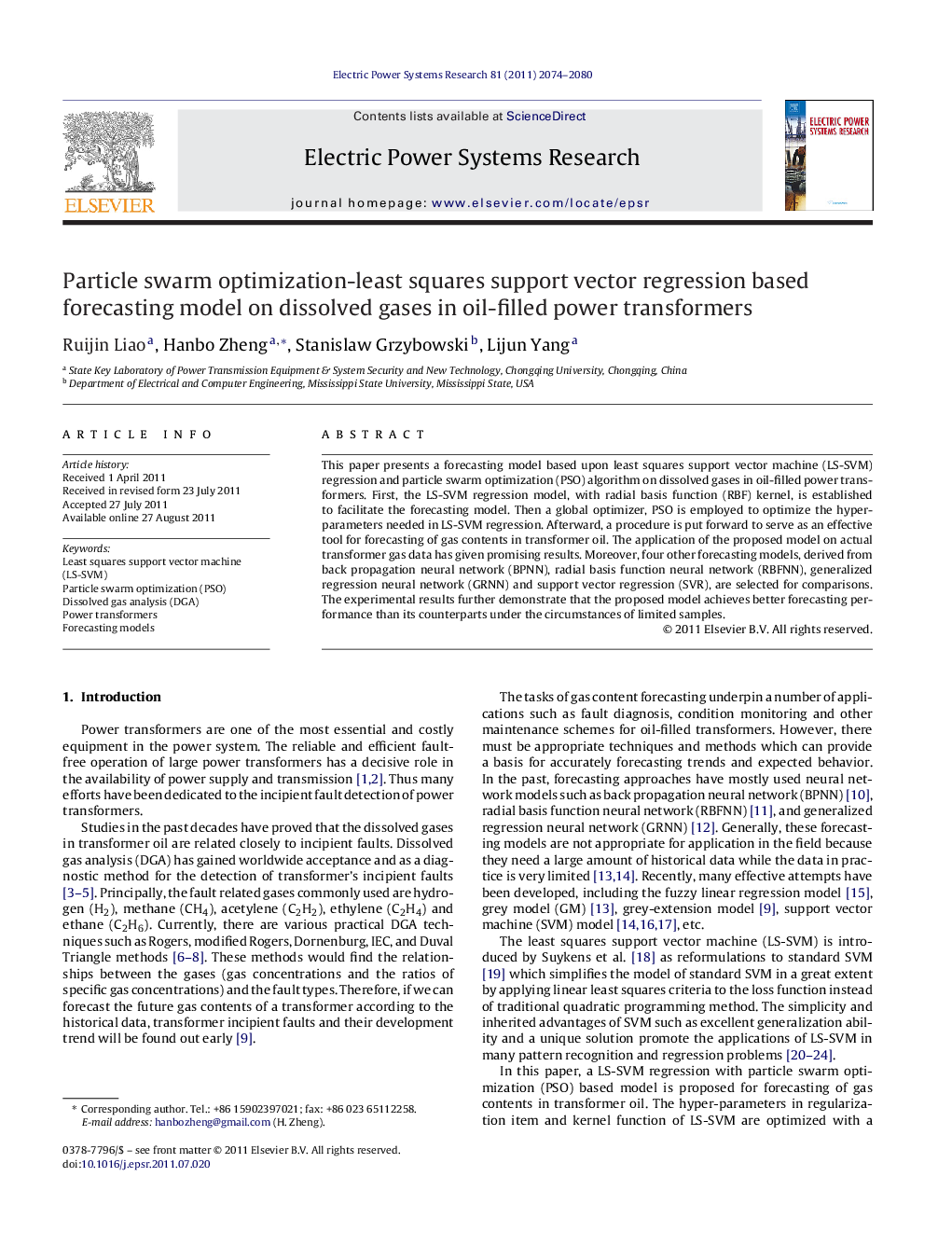
This paper presents a forecasting model based upon least squares support vector machine (LS-SVM) regression and particle swarm optimization (PSO) algorithm on dissolved gases in oil-filled power transformers. First, the LS-SVM regression model, with radial basis function (RBF) kernel, is established to facilitate the forecasting model. Then a global optimizer, PSO is employed to optimize the hyper-parameters needed in LS-SVM regression. Afterward, a procedure is put forward to serve as an effective tool for forecasting of gas contents in transformer oil. The application of the proposed model on actual transformer gas data has given promising results. Moreover, four other forecasting models, derived from back propagation neural network (BPNN), radial basis function neural network (RBFNN), generalized regression neural network (GRNN) and support vector regression (SVR), are selected for comparisons. The experimental results further demonstrate that the proposed model achieves better forecasting performance than its counterparts under the circumstances of limited samples.
► We present least squares support vector regression (LSSVR) based model to forecast the dissolved gas concentrations for oil-filled power transformers.
► We introduce the particle swarm optimization (PSO) to optimize the LSSVR model and put forward the forecasting procedure.
► We carry out the extended comparisons with other models derived from BPNN, RBFNN, GRNN, SVR.
Journal: Electric Power Systems Research - Volume 81, Issue 12, December 2011, Pages 2074–2080