کد مقاله | کد نشریه | سال انتشار | مقاله انگلیسی | نسخه تمام متن |
---|---|---|---|---|
7222974 | 1470555 | 2018 | 10 صفحه PDF | دانلود رایگان |
عنوان انگلیسی مقاله ISI
Hyperspectral image classification based on adaptive segmentation
دانلود مقاله + سفارش ترجمه
دانلود مقاله ISI انگلیسی
رایگان برای ایرانیان
کلمات کلیدی
موضوعات مرتبط
مهندسی و علوم پایه
سایر رشته های مهندسی
مهندسی (عمومی)
پیش نمایش صفحه اول مقاله
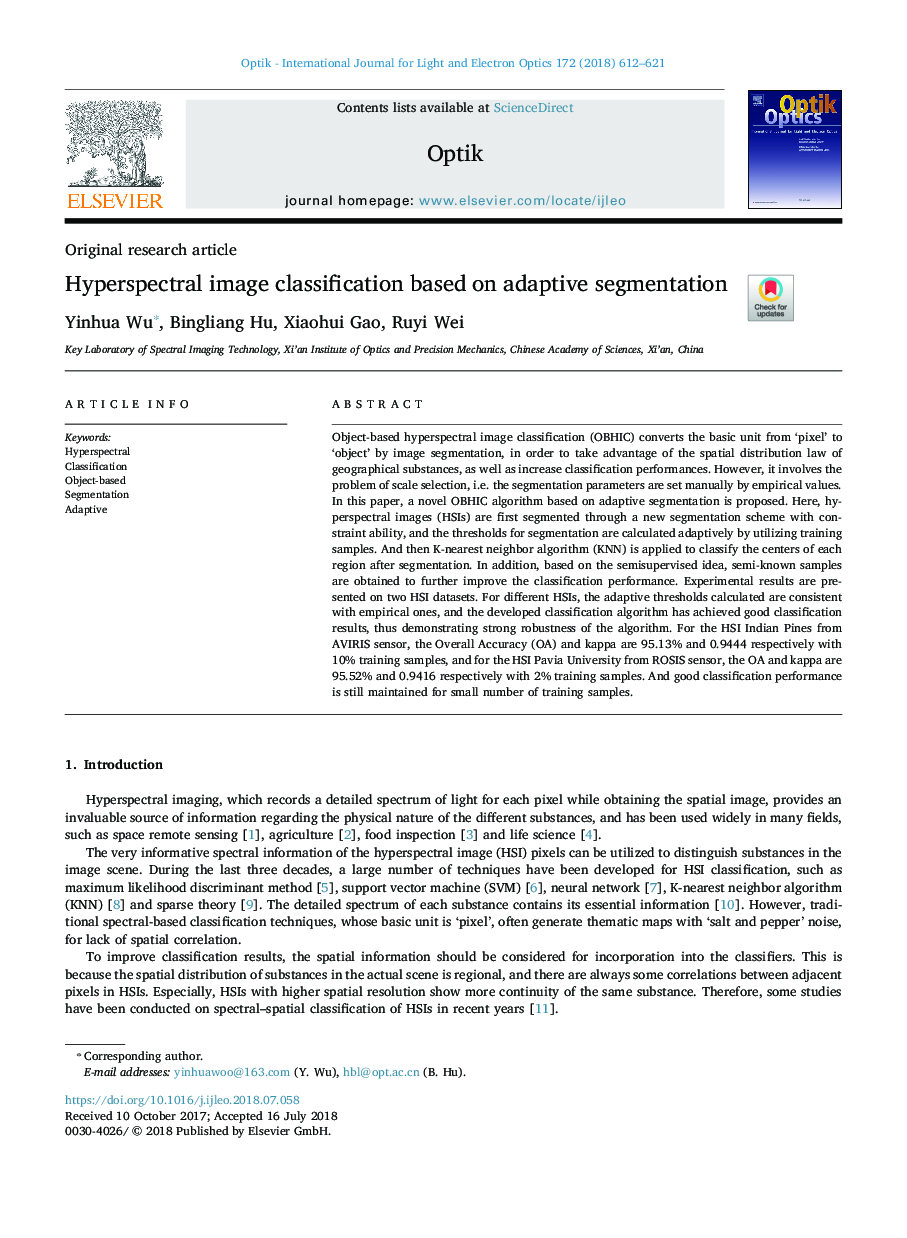
چکیده انگلیسی
Object-based hyperspectral image classification (OBHIC) converts the basic unit from 'pixel' to 'object' by image segmentation, in order to take advantage of the spatial distribution law of geographical substances, as well as increase classification performances. However, it involves the problem of scale selection, i.e. the segmentation parameters are set manually by empirical values. In this paper, a novel OBHIC algorithm based on adaptive segmentation is proposed. Here, hyperspectral images (HSIs) are first segmented through a new segmentation scheme with constraint ability, and the thresholds for segmentation are calculated adaptively by utilizing training samples. And then K-nearest neighbor algorithm (KNN) is applied to classify the centers of each region after segmentation. In addition, based on the semisupervised idea, semi-known samples are obtained to further improve the classification performance. Experimental results are presented on two HSI datasets. For different HSIs, the adaptive thresholds calculated are consistent with empirical ones, and the developed classification algorithm has achieved good classification results, thus demonstrating strong robustness of the algorithm. For the HSI Indian Pines from AVIRIS sensor, the Overall Accuracy (OA) and kappa are 95.13% and 0.9444 respectively with 10% training samples, and for the HSI Pavia University from ROSIS sensor, the OA and kappa are 95.52% and 0.9416 respectively with 2% training samples. And good classification performance is still maintained for small number of training samples.
ناشر
Database: Elsevier - ScienceDirect (ساینس دایرکت)
Journal: Optik - Volume 172, November 2018, Pages 612-621
Journal: Optik - Volume 172, November 2018, Pages 612-621
نویسندگان
Yinhua Wu, Bingliang Hu, Xiaohui Gao, Ruyi Wei,